User Search Intent
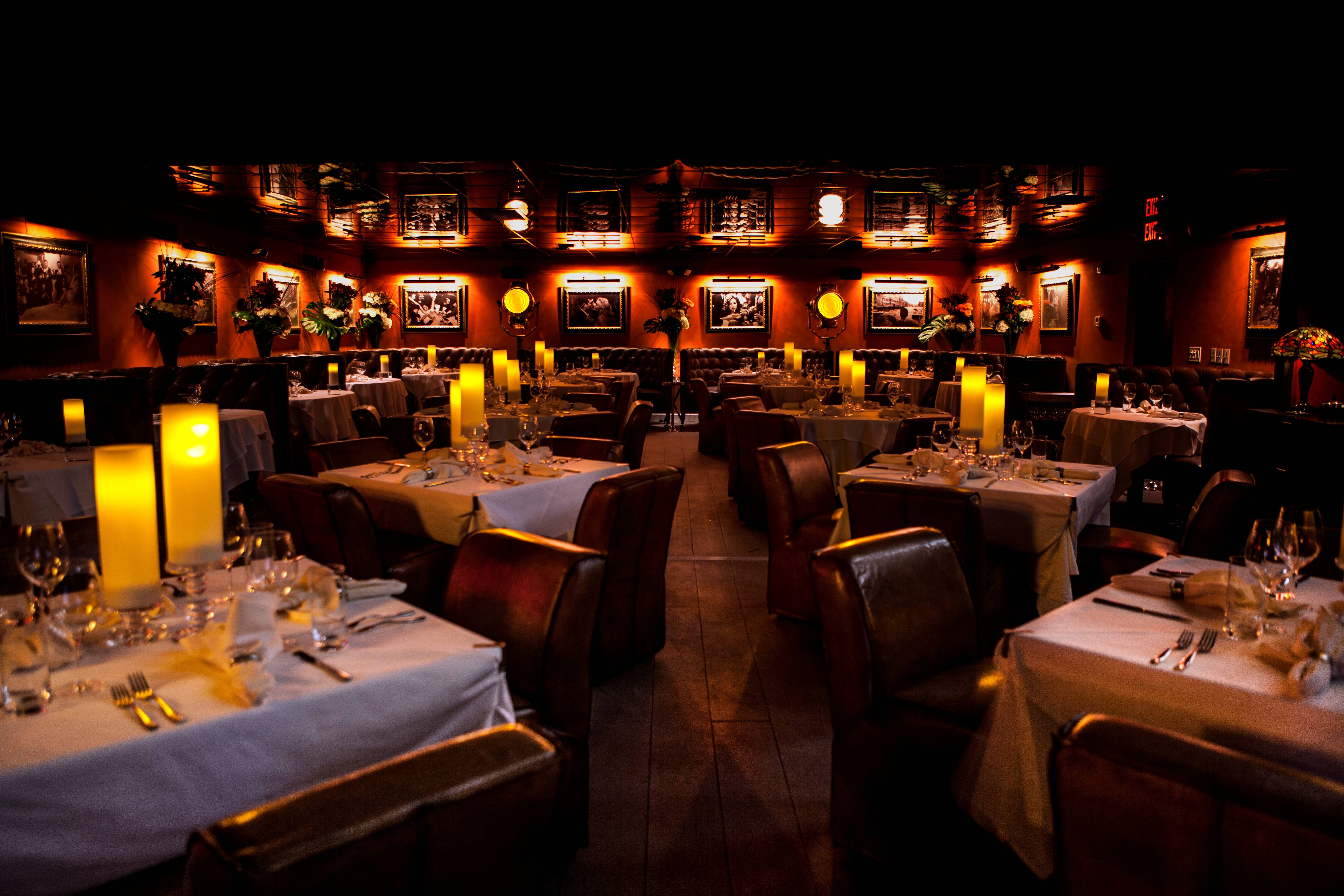
Understanding user search intent is paramount for any business, especially in the competitive landscape of online restaurant discovery. When a user searches for “nearby restaurant,” they’re not just looking for a list of establishments; they’re expressing a specific need at a particular moment. Deciphering this underlying intent is key to optimizing your online presence and attracting the right customers. This involves analyzing the various motivations behind the search, the contextual factors influencing the query, and ultimately, providing the most relevant and timely results.
