Understanding User Intent Behind “Nearest Place to Eat”
:max_bytes(150000):strip_icc()/restaurant-438385_1920-57a60ed43df78cf459e7b762.jpg)
The seemingly simple search query, “nearest place to eat,” reveals a complex tapestry of user needs and motivations. Understanding these nuances is crucial for businesses aiming to capture this high-intent traffic and convert it into paying customers. The urgency, the specific desires, and the contextual factors all play a significant role in shaping the user’s decision-making process.
The urgency inherent in this search is often high. A hungry user isn’t likely to browse extensively; they need a quick and relevant answer. This immediacy necessitates a fast-loading, accurate, and user-friendly search result. Delay can lead to frustration and a switch to a competitor, highlighting the importance of optimized search engine results and a seamless user experience.
User Needs Implied by the Search Query
The search “nearest place to eat” can stem from various needs. A user might be looking for a quick, inexpensive bite, a sit-down restaurant for a special occasion, or something in between. They may have dietary restrictions, be seeking a specific type of cuisine, or prioritize ambiance and service. The query lacks specificity, leaving the search engine to interpret the user’s implicit needs based on context and location data. For example, a search performed near a stadium after a game suggests a different need than one performed during a business lunch break in a downtown area.
Urgency Associated with the Search
The level of urgency significantly impacts the user’s behavior. Someone searching while already experiencing hunger will prioritize speed and proximity over extensive reviews or price comparisons. In contrast, a user planning a dinner date might invest more time in exploring options and reading reviews. This difference underscores the importance of presenting results tailored to the likely urgency of the search. Real-time updates on wait times and availability could be a valuable addition for users with higher urgency. Imagine a family with hungry children – their need for immediate gratification is paramount.
Factors Influencing Restaurant Choice
Numerous factors influence a user’s final restaurant selection. Price, location, cuisine type, reviews, ambiance, and operating hours are all key considerations. Accessibility for those with disabilities, parking availability, and the presence of outdoor seating are also significant factors for many users. The availability of online ordering and delivery options further influences the decision, particularly for users prioritizing convenience. Consider a business traveler – their choice might heavily favor speed and efficiency over ambiance.
User Persona: The Hungry Traveler
Let’s create a user persona: Sarah, a 35-year-old businesswoman traveling for a conference. She’s arrived in a new city, exhausted and hungry after a long flight. Her phone battery is low, and she needs a quick and reliable lunch option nearby. She values convenience, speed, and a decent meal at a reasonable price. She’s less concerned with ambiance and more focused on satisfying her immediate hunger. This persona highlights the need for clear, concise information presented quickly and efficiently in search results. She likely won’t spend time browsing extensive menus or detailed reviews. A simple list of nearby restaurants with basic information like price range and cuisine type would suffice.
Data Sources for Finding Nearby Eateries
Finding the nearest place to eat shouldn’t be a culinary quest. The right data source can transform your search from a frustrating hunt to a seamless experience. Choosing wisely hinges on understanding the strengths and weaknesses of different platforms, each offering a unique perspective on the vast landscape of local restaurants.
Nearest place to eat – Several platforms dominate the market for locating nearby eateries, each employing different methodologies and data collection practices. This leads to variations in accuracy, comprehensiveness, and the type of information presented. Understanding these differences is key to optimizing your search and finding the perfect meal, quickly and efficiently.
Google Maps Data Structure and Capabilities
Google Maps boasts a massive user base and an incredibly detailed database. Its strength lies in its comprehensive coverage and generally accurate location data. Information is typically structured with a prominent map display showing the restaurant’s location, followed by a concise summary including user ratings, reviews, photos, hours of operation, contact details, and often a menu snippet. For example, searching for “pizza near me” on Google Maps would yield a list of pizzerias, each with a map pin, star rating, and short description. However, while Google Maps excels at location accuracy and broad coverage, it might lack the depth of culinary detail found in other specialized platforms. It also heavily relies on user-generated content, meaning the accuracy of reviews and information can vary.
