Understanding User Intent Behind “Lunch Near Me”
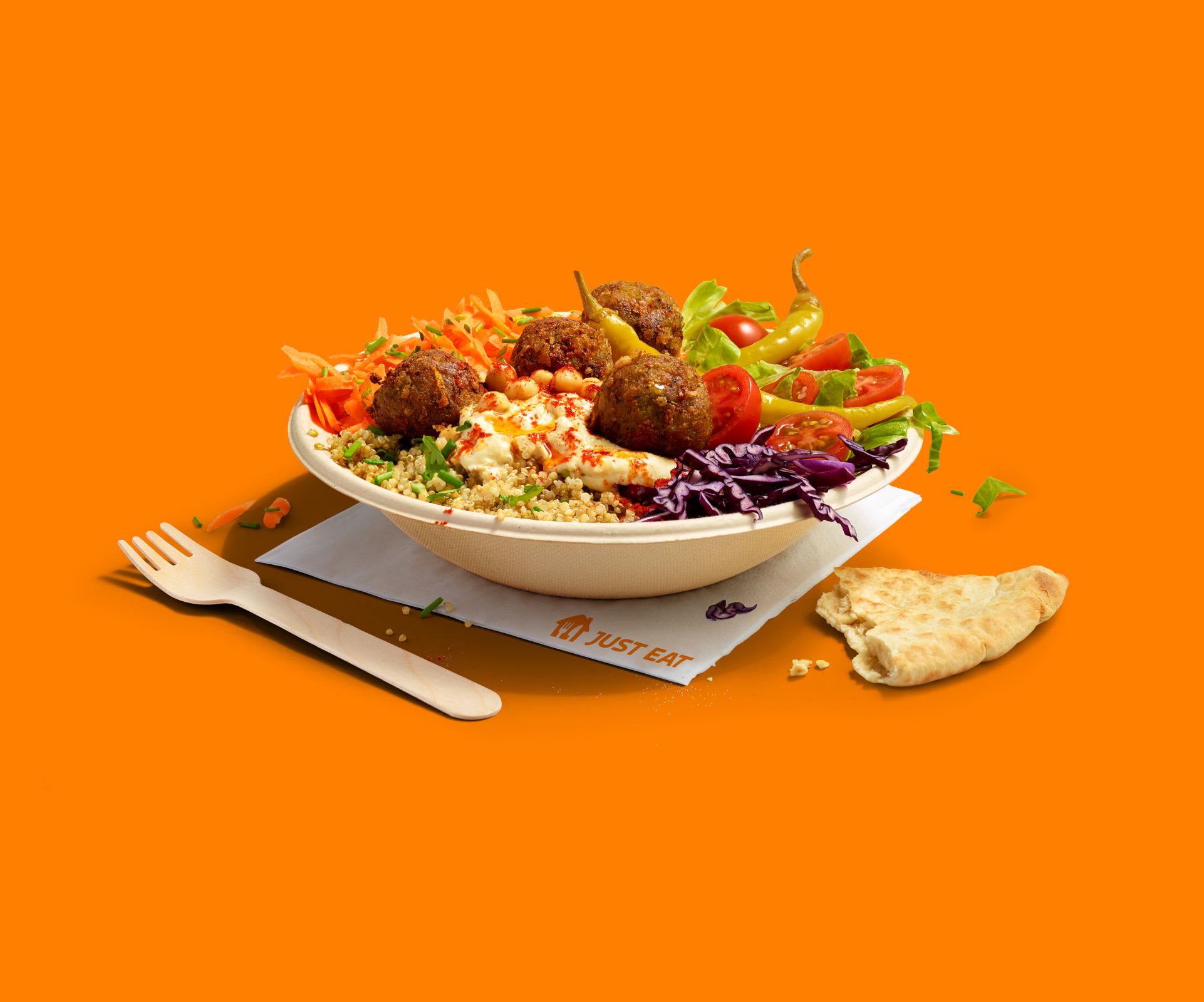
The seemingly simple search query “lunch near me” reveals a complex tapestry of user needs and desires. Understanding these underlying intentions is crucial for businesses looking to optimize their online presence and attract hungry customers. This goes beyond simply providing a list of restaurants; it’s about anticipating the specific context of each search.
