Understanding User Search Intent
Understanding the user’s motivation behind a search query like “eat out restaurants near me” is crucial for optimizing online visibility and providing relevant results. This seemingly simple search hides a multitude of underlying needs and desires, each requiring a tailored approach to satisfy. Failing to understand these nuances can lead to missed opportunities and frustrated users. By dissecting the intent, we can craft targeted content and marketing strategies that resonate deeply with specific user segments.
The search “eat out restaurants near me” isn’t a monolithic query. It represents a spectrum of needs, from a quick and cheap lunch to a romantic anniversary dinner. Each user has a unique context, influencing their expectations and priorities. Ignoring this diversity will result in a generic, ineffective response. A sophisticated understanding of user intent translates directly into higher conversion rates and improved customer satisfaction.
User Motivations and Needs
Users searching for “eat out restaurants near me” have diverse motivations and needs. These can be broadly categorized based on factors like occasion, budget, and desired dining experience. Consider the differences between someone grabbing a quick bite before work and a couple celebrating a special occasion. These distinct scenarios demand different solutions.
User Persona: The Quick Lunch Grabber
This persona, let’s call her Sarah, is a busy professional. Sarah needs a fast, affordable, and convenient lunch option near her workplace. She values speed and efficiency above all else. She’s less concerned with ambiance and more focused on getting a satisfying meal quickly and returning to work. Sarah likely uses her phone during her lunch break and wants to see clear pricing and estimated wait times.
User Persona: The Romantic Dinner Couple
This persona, let’s call them Mark and Jessica, are looking for a special dining experience. They are seeking a romantic atmosphere, excellent food, and perhaps a curated wine list. Price is less of a concern than the overall experience. They’re likely planning ahead and researching options based on reviews, ambiance photos, and menu details. They may prioritize reservations and are looking for a memorable evening.
User Persona: The Family-Friendly Diner
This persona, let’s call them the Miller Family, is looking for a restaurant that caters to children. They prioritize a kid-friendly menu, a relaxed atmosphere, and possibly features like high chairs and a play area. Value for money is important, as is a menu with options for picky eaters. They might be searching on a weekend and are likely to check for family-friendly reviews and photos of the restaurant’s interior.