Yelp Data Structure and Capabilities
Yelp focuses specifically on user reviews and business ratings. Its data structure centers around detailed user reviews, photos uploaded by users, and business profiles that often include menus, hours, price ranges, and contact information. The strength of Yelp lies in its community-driven approach; its wealth of reviews provides a nuanced perspective on the dining experience. Searching for “best Thai food” on Yelp would yield a curated list emphasizing user ratings and detailed reviews. However, Yelp’s reliance on user-generated content can also be a weakness, making it susceptible to biased or inaccurate reviews, and its coverage might be less comprehensive in smaller or less-populated areas compared to Google Maps.
Foursquare Data Structure and Capabilities
Foursquare’s data structure emphasizes location-based check-ins and user recommendations. It offers a detailed profile for each venue, including user ratings, photos, check-in counts, and lists curated by users and Foursquare itself. The platform’s strength is its ability to provide insights into the popularity and trends of various eateries. For instance, searching for “trendy brunch spots” on Foursquare might reveal hidden gems and popular locations based on user check-ins and curated lists. However, Foursquare’s database might be less comprehensive than Google Maps, particularly regarding detailed menu information or extensive reviews.
Comparison of Data Sources
The following table summarizes the key differences between Google Maps, Yelp, and Foursquare as data sources for finding nearby eateries:
Feature | Google Maps | Yelp | Foursquare |
---|---|---|---|
Accuracy of Location Data | High | Medium | Medium |
Comprehensiveness of Coverage | High | Medium | Medium-Low |
Depth of Restaurant Information | Medium | High | Medium |
Reliance on User-Generated Content | High | High | High |
Focus | Maps & Navigation | Reviews & Ratings | Check-ins & Recommendations |
Factors Influencing Restaurant Recommendations
Finding the perfect eatery near you isn’t just about proximity; it’s a complex algorithm balancing several crucial factors. Understanding these factors and how they interact is key to building a truly effective restaurant recommendation system – one that consistently delivers results users love. This involves more than just throwing a bunch of data together; it requires strategic weighting and contextual awareness.
The ranking of restaurants is a dynamic process influenced by a multitude of variables, all vying for influence in the final recommendation. These variables are weighted differently depending on the user’s specific needs and preferences at the moment of the search. Think of it as a finely tuned engine, constantly recalibrating based on the input it receives.
Restaurant Ranking Factors
Several key factors contribute to a restaurant’s ranking in a search for “nearest place to eat.” These factors work in concert, and their relative importance changes depending on the user’s context.
- Proximity: This is usually the most significant factor. Users generally want the closest option, especially when hungry!
- Ratings and Reviews: High average ratings and a large number of positive reviews strongly indicate quality and customer satisfaction.
- Cuisine Type: A user searching for “Italian food near me” will prioritize Italian restaurants over others, regardless of proximity or rating.
- Price Range: Budget plays a critical role. A user looking for a cheap lunch will prioritize affordable options, while someone celebrating an anniversary might prefer a more expensive establishment.
- Opening Hours: A restaurant’s current operating status is essential. A place closed for the night won’t be a useful recommendation, no matter how highly rated it is.
- Dietary Restrictions: Increasingly important, this filters restaurants based on specific dietary needs (vegetarian, vegan, gluten-free, etc.).
Contextual Weighting of Factors
The relative importance of these factors shifts dramatically based on user context. Consider a busy professional grabbing a quick lunch versus a family planning a celebratory dinner. The weighting would differ significantly.
For the busy professional, proximity and speed of service (often implied by reviews mentioning quick service) might outweigh ratings or cuisine type. They might prioritize a nearby sandwich shop over a highly-rated but further-away restaurant. The family, however, might prioritize higher ratings, a specific cuisine, and a suitable atmosphere over sheer proximity.
Hypothetical Scenario: Restaurant Recommendation in Action
Imagine a user searches for “nearest place to eat” at 7 PM on a Friday night. They are looking for something relatively affordable and prefer Italian food. Let’s say three restaurants are within a reasonable distance:
- Restaurant A: Italian, 4.5 stars, $25 average price, 1 mile away, open until 10 PM.
- Restaurant B: Mexican, 4 stars, $15 average price, 0.5 miles away, open until 11 PM.
- Restaurant C: Italian, 3.5 stars, $10 average price, 0.2 miles away, open until 9 PM.