The seemingly simple search phrase “nearby restaurant” masks a surprising diversity of user needs and desires. It’s a gateway to a complex interplay of hunger, convenience, occasion, and personal preferences. By dissecting these elements, we can better understand the user’s journey and tailor our offerings to meet their expectations. Ignoring this nuance risks losing potential customers to competitors who have mastered the art of intent-based optimization.
Search Intent Variations
The reasons behind a “nearby restaurant” search are multifaceted. Users might be ravenous and seeking immediate gratification, or they might be planning a future meal, looking for a specific type of cuisine, or scouting options for a special occasion. The context surrounding the search dramatically alters the user’s expectations and priorities. For instance, a spontaneous lunchtime search differs greatly from a carefully researched dinner reservation. Understanding this spectrum is crucial for effective targeting and conversion.
User Needs and Desires
A user’s needs extend beyond simple hunger. They might be seeking convenience (quick service, delivery options), a specific atmosphere (romantic, family-friendly), a particular type of food (Italian, vegan), or a certain price range. Some might prioritize reviews and ratings, while others focus on location and accessibility. Understanding these diverse needs requires a deep dive into user behavior and data analysis. This analysis will reveal patterns and trends that can inform marketing strategies and service improvements.
Contextual Factors
The context surrounding the search plays a vital role in shaping the user’s expectations. A user searching during lunchtime is likely looking for a quick and convenient option, whereas an evening search might indicate a more leisurely dining experience. The location context is also crucial; a search in a busy city center will differ significantly from one in a quiet suburban area. Moreover, the user’s device (mobile vs. desktop) and their previous online behavior (past searches, website visits) provide valuable clues about their intent.
Search Intent Matrix
This table summarizes the various facets of user search intent for “nearby restaurant”:
Search Intent | User Need | Location Context | Time Context |
---|---|---|---|
Quick lunch | Fast service, affordable price | Office area, shopping mall | Midday (12pm-2pm) |
Romantic dinner | Upscale atmosphere, fine dining | City center, waterfront | Evening (6pm-10pm) |
Family meal | Kid-friendly menu, spacious seating | Residential area, family-friendly establishment | Early evening (5pm-7pm) |