Lunch near me – The user’s intent isn’t always explicitly stated. Sometimes, it’s a quick bite, other times, it’s a leisurely affair. This nuance is what separates effective search engine optimization () from generic stuffing.
User Needs and Lunch Options
Users searching for “lunch near me” exhibit a wide range of needs, impacting their choice of establishment. These needs often intertwine, creating a multifaceted decision-making process.
For example, a busy professional might prioritize speed and convenience, opting for a quick-service restaurant or a place offering takeout. A health-conscious individual might seek out a salad bar or a restaurant with nutritious options. Conversely, a celebratory lunch might involve a more elaborate fine-dining experience.
Factors Influencing Lunch Location Choice
Several factors influence a user’s decision when choosing a lunch spot. These factors often interact, leading to a personalized selection process that reflects individual preferences and immediate circumstances.
Proximity plays a significant role; a user is unlikely to travel across town for a simple sandwich. Budget constraints also heavily influence the choice, with price ranges varying widely depending on the user’s financial situation and the type of lunch they are looking for. Cuisine preferences and online reviews also hold considerable weight, with positive reviews often swaying decisions in favor of a particular restaurant.
Factors Influencing Lunch Location Selection
Factor | Description | Importance | Example |
---|---|---|---|
Distance | Physical proximity to the user’s current location. | High, especially for quick lunches. | A user won’t travel across town for a fast-food lunch. |
Price Range | Cost of the meal, reflecting the user’s budget. | High; budget significantly impacts restaurant choice. | A student might choose a cheaper option compared to a business executive. |
Cuisine Type | Specific type of food the user desires (e.g., Italian, Mexican, etc.). | Medium to High; depends on the user’s preferences. | Someone craving pizza will search for pizza restaurants. |
Reviews | User ratings and comments about the restaurant’s food and service. | High; positive reviews often influence decisions. | A restaurant with consistently high ratings is more likely to be chosen. |
Location-Based Services and “Lunch Near Me”
The seemingly simple search query, “lunch near me,” unleashes a complex interplay of technology, specifically location-based services (LBS), to deliver relevant results. Understanding how this works is crucial for anyone building location-aware applications or analyzing user behavior online. The power lies in the seamless integration of GPS technology, mapping services, and sophisticated algorithms to translate a user’s implicit request for nearby lunch options into a curated list of viable choices.
Location data is the backbone of this process. GPS, or Global Positioning System, utilizes a network of satellites to pinpoint a device’s location with remarkable accuracy. This location data is then transmitted to mapping services like Google Maps or Apple Maps, which possess extensive databases of businesses, including restaurants. These databases are constantly updated, reflecting changes in restaurant locations, operating hours, and even menu offerings.
GPS and Location Services in “Lunch Near Me” Searches
GPS technology works by receiving signals from multiple satellites orbiting Earth. By measuring the time it takes for these signals to reach a device, the device can calculate its precise location using triangulation. This location information, usually expressed as latitude and longitude coordinates, is then shared with the user’s device’s operating system and apps that have requested access to location data. Apps like Google Maps or Yelp use this information to filter restaurant listings, presenting only those within a specific radius of the user’s location. Accuracy can be affected by factors like atmospheric conditions and the density of buildings, but generally provides results within a margin of error small enough to be practically useful.
The Role of Mapping Services
Mapping services are indispensable in translating raw location data into actionable information. They act as the intermediary between the user’s location and the database of nearby restaurants. These services don’t simply display points on a map; they employ sophisticated algorithms to organize and prioritize results based on factors like distance, user reviews, and popularity. For instance, a search for “lunch near me” might prioritize restaurants with high ratings, positive user reviews, and a close proximity to the user’s location, presenting a ranked list of options tailored to the user’s preference and physical location. The visual aspect of the map is also key, allowing users to see the restaurants’ relative positions and potentially plan their route.
Refining Search Results with Location Data, Lunch near me
Location data is used in numerous ways to refine search results beyond simple proximity. For example:
- Radius Filtering: Users can often specify a search radius (e.g., 1 mile, 5 miles) to further narrow the results.
- Cuisine Filtering: Location data can be combined with user preferences (e.g., “Italian restaurants near me”) to refine results based on restaurant type.
- Operating Hours: Restaurants with current operating hours are prioritized over those that are closed, ensuring relevant results.
- Traffic Considerations: Some services integrate real-time traffic data to estimate travel times and adjust result rankings accordingly.