While Restaurant B is closest, the user’s preference for Italian food significantly boosts Restaurant A and C. Restaurant C, despite its proximity and affordability, has lower ratings, making Restaurant A the most likely recommendation. The slight increase in price and distance is offset by the higher ratings and the preferred cuisine type. The system prioritizes user preference, but proximity still plays a role.
Visual Representation of Factor Interaction
The decision-making process can be visualized as a flowchart. The user’s search initiates the process. Each factor (proximity, ratings, cuisine, price, hours) is evaluated and assigned a weighted score based on the user’s search query and contextual information. These scores are then combined to generate a ranked list of restaurants. Restaurants with higher combined scores appear higher in the list. A simple textual representation would show a series of decision points (e.g., “Is the cuisine type a match?”, “Is the price within budget?”, “Is it open now?”), each leading to different branches of the flowchart. The final branch indicates the recommended restaurant.
Displaying Results to the User
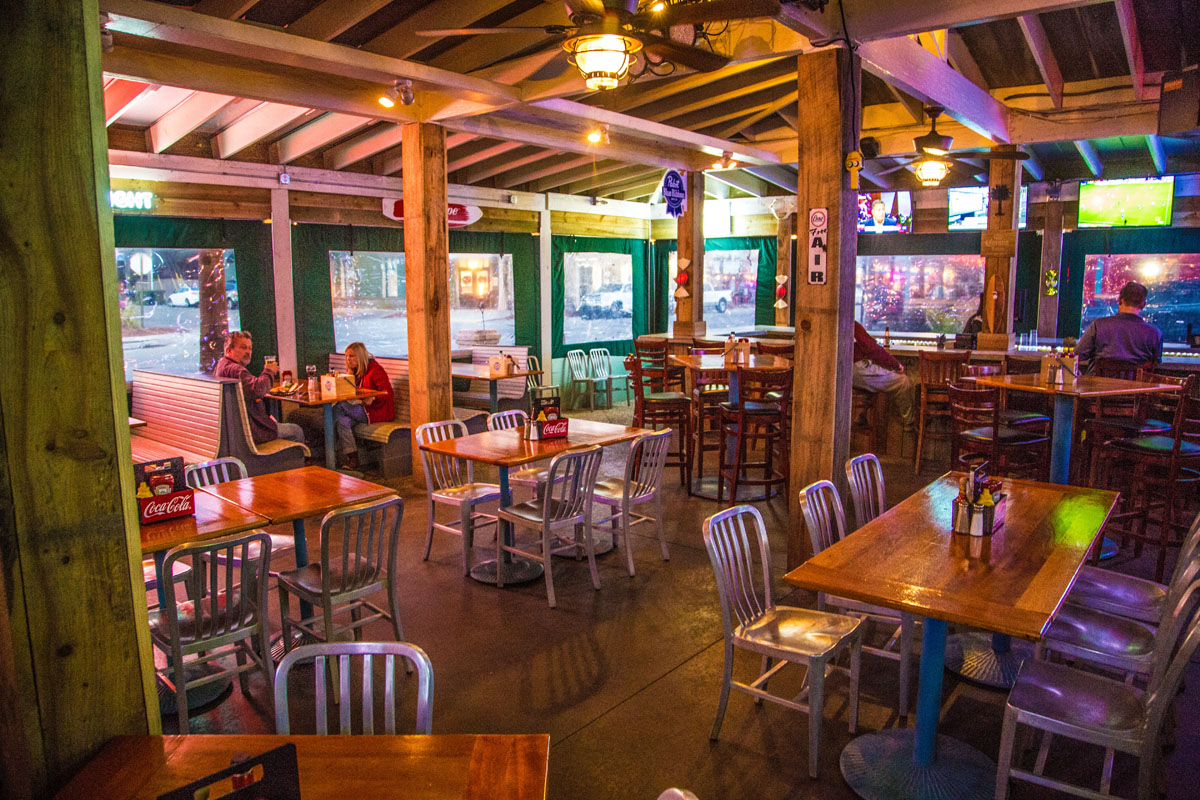
The user experience hinges on how effectively you present search results for nearby eateries. A poorly designed interface can lead to frustration and lost conversions, while a well-crafted one can significantly boost user satisfaction and engagement. Think of it as the culmination of your entire process – all the data gathering, processing, and filtering culminates in this final display. Make it count.