Spontaneous craving | Immediate availability, variety of options | Current location, nearby options | Anytime |
Restaurant Information Needs: Near By Restaurant
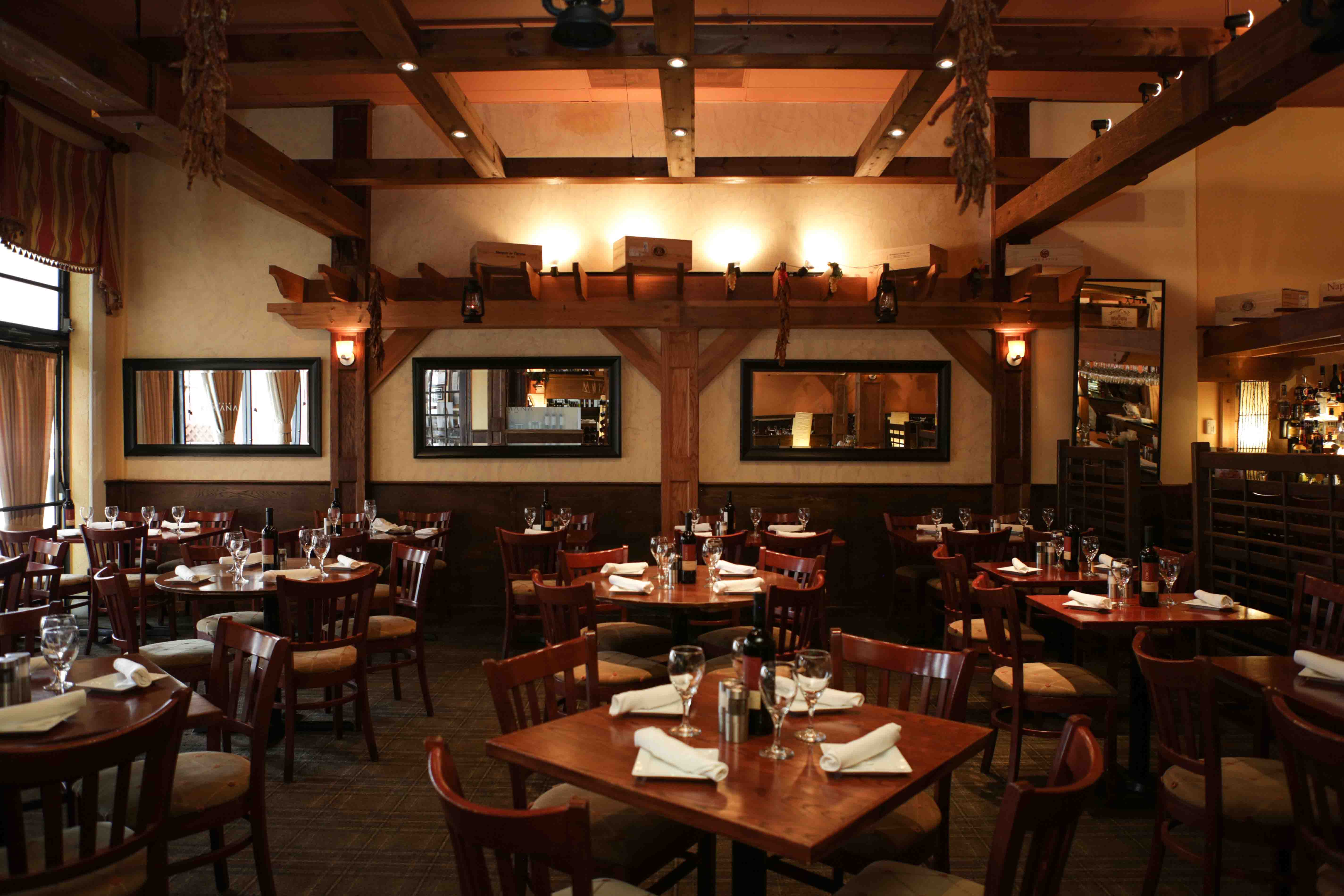
Understanding what information users seek when searching for nearby restaurants is crucial for optimizing your online presence and driving conversions. Failing to provide this readily accessible information leads to lost opportunities and frustrated customers. This section details the essential information users expect and how to present it effectively.
Essential Information for Restaurant Listings
Users searching for nearby restaurants need quick access to key details to make informed decisions. Providing this information clearly and concisely is paramount to attracting customers. Missing even one crucial piece of information can deter a potential diner.
- Restaurant Name: Clear and concise, ideally reflecting the restaurant’s style and cuisine.
- Address and Location: A complete address with easily accessible map integration. Consider including landmarks for easier identification.
- Cuisine Type: Clearly state the type of food served (e.g., Italian, Mexican, Seafood).
- Operating Hours: List daily hours, including any variations for weekends or holidays. Note any closures.
- Customer Ratings and Reviews: Display aggregated ratings from reputable platforms (e.g., Google, Yelp) alongside a sample of recent reviews.
- Menu (or Link to Menu): Provide a link to an online menu or, if space allows, a concise summary of popular dishes and price ranges.
- Photos: High-quality images of the restaurant’s ambiance, food, and exterior are essential for creating a positive first impression.
- Contact Information: Phone number and website (if applicable) for easy communication and reservations.
- Price Range: Indicate the average cost per person to help users budget their meal.
- Special Offers or Promotions: Highlight any current deals or discounts to incentivize immediate visits.
Visual Presentation of Restaurant Information, Near by restaurant
The visual presentation of information is just as important as the information itself. A cluttered or poorly designed interface will drive users away, regardless of how good your restaurant is.
Consider using clear typography, high-quality images, and a logical layout to present information effectively. For example, star ratings should be prominent, photos should be visually appealing and high-resolution, and the address should be easily copied or integrated with mapping services. Use color strategically to highlight key information. For example, using a distinct color for the price range can make it immediately noticeable.