- Personalization: Based on past search history and preferences, the algorithm may prioritize certain restaurants over others.
Flowchart Illustrating the Process
Imagine a flowchart: The process begins with the user initiating the search (“lunch near me”). This triggers the device to access its GPS coordinates. These coordinates are then sent to a mapping service (e.g., Google Maps). The mapping service queries its database of restaurants, filtering based on proximity, user preferences (if specified), and other relevant factors. The filtered results are then ranked and presented to the user in a visually appealing format, often including maps, photos, reviews, and operating hours. The user then selects a restaurant and potentially navigates there using the mapping service’s navigation feature. This entire process occurs almost instantaneously, showcasing the efficiency and sophistication of modern location-based services.
Data Sources for Lunch Recommendations
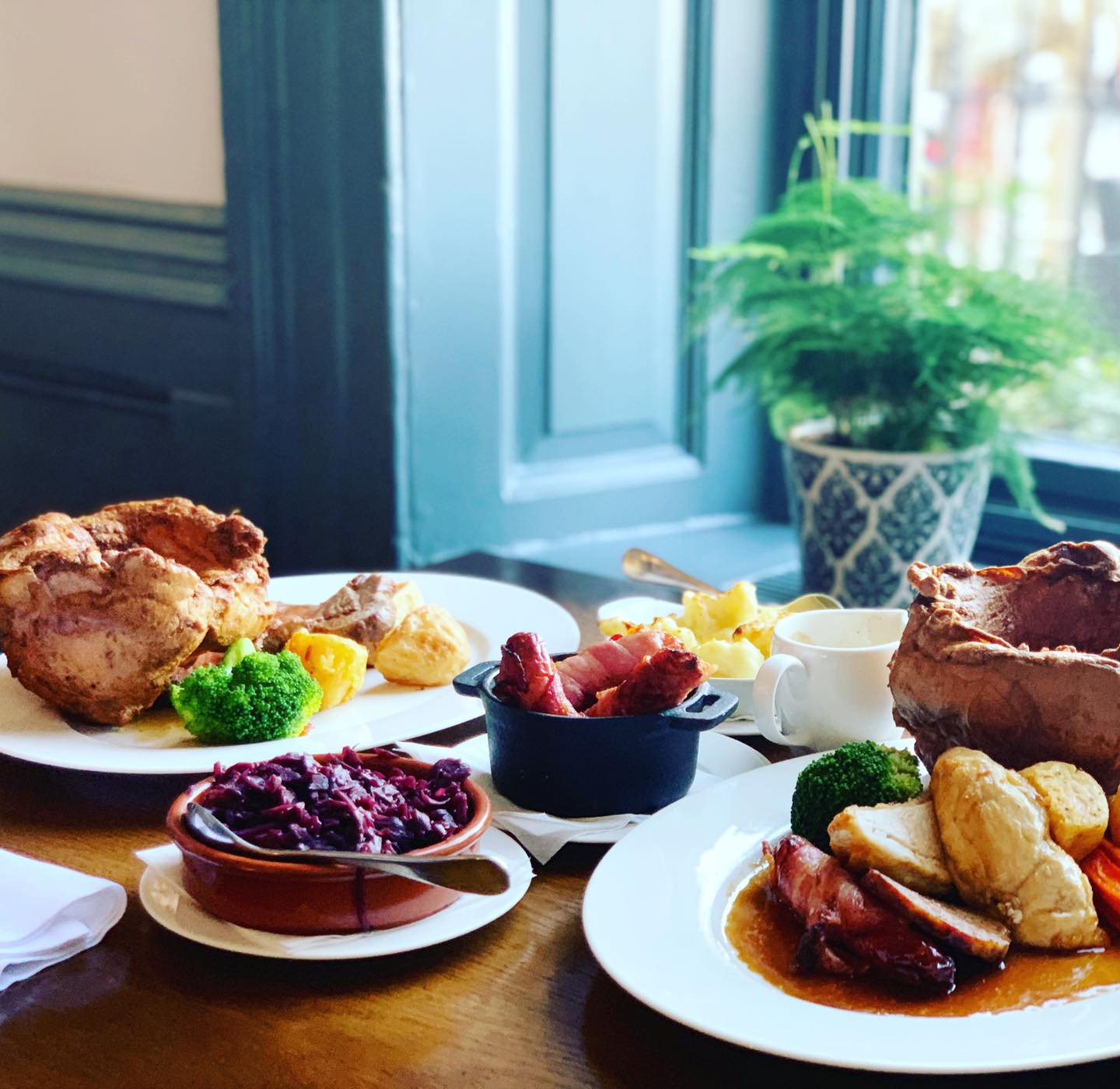
The accuracy and comprehensiveness of lunch recommendations hinge entirely on the quality of the data used to generate them. Location-based services rely on a complex interplay of information sources, each with its own strengths and weaknesses. Understanding these sources is crucial for building a truly effective and reliable system. Ignoring this critical element can lead to inaccurate suggestions, frustrated users, and ultimately, a failed product.