A seamless and intuitive presentation is paramount. We need to go beyond simply listing restaurants; we need to create a visually appealing and informative experience that helps users quickly find what they’re looking for. This involves strategic use of maps, clear information architecture, and a thoughtful visual hierarchy.
User Interface Design: Maps and Restaurant Details
The ideal user interface incorporates an interactive map prominently displaying search results. Each restaurant should be represented by a clearly identifiable marker, perhaps using different icons to represent cuisine types (a pizza slice for Italian, a fork and knife for general dining, etc.). Clicking on a marker should instantly reveal a concise summary of the restaurant, including its name, address, rating (if available), and perhaps a small preview image. This visual representation provides immediate context and allows users to quickly scan and compare options. Consider using a zoom function and possibly street view integration for enhanced user experience. Imagine the user looking at a map of their neighborhood, easily identifying restaurants based on proximity and visual cues. They can then drill down into specific listings for detailed information.
Organizing Restaurant Information
Clarity and conciseness are key when presenting restaurant information. Avoid overwhelming the user with unnecessary details. Prioritize crucial information such as the restaurant’s name, rating (e.g., stars from Yelp or Google Reviews), cuisine type, price range, address, operating hours, and distance from the user’s location. This information should be presented in a logical and easily digestible format, perhaps using a consistent layout for each listing. Think of a clean, card-like layout for each restaurant, where the most important information is prominently displayed at the top, with less critical details expandable upon request. This minimizes visual clutter and enhances scannability.
Visual Hierarchy and Information Prioritization
Visual hierarchy guides the user’s eye through the information presented. Use size, color, contrast, and spacing to emphasize key elements. For example, the restaurant’s name should be the largest and most prominent element, followed by its rating and cuisine type. Use clear visual separators between different restaurant listings to prevent the page from feeling cluttered and overwhelming. This is crucial for improving user comprehension and minimizing cognitive load. A visually appealing and well-structured page is more likely to be understood and engaged with, leading to better user experience.
Best Practices for Presenting Restaurant Information
- Prioritize Key Information: Name, rating, cuisine, price range, and distance should be immediately visible.
- Use Clear Visual Cues: Employ icons, color-coding, and consistent formatting to enhance readability.
- Incorporate User Reviews: Display snippets of positive reviews to build trust and provide social proof.
- Show High-Quality Images: Include attractive photos of the restaurant’s exterior and food.
- Offer Filtering and Sorting Options: Allow users to filter by cuisine, price range, rating, and other relevant criteria.
- Provide Clear Call-to-Actions: Make it easy for users to view the restaurant’s menu, website, or directions.
- Ensure Mobile Responsiveness: The interface must be optimized for seamless viewing on various devices.
Handling Edge Cases and Errors: Nearest Place To Eat
Building a robust “nearest place to eat” application requires anticipating and gracefully handling various scenarios that can disrupt the user experience. Ignoring potential errors can lead to frustrated users and a damaged reputation. A well-designed error-handling system is crucial for providing a seamless and reliable service. This section details strategies for addressing common issues and providing helpful feedback to the user.
Error handling isn’t just about displaying a message; it’s about providing context and suggesting solutions. Users need to understand *why* something went wrong and what they can do to fix it. This proactive approach significantly improves user satisfaction and builds trust in your application.
No Restaurants Found Near User’s Location
This is a common scenario, particularly in rural areas or locations with limited restaurant density. Simply displaying a generic “No restaurants found” message is insufficient. Instead, the application should provide more context and potential solutions. For instance, it could suggest widening the search radius, checking the accuracy of the user’s location, or searching for a different type of cuisine.
The application could also provide a visual representation of the search area, allowing the user to understand the geographical scope of the search. A map showing the user’s location with a circle indicating the search radius would be highly beneficial. If no results are found even with a significantly expanded radius, a message such as, “No restaurants found within a 20-mile radius. You might consider broadening your search criteria or checking for restaurants in nearby towns,” would be far more informative than a simple “No results” message.