User Persona: The Specific Cuisine Seeker
This persona, let’s call him David, is looking for a specific type of cuisine, such as Thai food or Italian pizza. He already knows what he wants and is primarily searching for the nearest option offering that specific type of food. Reviews focusing on the authenticity and quality of the cuisine are important to him. He might also be looking at menus online to ensure the restaurant offers his desired dishes.
Local Restaurant Data Analysis
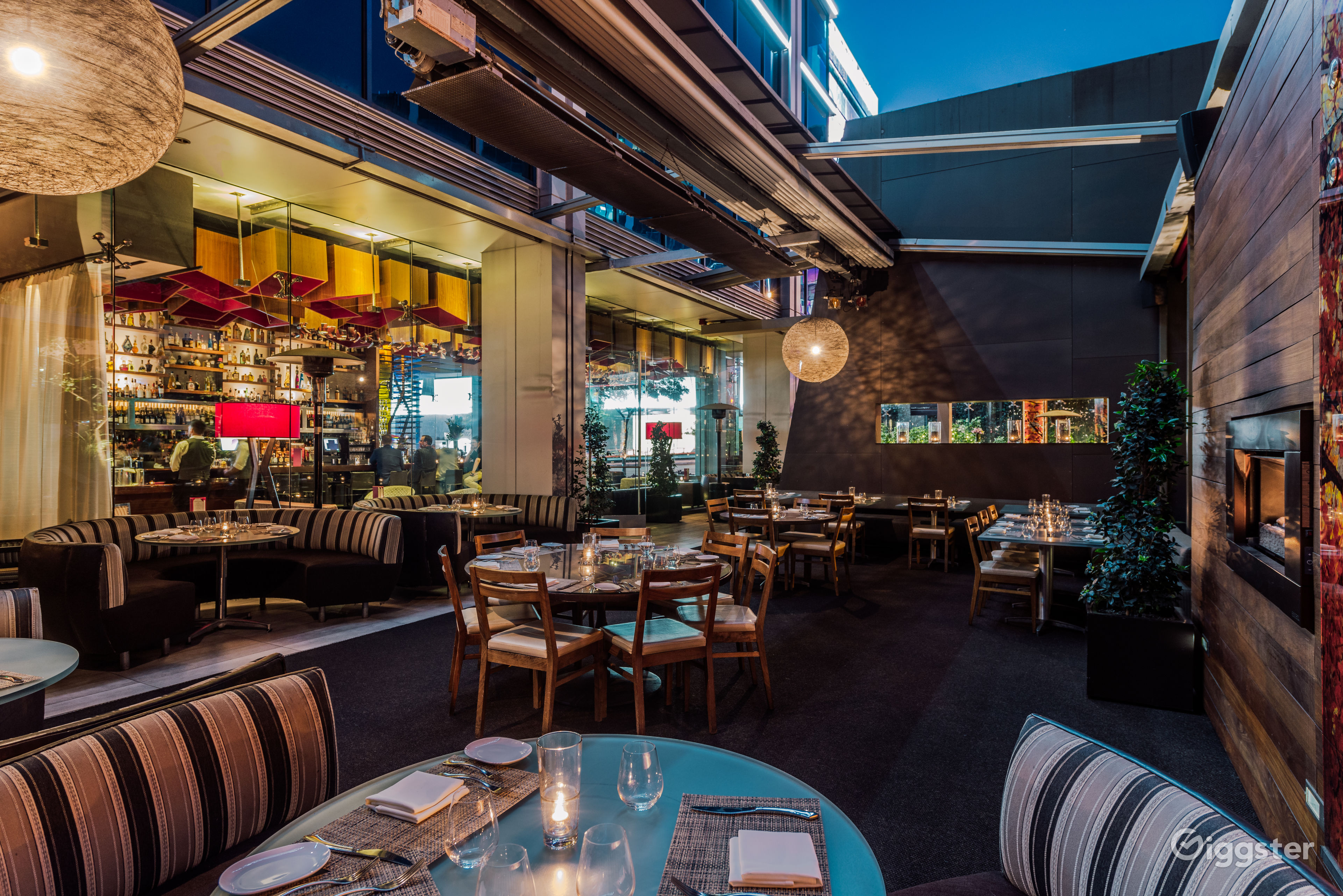
Understanding the nuances of local restaurant data is crucial for any business aiming to thrive in the competitive food industry, or even for a discerning consumer looking for the perfect dining experience. Effective analysis goes beyond simply finding restaurants; it involves understanding the factors influencing customer choices and leveraging various data sources to gain a comprehensive view of the local culinary landscape. This analysis allows for informed decisions, whether you’re a restaurant owner strategizing location or a customer seeking the best meal in your area.
Factors Influencing Restaurant Choice Based on Location
Proximity is obviously a key factor. People tend to choose restaurants within a reasonable driving distance or walking distance, especially for everyday meals. However, convenience also includes aspects like parking availability and ease of access. Beyond convenience, the local neighborhood itself plays a significant role. A restaurant located in a vibrant, upscale area might attract a different clientele than one situated in a quiet, residential neighborhood. The presence of complementary businesses, such as movie theaters or shopping centers, can also increase foot traffic and overall appeal. Finally, the perception of safety and cleanliness within the immediate vicinity significantly impacts a customer’s decision to dine at a particular establishment.
Comparison of Data Sources for Finding Local Restaurants
Several platforms provide data on local restaurants, each with its own strengths and weaknesses. Understanding these differences is essential for choosing the right tool for your specific needs. For example, Google Maps offers broad coverage, while Yelp focuses more on user reviews and ratings. Specialized restaurant websites may offer more curated lists or niche options. Analyzing these differences helps refine search strategies and provides a more complete picture of the local restaurant scene.