User Interface Mock-up
The user interface should feature a clean, card-like design. Each restaurant listing would be a separate card with a prominent image at the top. Below the image, the restaurant name would be displayed in a large, bold font. Directly beneath the name, a concise description of the cuisine would appear. Customer ratings (e.g., 4.5 stars out of 5) would be displayed prominently using a standard star rating system. A small preview of recent reviews could be included, perhaps showing only the first sentence or two. The address would be clearly displayed, with a clickable link to open the location in a map application. Finally, the price range (e.g., $, $$, $$$) should be easily visible. This entire card could then be clickable to lead to a detailed restaurant page.
Information Categorization for Optimal User Experience
Organize information logically into categories to improve the user experience. A common approach is to group similar information together. For instance, contact information (phone, website) can be grouped under a “Contact” heading, while operational details (hours, price range) can be under an “Information” heading. This approach significantly enhances the overall usability and findability of the information presented.
Map Integration and Location Services
In today’s hyper-connected world, seamlessly integrating map functionality into your restaurant finder is no longer a luxury—it’s a necessity. Accurate and visually appealing map integration significantly enhances user experience, driving engagement and ultimately, more customers to your clients’ establishments. Without it, you’re leaving significant potential on the table.
Map integration allows users to quickly and easily locate nearby restaurants, filtering their search based on proximity. This crucial feature converts passive browsers into active diners, boosting conversion rates and establishing a competitive edge in the crowded online food scene. The key is not just having a map, but having a map that’s intuitive, informative, and visually appealing.
Visual Representation of Restaurant Locations
Effective visual representation of restaurant locations is critical for a positive user experience. Simply dropping markers on a map isn’t enough; you need to consider factors like density and visual clarity. For areas with a high concentration of restaurants, marker clustering is essential. This technique groups closely located markers into a single cluster, preventing map clutter and maintaining readability. The cluster itself can display the number of restaurants within it, expanding to reveal individual markers upon user interaction. Alternatively, heatmaps can be used to visually represent restaurant density across a larger area, providing a high-level overview before zooming into specific locations. Color-coding markers based on restaurant type (e.g., Italian, Mexican, etc.) or rating can further enhance visual organization and help users quickly find what they’re looking for. Consider also the size and style of the markers themselves; a clear, easily identifiable marker is crucial for usability.
Incorporating User Location Data
Accurately determining and using user location data is paramount for providing relevant search results. This requires obtaining user permission to access their location, typically through browser-based geolocation APIs. Once permission is granted, the system should use this data to center the map on the user’s current location and prioritize restaurants within a specified radius. Providing users with the ability to adjust this radius (e.g., 1 mile, 5 miles, 10 miles) gives them granular control over their search. Transparency is key; clearly indicate to the user how their location data is being used and allow them to disable location services if they choose. Remember, respecting user privacy is crucial for building trust and maintaining a positive brand image.
Map Provider Comparison
Several map providers offer robust APIs for integrating maps into your application. Google Maps Platform, for example, is a widely used and well-documented option offering extensive features including street view, directions, and place details. However, it comes with associated costs based on usage. OpenStreetMap, on the other hand, is a free and open-source alternative, providing a viable cost-effective solution, although its feature set might be slightly less extensive compared to Google Maps. Other providers such as Mapbox offer a balance between functionality and cost, providing a range of pricing tiers to suit different needs. When selecting a provider, carefully evaluate factors such as cost, feature set, ease of integration, and the quality of map data for your target area. Consider factors like the level of detail provided, accuracy of location data, and the availability of relevant features like street view or business information. Choosing the right provider can significantly impact the user experience and the overall success of your restaurant finder application.
Filtering and Sorting Options
Optimizing the user experience on your restaurant-finding platform hinges on providing robust filtering and sorting capabilities. Users need efficient ways to sift through numerous options and quickly identify the perfect place to dine. By implementing powerful filtering and sorting mechanisms, you dramatically improve user engagement and satisfaction, leading to increased conversions and a more loyal user base.
Filtering and sorting options allow users to refine search results based on specific criteria, significantly improving the efficiency of their search. This translates directly into a better user experience, leading to higher engagement and ultimately, more successful restaurant discoveries.