Inaccurate Location Data
GPS data can be unreliable, especially indoors or in areas with weak signals. If the application suspects inaccurate location data, it should prompt the user to verify their location. This could involve displaying a map with their current location pinpointed and offering the option to manually adjust the location. A message like, “Your location appears to be inaccurate. Please verify your location on the map or manually adjust it for better search results.” would guide the user towards a solution.
Consider incorporating a mechanism to allow users to manually enter their address if GPS fails. This provides a fallback option for users experiencing location-related problems.
Network Errors
Network connectivity issues can prevent the application from accessing restaurant data. The application must gracefully handle these situations by displaying a clear message indicating the network problem and suggesting actions such as checking the internet connection or trying again later. For example, “We’re having trouble connecting to the internet. Please check your connection and try again.” Adding a visual indicator, such as a spinning loading icon, can provide immediate feedback to the user that the application is attempting to connect.
Beyond a simple message, consider implementing retry logic. The app could automatically attempt to reconnect after a short delay, perhaps with a visual countdown timer, providing a user-friendly experience while addressing the network error. If the error persists after several attempts, a more persistent error message can be displayed.
Data Errors, Nearest place to eat
Inaccurate or outdated restaurant data can lead to incorrect recommendations or missing entries. While the application can’t directly fix data errors, it can implement strategies to minimize their impact. For example, it could prioritize data from reliable sources and display a warning if the data source is known to be unreliable. The application could also allow users to report inaccurate information.
Regular data updates are crucial to ensure accuracy. A system for flagging potentially outdated information, perhaps based on last-updated timestamps, can help maintain data quality.
Improving the User Experience
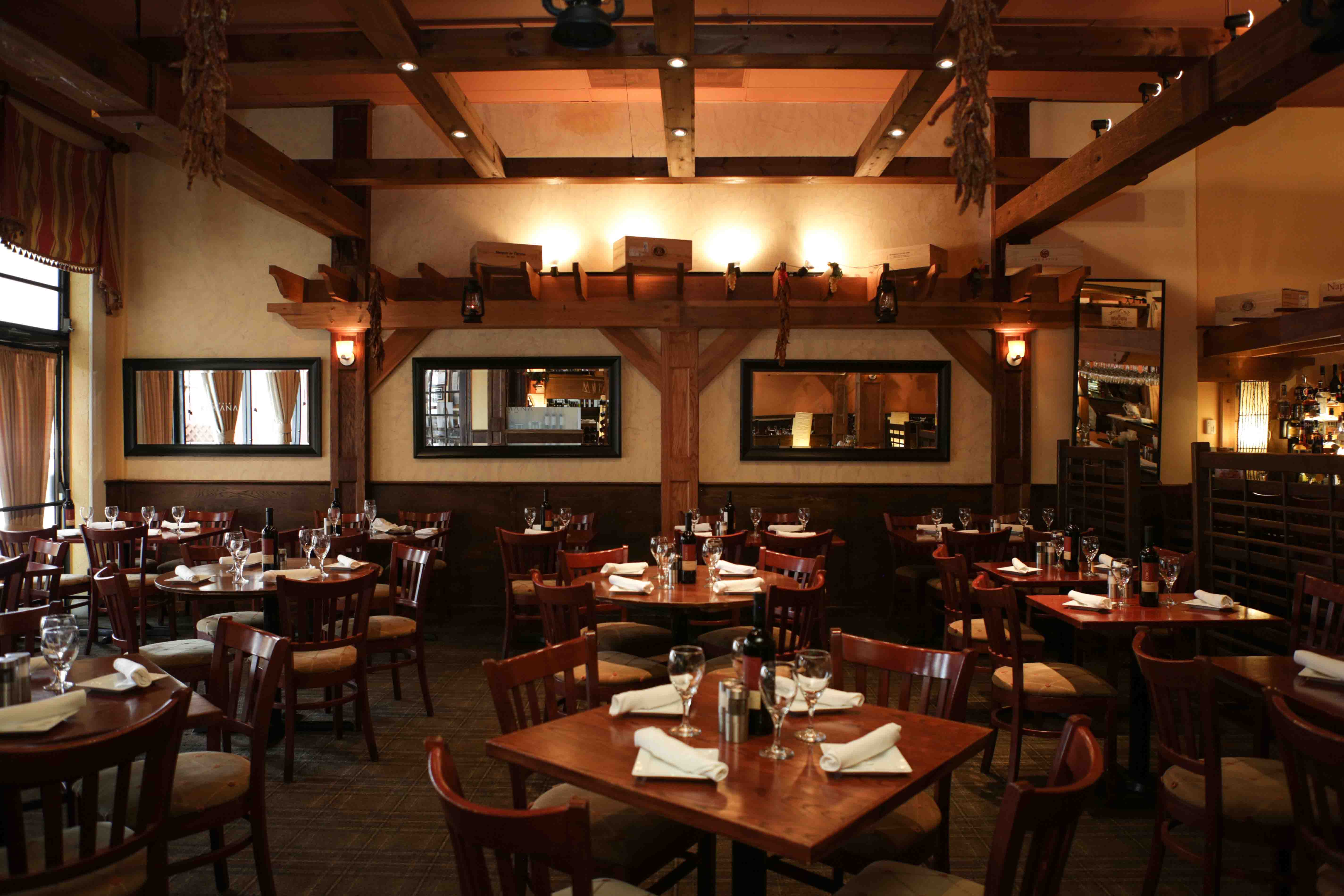
Optimizing the user experience (UX) for a “nearest place to eat” application is crucial for driving engagement and building a loyal user base. A seamless and intuitive experience will not only encourage repeat usage but also foster positive word-of-mouth marketing. Focusing on personalization, efficient information display, and accessibility ensures that the app caters to a broad audience and maximizes its value proposition.
Personalization significantly enhances user satisfaction. By leveraging user data, the application can offer more relevant and appealing restaurant recommendations. This goes beyond simply showing proximity; it’s about understanding individual tastes and dietary needs.