Name | Data Type | Strengths | Weaknesses |
---|---|---|---|
Google Maps | Restaurant listings, location data, user reviews, photos | Wide coverage, accurate location data, integrated with other Google services | Reviews can be less detailed than on specialized platforms, potential for biased reviews |
Yelp | Restaurant listings, user reviews, ratings, photos, price range | Extensive user reviews, detailed information, useful filtering options | Can be susceptible to fake reviews, coverage might be less comprehensive in certain areas |
Zomato (example of specialized site) | Restaurant listings, menus, photos, user reviews, reservations | Detailed menu information, often includes reservation options, strong focus on restaurant specifics | Coverage may be limited to specific geographic areas, less focus on overall location factors |
Restaurant Features and Attributes
Understanding the specific features and attributes of a restaurant is crucial for optimizing online search results and, more importantly, for satisfying the user’s needs. A potential diner’s decision-making process is heavily influenced by a multitude of factors, all of which should be carefully considered and presented clearly in your online restaurant profile. Failing to do so means losing potential customers to competitors who are more effectively communicating their value proposition.
The impact of accurate and comprehensive restaurant data on user experience is undeniable. Users are increasingly reliant on online platforms to discover and evaluate restaurants before making a visit. A poorly presented profile, lacking key details, can lead to lost opportunities and negative reviews. Conversely, a detailed and accurate profile enhances user confidence, encouraging bookings and positive word-of-mouth marketing.
Key Restaurant Attributes Influencing User Choice
The following attributes significantly impact a user’s decision to choose one restaurant over another. Presenting these clearly and accurately is essential for driving online bookings and foot traffic.
- Price Range: Clearly stating the average cost per person (e.g., $, $$, $$$) allows users to quickly filter options based on their budget. Ambiguity here can lead to disappointment and negative reviews.
- Cuisine Type: Precisely defining the cuisine (e.g., Italian, Mexican, Thai, Fusion) helps users find restaurants that cater to their specific preferences. Vague descriptions like “International Food” are less effective.
- Ambiance: Describing the atmosphere (e.g., romantic, casual, family-friendly, upscale) allows users to select a restaurant that matches the desired dining experience. Using evocative language is key here.
- Reviews and Ratings: Displaying aggregated reviews from reputable sources (e.g., Google, Yelp) builds trust and provides social proof. Highlighting both positive and negative reviews (with appropriate responses) demonstrates transparency.
- Hours of Operation: Clearly stating daily and weekly opening and closing times prevents wasted trips and frustrated customers. Consider highlighting any variations due to holidays or special events.
- Parking Availability: Indicating whether parking is available on-site, nearby, or requires valet service is especially important in urban areas. This information directly impacts the convenience factor for potential diners.
- Location and Accessibility: Providing a precise address, along with information about accessibility features (e.g., wheelchair ramps, accessible restrooms), ensures inclusivity and avoids potential problems for users with mobility limitations. Including a map link is also beneficial.
- Menu Options: While not directly an attribute, offering online access to a menu, or at least a sample menu, allows users to preview offerings and make informed decisions. This can significantly increase conversion rates.
- Dietary Options: Specifying available dietary options (e.g., vegetarian, vegan, gluten-free) caters to a wider range of customer needs and preferences, increasing the likelihood of attracting more diners.
- Reservations: Clearly stating whether reservations are accepted, and how to make them (e.g., online booking, phone call), streamlines the dining process for users. Consider integrating online booking directly into your online presence.
Visual Representation of Search Results
Optimizing the visual presentation of search results for “eat out restaurants near me” is crucial for user engagement and conversion. A well-designed results page should seamlessly integrate relevant information, making it effortless for users to find and select the perfect dining experience. This involves a strategic blend of visual elements, intuitive filtering options, and a clear visual hierarchy.
The visual design should prioritize speed and clarity. Users should instantly grasp the key information about each restaurant without needing to scroll extensively or strain their eyes.
Search Results Page Mock-up
Imagine a mobile-first design. The top portion of the screen displays a map centered on the user’s location, showing the relative positions of all listed restaurants. Each restaurant is represented by a pin, color-coded perhaps by cuisine type (e.g., Italian restaurants are green, Mexican are red, etc.). Clicking a pin expands a small info card displaying the restaurant’s name, rating, and a small preview image. This map is interactive, allowing users to zoom and pan freely.
Below the map, the main list of restaurants appears. Each restaurant entry includes a prominent image (perhaps a high-quality hero shot of a signature dish or the restaurant’s ambiance), its name in a bold, easily readable font, its overall rating (e.g., 4.5 stars out of 5), the type of cuisine, a price range indicator (e.g., $, $$, $$$), and the distance from the user’s location. The layout should be clean, with ample white space to prevent visual clutter. For example, Yelp uses a similar approach, presenting images, ratings, and key details concisely.