The power of any location-based service, particularly one focused on finding lunch options, lies in its ability to synthesize information from diverse sources into a cohesive and user-friendly experience. This involves more than simply pulling data; it requires careful evaluation, validation, and intelligent integration to ensure the results are relevant, timely, and trustworthy.
Restaurant Databases
Restaurant databases are a cornerstone of location-based lunch recommendation systems. These databases typically contain structured information about restaurants, including their name, address, phone number, cuisine type, price range, operating hours, and sometimes even menus. However, the reliability of this data varies significantly depending on the database provider and how frequently the information is updated. Larger, more established providers generally offer more accurate and comprehensive data, but even these can suffer from inaccuracies due to the constant flux of the restaurant industry—new openings, closures, menu changes, and address updates. Smaller, less well-maintained databases might contain outdated or incomplete information, leading to unreliable recommendations. For example, a database might list a restaurant as open when it has actually closed, or fail to include a newly opened, highly-rated eatery.
User Reviews and Ratings
User-generated content, such as reviews and ratings on platforms like Yelp, Google Maps, or TripAdvisor, provides invaluable real-time feedback on restaurants. This data offers insights into customer experiences, including food quality, service, atmosphere, and value. The sheer volume of reviews can help identify trends and patterns, providing a more nuanced picture than simply relying on static restaurant data. However, user reviews are not without their limitations. They can be subjective, biased, and sometimes even fraudulent. Furthermore, the sheer volume of data can be overwhelming and requires sophisticated filtering and aggregation techniques to extract meaningful insights. For instance, a few negative reviews amidst a sea of positive ones might still significantly impact a restaurant’s overall rating, and identifying those genuinely problematic reviews amidst potentially biased or malicious ones is a constant challenge.
Social Media Data
Social media platforms like Instagram, Facebook, and Twitter offer another rich source of information about restaurants. User-generated photos, check-ins, and mentions can provide valuable insights into a restaurant’s popularity, ambiance, and menu offerings. This data is often more immediate and reflects current trends and customer preferences. However, social media data is often unstructured and requires sophisticated natural language processing (NLP) techniques to extract meaningful information. Furthermore, the data can be noisy and biased, and may not always reflect the true quality of a restaurant. A highly-Instagrammable restaurant might be popular for its aesthetics rather than its food, leading to misleading recommendations. Also, the organic reach of posts and the presence of paid advertising significantly influences what information users see, creating a skewed perspective.