Cuisine Type Filtering
Users frequently search for restaurants based on their preferred cuisine. This filter allows users to select from a pre-defined list of cuisines (e.g., Italian, Mexican, Indian, American, etc.). The implementation should be straightforward, using checkboxes or a dropdown menu. For example, a user could select “Italian” and “Mexican” to see results that match either cuisine. Behind the scenes, this involves a simple database query filtering results based on the selected cuisine types. Consider allowing users to select multiple cuisines simultaneously for a more comprehensive search.
Price Range Filtering
Allowing users to filter by price range is critical. This filter can be implemented using a slider or a dropdown menu with predefined price brackets (e.g., $, $$, $$$, $$$$). The database query would then filter results based on the restaurant’s average price per person or a similar metric. This is essential for users on a budget or those looking for a specific level of fine dining.
Rating Filtering
Users often rely on ratings and reviews to make informed decisions. A star rating system (1-5 stars) with a filter allowing users to select a minimum rating (e.g., 4 stars and above) is highly effective. The algorithm would simply filter results based on the average rating of the restaurant, excluding any restaurants below the selected threshold. This filter significantly improves the quality of results presented to the user.
Dietary Restriction Filtering
Catering to dietary restrictions is crucial for inclusivity. This filter could include options such as vegetarian, vegan, gluten-free, halal, kosher, etc. Implementing this requires detailed information about each restaurant’s menu and offerings, accurately tagged in your database. The filtering algorithm would then match restaurants based on the user’s selected dietary restrictions, ensuring that only suitable options are displayed.
Sorting and Ranking Algorithms
Efficiently sorting and ranking restaurant results is vital for presenting the most relevant options first. A combination of factors can be used, prioritizing results based on user preferences and proximity. For example, a simple algorithm might prioritize:
- Proximity: Restaurants closest to the user’s location are ranked higher.
- Rating: Higher-rated restaurants are prioritized.
- Cuisine Preference: If the user has selected a cuisine, restaurants matching that cuisine are given higher priority.
- Price Range: Restaurants within the user’s specified price range are favored.
More sophisticated algorithms could incorporate machine learning techniques to learn user preferences over time and personalize the ranking further. For instance, a restaurant frequently visited by a user or one consistently viewed but not visited might receive a higher ranking due to implied interest.