Filtering and Sorting Results
To refine search results, a robust filtering system is essential. This could be implemented as a collapsible panel, accessible via a clearly labeled button (e.g., “Filter Results”). Within this panel, users could select options for:
- Cuisine Type: A dropdown menu or a series of checkboxes listing various cuisines (e.g., American, Italian, Mexican, Chinese, etc.).
- Price Range: A slider or a series of radio buttons representing different price ranges ($, $$, $$$, $$$$).
- Rating: A slider or a series of checkboxes to filter by minimum star rating.
- Distance: A slider to specify the maximum distance from the user’s location.
- Dietary Restrictions: Checkboxes for common dietary restrictions like vegetarian, vegan, gluten-free, etc.
Sorting options should also be readily available, perhaps as a dropdown menu labeled “Sort By.” Options could include:
- Rating: Highest rated first.
- Distance: Closest first.
- Price: Lowest price first.
- Popularity: Most reviewed first.
Visual Hierarchy and User Experience
The visual hierarchy dictates the order in which users perceive information. To enhance user experience, the most important elements should be visually prominent. In this context, the restaurant images, names, and ratings should be the most eye-catching elements. Secondary information (cuisine, price, distance) should be clearly visible but less dominant. Using appropriate font sizes, weights, and colors will be key. For instance, the restaurant name should be larger and bolder than the cuisine type. Consistent use of spacing and visual cues will create a clear and intuitive flow. A clear visual hierarchy minimizes cognitive load, making the search experience quick and efficient. Consider the visual design of Google Maps; its simplicity and clear hierarchy are exemplary.
User Reviews and Ratings

User reviews and ratings are undeniably the most influential factor shaping a diner’s decision when choosing a restaurant. In today’s hyper-connected world, potential customers rely heavily on the collective wisdom of their peers before committing to a dining experience. This reliance significantly impacts restaurant success, making effective review management a critical component of any successful restaurant marketing strategy. Understanding how these reviews influence choices and leveraging data analysis to improve the business are key to thriving in the competitive restaurant landscape.
The impact of online reviews extends beyond simple star ratings. A comprehensive understanding requires analyzing the sentiment expressed within the reviews themselves. This is where review sentiment analysis comes into play. Sentiment analysis goes beyond simply counting positive and negative reviews; it delves into the nuances of language to understand the underlying emotion and opinion expressed by each reviewer. This granular level of analysis allows restaurants to identify specific areas for improvement and to proactively address concerns raised by their customers. Ignoring this crucial data point is a significant missed opportunity for growth and improvement.
Review Sentiment Analysis: Uncovering Valuable Insights, Eat out restaurants near me
Review sentiment analysis uses natural language processing (NLP) and machine learning algorithms to categorize reviews as positive, negative, or neutral. However, the true power lies in identifying the specific aspects of the restaurant that are driving positive or negative sentiment. For example, a restaurant might receive many positive reviews praising its excellent service, but also several negative reviews criticizing the slow kitchen turnaround time. This detailed analysis enables targeted improvements. By pinpointing specific pain points, restaurants can allocate resources effectively to enhance their overall customer experience. For example, a restaurant might invest in additional kitchen staff to address slow service, or implement a new reservation system to better manage customer flow.
Examples of Positive and Negative Review Phrases
Understanding the language used in reviews is crucial. Positive reviews often include phrases such as “delicious food,” “excellent service,” “friendly staff,” “great atmosphere,” “highly recommend,” and “will definitely return.” These phrases signal a high level of customer satisfaction and contribute to a positive online reputation. Conversely, negative reviews may contain phrases like “poor service,” “overpriced,” “long wait times,” “unclean restaurant,” “disappointing food,” and “would not recommend.” These phrases highlight areas requiring immediate attention and can severely damage a restaurant’s reputation if left unaddressed. A single negative review mentioning food poisoning, for example, can have a devastating impact on business, highlighting the importance of proactive review monitoring and response.