Comparison of Data Sources
The following table summarizes the strengths and weaknesses of the three data sources discussed above:
Data Source | Strengths | Weaknesses |
---|---|---|
Restaurant Databases | Structured data, comprehensive information (when accurate), consistent format | Can be outdated, prone to inaccuracies, may lack real-time feedback |
User Reviews & Ratings | Real-time feedback, diverse perspectives, reflects customer experiences | Subjective, prone to bias and fraud, requires sophisticated filtering |
Social Media Data | Immediate insights, reflects current trends, rich multimedia content | Unstructured data, noisy, biased, requires NLP for analysis |
Visual Representation of Lunch Options: Lunch Near Me
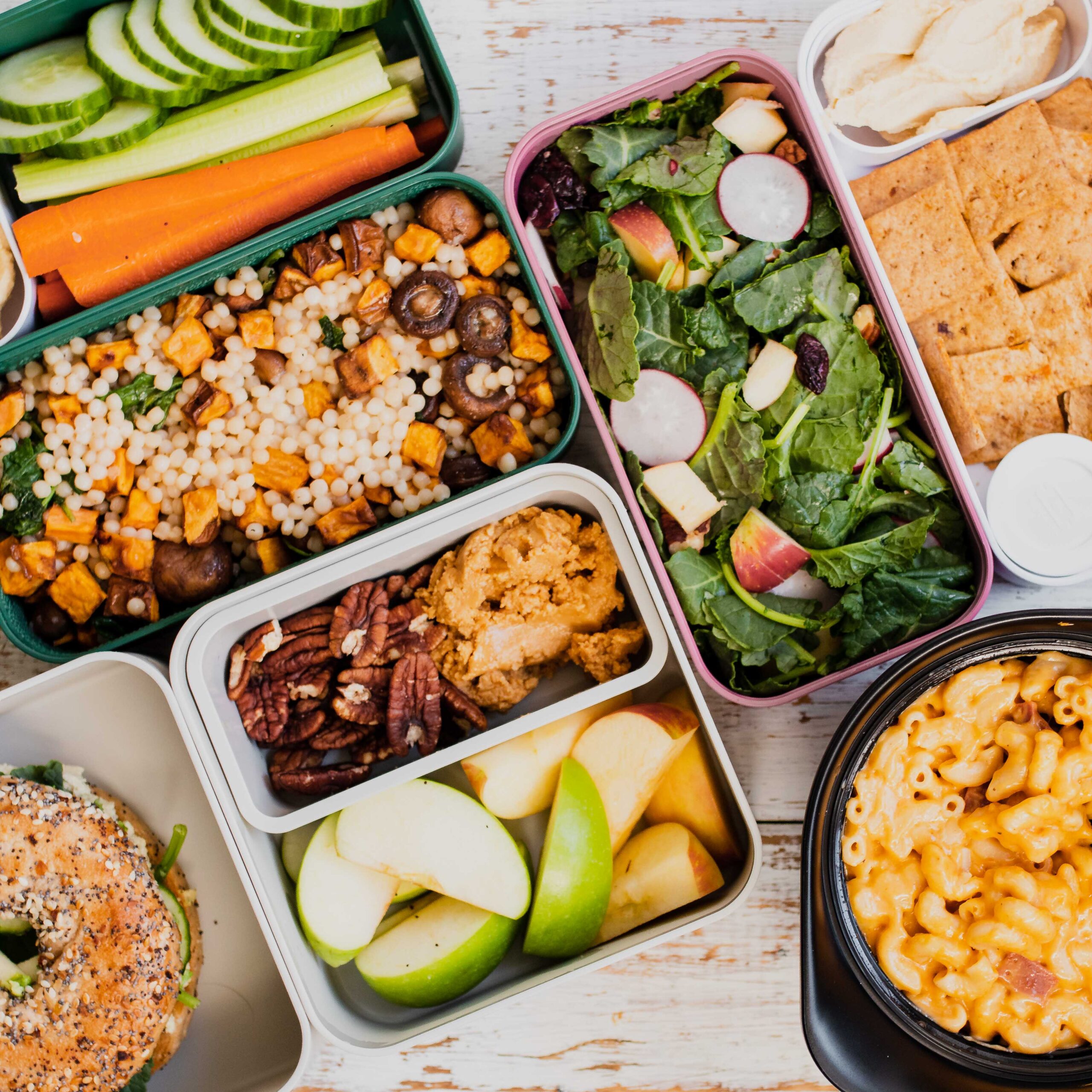
In today’s hyper-visual world, the effectiveness of a “lunch near me” search hinges critically on compelling visual representation. A simple list of restaurant names and addresses is simply insufficient; users crave a quick, immersive experience that allows them to instantly assess whether a restaurant aligns with their preferences. High-quality visuals are the key to unlocking engagement and driving conversions.
High-quality images and well-structured information dramatically improve user experience. A picture is worth a thousand words, especially when choosing a place to eat. Seeing mouthwatering photos of dishes, the inviting ambiance of a restaurant, or even a quick glance at a menu can sway a decision more effectively than any amount of text. This visual immediacy reduces decision fatigue and allows users to quickly filter options based on their visual preferences.
Effective Visual Representations of Restaurant Information
Effective visual representations go beyond simple snapshots. They strategically combine different visual elements to provide a holistic view of the restaurant. High-resolution photos of signature dishes, showcasing plating and ingredients, are crucial. Images of the restaurant’s interior, capturing the atmosphere (casual, upscale, family-friendly, etc.), are equally important. Menu snippets, showing price points and popular items, can provide valuable context. Consider a carousel of images showing various aspects of the restaurant experience—the food, the ambiance, and maybe even happy customers.