Filtering and Sorting Flowchart
Imagine a flowchart. It begins with the user inputting search criteria (location, cuisine, price, rating, dietary restrictions). This input feeds into a filtering module that uses the database to retrieve matching restaurants. These filtered results then proceed to a sorting module that ranks them based on proximity, rating, and other selected preferences. Finally, the sorted results are displayed to the user. Each step involves database queries and algorithmic logic to ensure efficient processing and accurate results. The flowchart visually depicts this sequential process, illustrating how user preferences are translated into refined and ranked restaurant recommendations.
Visual Presentation of Restaurant Information
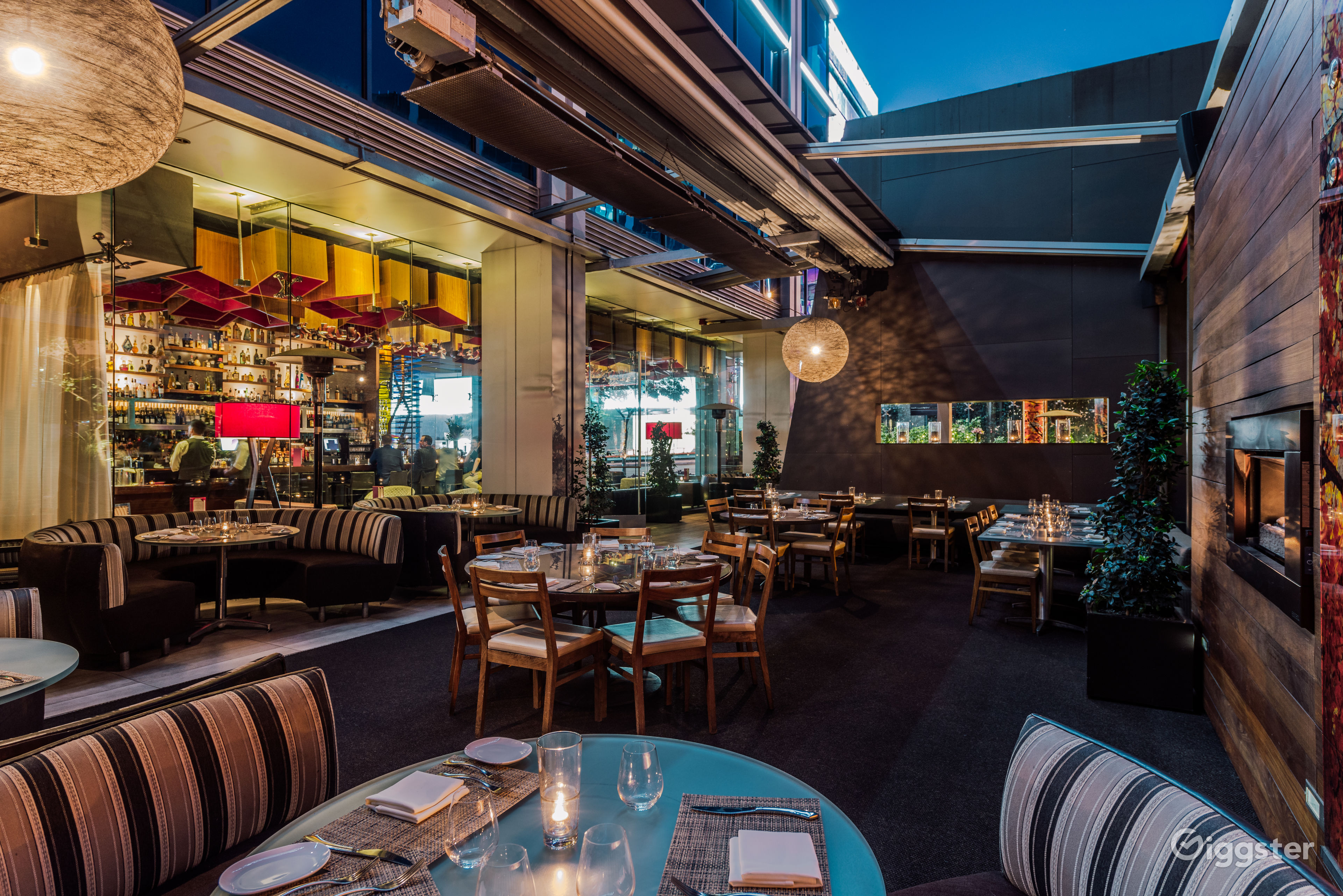
In today’s digital landscape, a restaurant’s online presence is as crucial as its physical location. Attracting customers hinges on more than just accurate information; it requires a compelling visual narrative that entices potential diners and sets the stage for a memorable experience. High-quality visuals are the cornerstone of this narrative, transforming a simple listing into an irresistible invitation.
High-quality images are paramount for showcasing restaurants effectively. They bridge the gap between the online world and the tangible experience, allowing potential customers to “see” the restaurant’s ambiance, food quality, and overall appeal before they even step inside. A blurry, poorly lit image can deter customers, while a crisp, professionally shot photograph can spark immediate interest and drive bookings. Think of it as your restaurant’s first impression – make it count.
Descriptive Captions for Restaurant Images
Compelling captions are more than just descriptions; they’re opportunities to highlight key selling points and evoke emotion. They should be concise, informative, and engaging.
For example, instead of simply stating “Our delicious pasta dish,” a more effective caption would be: “Hand-rolled pasta, slow-simmered tomato sauce, fresh basil – our signature Spaghetti alla Puttanesca is a taste of Italy in every bite.” Or, for a picture of the restaurant’s interior: “Relax in our cozy ambiance, perfect for a romantic dinner or a night out with friends. Our fireplace adds a touch of warmth to every evening.” These captions paint a vivid picture and create a desire to experience the restaurant firsthand.
Effective Use of Visual Elements to Convey Ambiance and Atmosphere
Visual elements extend beyond just food photography. Consider the overall aesthetic: the lighting, the décor, the arrangement of tables, even the silverware. These details subtly communicate the restaurant’s personality and target audience.