Restaurant Recommendations and Personalization
Personalizing the restaurant recommendation experience is crucial for driving user engagement and loyalty in today’s competitive online landscape. Users are overwhelmed with choices; providing them with tailored suggestions significantly improves their satisfaction and increases the likelihood of them choosing your platform for their dining needs. This translates directly into increased bookings and revenue for the restaurants listed. By understanding user preferences and past behavior, we can move beyond generic search results and deliver truly valuable recommendations.
Personalized restaurant recommendations enhance user experience by saving time and reducing decision fatigue. Instead of sifting through countless options, users are presented with a curated selection of restaurants perfectly matched to their individual tastes and needs. This leads to higher satisfaction rates, increased conversion rates, and ultimately, a more loyal user base. Imagine a user who consistently orders Italian food and prefers casual settings – a system that only shows them Italian trattorias and avoids upscale establishments significantly improves their experience.
Methods for Creating Personalized Restaurant Recommendations
Creating effective personalized recommendations requires a multi-pronged approach leveraging various data points and algorithms. The following table Artikels several key methods, their descriptions, and their respective advantages and disadvantages.
Method | Description | Advantages | Disadvantages |
---|---|---|---|
Collaborative Filtering | This method analyzes user preferences based on the preferences of similar users. If User A and User B both frequently visit Italian restaurants, the system recommends similar restaurants to both. | Discover hidden gems and less popular restaurants based on similar user tastes; effective for niche preferences. | Requires a large user base for accurate recommendations; can struggle with new users or restaurants with limited data. |
Content-Based Filtering | This method recommends restaurants based on a user’s past behavior. If a user has consistently rated highly-rated seafood restaurants, the system will prioritize similar restaurants. | Simple to implement; works well even with a small user base; good for highlighting specific features users enjoy. | Can lead to recommendation bubbles; users may miss out on diverse options; doesn’t account for changing preferences. |
Hybrid Approach | Combines collaborative and content-based filtering to leverage the strengths of both methods. This often provides the most accurate and diverse recommendations. | Offers a more balanced and comprehensive recommendation system; mitigates the weaknesses of individual methods. | More complex to implement; requires more computational resources. |
Knowledge-Based Systems | Uses explicit user preferences (e.g., dietary restrictions, price range) and restaurant attributes (cuisine, ambiance) to generate recommendations. | Directly addresses user needs; highly transparent and controllable; allows for precise filtering. | Requires extensive data on restaurant attributes; relies on users accurately inputting their preferences. |