Structuring Restaurant Information with HTML Blockquotes
Utilizing HTML blockquotes provides a clean and easily digestible way to present key restaurant features. This structured approach improves readability and allows users to quickly scan for essential information.
Price Range: $10-$20
Cuisine: Italian
Hours: 11:00 AM – 8:00 PM
This simple structure, replicated for each relevant feature, offers a clear and concise summary, making it easy for users to compare different options. Information such as dietary options (vegetarian, vegan, gluten-free), delivery availability, and special offers can also be effectively integrated using this method.
Creating Descriptive Captions for Restaurant Images
A well-crafted caption complements the image, enhancing its impact. It shouldn’t just describe what’s in the picture; it should evoke the experience. Consider this example:
Indulge in our signature Margherita pizza, crafted with fresh basil, San Marzano tomatoes, and creamy mozzarella, all served in our cozy, rustic-chic dining room. The aroma alone is enough to transport you to the heart of Italy.
This caption doesn’t just describe the pizza; it paints a picture of the entire dining experience, appealing to both the visual and sensory aspects. Similarly, a caption for an image of a bustling restaurant might highlight the lively atmosphere and the friendly staff, inviting the user to imagine themselves enjoying the scene.
User Reviews and Ratings
User reviews and ratings are the lifeblood of any location-based service, especially those focused on finding the perfect lunch spot. They represent the collective wisdom of the crowd, offering invaluable insights beyond menu descriptions and star ratings. Understanding how these reviews are generated, aggregated, and potentially skewed is crucial for both businesses and consumers seeking authentic recommendations.
In the competitive landscape of the lunch market, user reviews act as powerful social proof. Positive reviews can dramatically increase a restaurant’s visibility and attract new customers, while negative reviews can severely impact its reputation and bottom line. This makes the analysis and management of online reviews a critical aspect of a restaurant’s overall strategy.
Review Aggregation and Display
Different platforms employ varying methods to aggregate and display user reviews. Some simply average the numerical ratings (e.g., a 4.5-star average), while others incorporate more sophisticated algorithms that consider factors like review recency, user activity, and the overall distribution of ratings. Visual representations often include star ratings, a summary of the overall sentiment (positive, negative, or neutral), and a selection of individual reviews. Platforms like Yelp and Google Maps, for example, often prioritize reviews deemed most helpful or relevant based on their algorithms, displaying them prominently. These algorithms often factor in factors such as length, detail, and the user’s overall review history. This means a shorter but insightful review from a frequent reviewer might be prioritized over a lengthy, rambling one from a first-time user.
Potential Biases and Inaccuracies in User Reviews
While user reviews offer valuable insights, it’s crucial to acknowledge potential biases and inaccuracies. For instance, reviews might be skewed by personal preferences (e.g., someone who dislikes spicy food might unfairly criticize a Mexican restaurant known for its spicy dishes). Furthermore, fake reviews, either positive or negative, can artificially inflate or deflate a restaurant’s rating. Businesses might attempt to manipulate reviews, while competitors might engage in “review bombing” to damage their reputation. Finally, reviews can be influenced by external factors like a bad day, a server’s mistake, or a temporary kitchen issue that doesn’t reflect the restaurant’s usual quality.
Characteristics of a Helpful User Review
A truly helpful user review provides detailed and objective information to potential customers. To be useful, a review should offer more than just a star rating. Consider the following aspects of a helpful review:
- Specific Details: Instead of saying “the food was great,” a helpful review would specify what dishes were particularly good and why (e.g., “The Pad Thai was perfectly balanced with fresh ingredients and a flavorful sauce”).
- Contextual Information: Mentioning the time of visit (e.g., busy lunch hour versus a quiet evening) can help others understand potential variations in service or wait times.
- Objectivity and Honesty: While personal preferences are understandable, a helpful review attempts to present a balanced perspective, acknowledging both positive and negative aspects.
- Clarity and Conciseness: Well-written reviews are easy to read and understand, conveying essential information efficiently.
- Relevant Information: Focus on aspects important to potential customers, such as food quality, service, ambiance, pricing, and cleanliness.