A brightly lit, modern restaurant with minimalist décor will appeal to a different clientele than a dimly lit, rustic trattoria with exposed brick walls and candlelight. High-quality images showcasing these details, combined with carefully chosen captions, can accurately convey the desired ambiance and atmosphere, ensuring that the online experience aligns with the physical reality. For instance, a series of images showcasing a bustling bar scene could emphasize a lively, social atmosphere, while images focusing on intimate table settings could highlight a romantic, quiet setting.
Visual Representation of a Restaurant Listing
Imagine a restaurant listing displayed as follows:

Restaurant Name
Near by restaurant – Cuisine Type: Italian
Address: 123 Main Street
Rating: 4.5 stars (based on 100 reviews)
This example showcases a prominent image, followed by key information presented in a clear, concise manner. The inclusion of ratings adds social proof and builds trust, while the calls to action encourage immediate engagement. The use of consistent fonts and colors maintains a professional and visually appealing layout. Consider using a responsive design to ensure the layout adapts seamlessly to various screen sizes. This ensures a consistent and positive user experience across all devices.
Handling User Preferences and Personalization
Personalizing the restaurant discovery experience is crucial for driving engagement and loyalty. By leveraging user data, we can move beyond generic search results and offer highly relevant recommendations, increasing the likelihood of a user finding their perfect meal. This involves understanding user behavior, learning from their past interactions, and intelligently predicting their future needs.
Understanding and utilizing user history and preferences is paramount for delivering personalized restaurant recommendations. This goes beyond simply remembering past searches; it’s about building a sophisticated profile that anticipates user needs. For example, a user who frequently searches for “vegan Thai food” should be prioritized with relevant options, even if their current search is broader, like “restaurants near me.” This proactive approach enhances user experience and increases the chance of conversion.
Restaurant Suggestion Based on Past Searches and Choices
The system can track past searches and choices to create a behavioral profile. For instance, if a user consistently selects restaurants with high ratings, a “favorites” section can be created, highlighting similarly rated establishments. Similarly, if a user frequently searches for specific cuisines or price ranges, future searches can pre-filter results to match their preferences, saving time and improving efficiency. This is achieved through a combination of analysis, rating analysis, and location data. The algorithm could also analyze the frequency of visits to particular restaurant types (e.g., fast food, fine dining) and prioritize recommendations accordingly. For example, a user who frequently orders fast food during the weekday might see fast food options prioritized in their midday search results.
Algorithms for Learning User Preferences Over Time
Collaborative filtering algorithms are highly effective in learning user preferences. These algorithms analyze the choices of similar users to identify patterns and make personalized recommendations. For example, if User A and User B both frequently choose Italian restaurants with a high rating, and User A has also rated a new Italian restaurant highly, the algorithm might suggest that same restaurant to User B. Content-based filtering is another approach; it analyzes the characteristics of restaurants a user has previously interacted with (e.g., cuisine, price range, location) and recommends similar establishments. Reinforcement learning can also be employed; this allows the system to learn from user feedback (e.g., clicks, ratings, bookings) and adjust its recommendations accordingly, continually optimizing the personalization process. The system can then adapt its weighting of different factors based on the user’s consistent interactions. For instance, if a user consistently ignores price suggestions, the algorithm might downplay the price filter in future recommendations.
Personalization Techniques for Restaurant Search Results
Several personalization techniques can enhance restaurant search results. A well-designed system might incorporate all of these for a truly tailored experience.
- Personalized Recommendations: Suggesting restaurants based on past searches, ratings, and preferences.
- Customizable Filters: Allowing users to save preferred filters (e.g., cuisine, price range, dietary restrictions) for easy reuse.
- Location-Based Suggestions: Prioritizing restaurants based on the user’s current location and frequently visited areas.
- Personalized Ranking: Adjusting the order of search results based on user preferences, rather than solely relying on popularity or proximity.
- Personalized Offers and Promotions: Showing targeted discounts or promotions based on user history and preferences.
- Contextual Recommendations: Offering different recommendations based on the time of day (e.g., breakfast suggestions in the morning, dinner suggestions in the evening).
- A/B Testing of Recommendations: Continuously testing different recommendation algorithms and personalization strategies to optimize results.