Handling Ambiguous Search Queries: Eat Out Restaurants Near Me
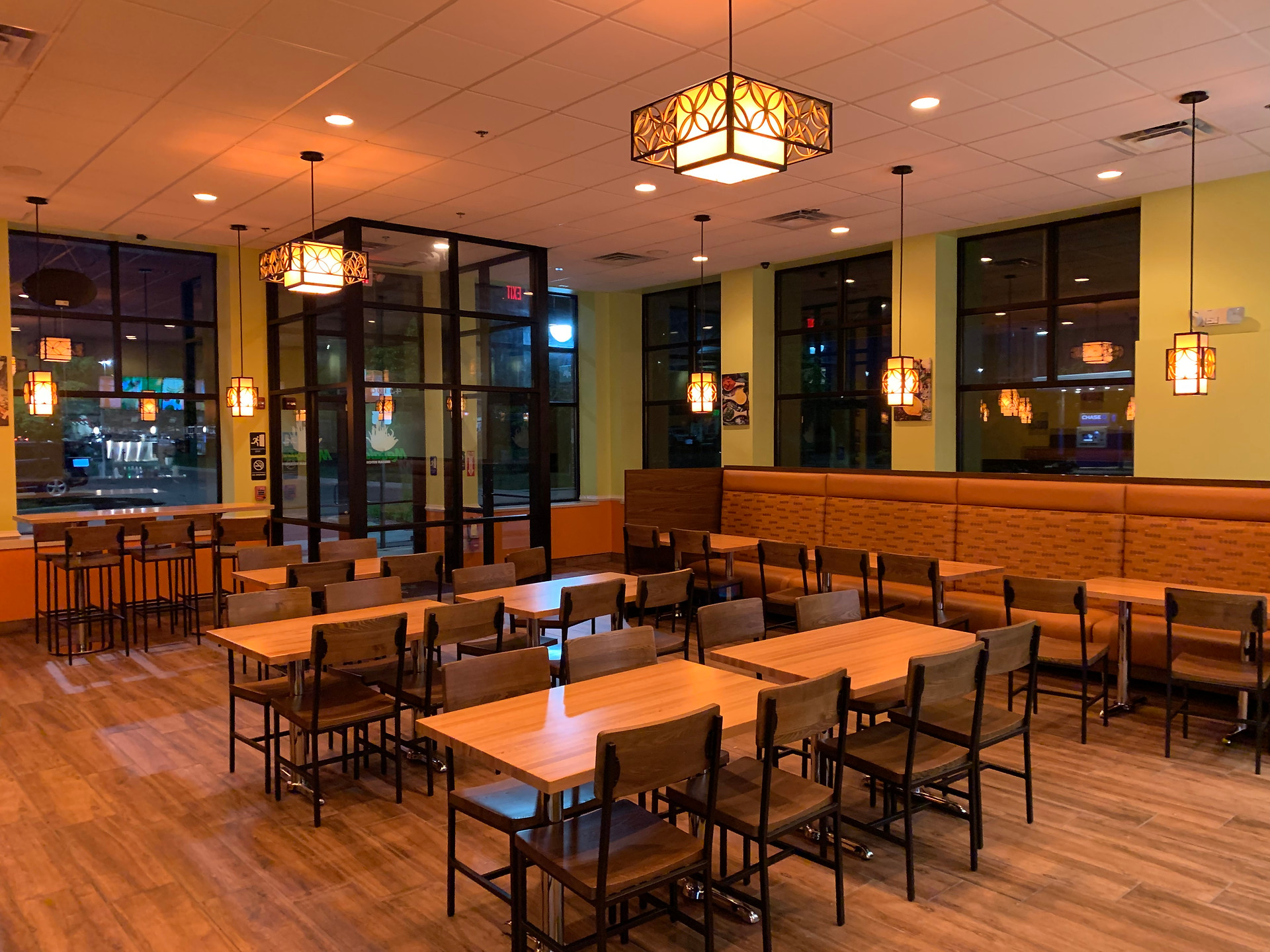
The seemingly simple query “eat out restaurants near me” hides a multitude of potential ambiguities. Successfully delivering relevant results hinges on understanding and resolving these uncertainties, a critical aspect of building a truly effective restaurant discovery system. Failing to address these ambiguities leads to a frustrating user experience and lost opportunities for both users and businesses.
The primary ambiguities stem from the undefined nature of “near me” and the lack of specified cuisine preferences. “Near me” requires geolocation data, which may not always be readily available or accurate. Similarly, the absence of a cuisine type leaves the system to interpret a vast range of possibilities, potentially overwhelming the user with irrelevant suggestions.
Location Clarification Strategies
To effectively address the “near me” ambiguity, we must leverage available contextual information. If the user is logged in, their previously saved location or IP address can be used to provide a default location. However, this should always be presented as a suggestion, allowing users to override the default with a specific address or area. Implementing a map interface where users can manually pinpoint their desired location offers a superior level of control and precision. Further refinement can be achieved by incorporating radius filters, allowing users to specify a search distance (e.g., “within 5 miles”).
Cuisine Type Disambiguation
Handling undefined cuisine types requires a multi-pronged approach. One strategy is to present a curated selection of popular cuisines in the area. This could be based on local trends or historical search data, providing a starting point for users to refine their search. Alternatively, employing natural language processing (NLP) techniques to identify potential cuisine preferences within the user’s search history or broader browsing behavior can personalize the experience. For example, if a user frequently searches for “Italian food recipes,” the system could intelligently suggest Italian restaurants even without explicit mention in the current query.
Contextual Information Utilization
Consider a user searching “eat out restaurants near me” while browsing a travel website dedicated to a specific city. In this context, the “near me” ambiguity can be resolved by assuming the user’s location is within that city. Similarly, if the user is on a page featuring articles about Mexican cuisine, the system could infer a preference for Mexican restaurants, even if not explicitly stated. These contextual cues dramatically improve the accuracy and relevance of search results, providing a more seamless and intuitive user experience. Leveraging user browsing history and integrating with other services can significantly enhance this contextual understanding.