Personalized Recommendations Based on User Preferences and History
Effective personalization requires a multi-pronged approach. First, the app should allow users to explicitly state their preferences – cuisine type, price range, dietary restrictions (vegetarian, vegan, gluten-free, etc.), and preferred ambiance (casual, fine dining). This information can be collected during the initial signup process or through an easily accessible settings menu. Secondly, the app should passively learn user preferences through their past searches and selections. For example, if a user frequently searches for Italian restaurants, the algorithm should prioritize Italian options in future searches, even without explicit preference settings. Finally, integrating user reviews and ratings allows for a more nuanced understanding of individual tastes. If a user consistently rates highly-rated seafood restaurants, the algorithm should prioritize similar establishments. Consider implementing a “thumbs up/thumbs down” system after each restaurant display to further refine recommendations.
Effective Incorporation of User Reviews and Ratings
User reviews are gold; they are social proof. Displaying reviews prominently and strategically is essential. Don’t just show a numerical average rating; display snippets of actual reviews, highlighting both positive and negative aspects. Prioritize reviews that are recent and detailed. Visual cues, such as star ratings, can help users quickly assess the overall sentiment. Consider implementing a system to filter reviews based on factors like cuisine type or dietary restrictions. For instance, a user searching for vegan restaurants should see only reviews from users who identified as vegans or vegetarians. Furthermore, visually highlighting key aspects of reviews, such as mentions of specific dishes or service quality, can greatly improve the decision-making process. Think of a visual system where mentions of “great service” are highlighted in green and “long wait times” in red.
Accessibility Features for Users with Disabilities
Accessibility is not just a matter of compliance; it’s a matter of inclusivity. A truly user-friendly application caters to everyone. Implementing features such as screen reader compatibility, sufficient color contrast, and keyboard navigation ensures that users with visual or motor impairments can easily access and utilize the application. Consider providing options for adjusting font sizes and styles. Implementing alternative text descriptions for images ensures that screen readers can accurately convey visual information to visually impaired users. Geolocation services should be designed with privacy in mind, allowing users to manage their location sharing permissions effectively. This is particularly important for users concerned about data privacy and security. Compliance with WCAG (Web Content Accessibility Guidelines) is paramount.