Understanding User Intent Behind “Good Dinner Places Near Me”
The search query “good dinner places near me” reveals a user with a clear and immediate need: to find a suitable restaurant for dinner within a convenient location. This seemingly simple query, however, masks a complex interplay of factors influencing the user’s decision-making process. Understanding these nuances is crucial for businesses aiming to attract customers through effective online marketing and search engine optimization.
The user’s intent is multi-faceted, extending beyond simply locating a restaurant. We can discern several key underlying needs shaping their search.
User Needs Implied by the Search Query
The search “good dinner places near me” implies three primary user needs: convenience, quality, and a specific dining experience. First, convenience is paramount; the user wants a restaurant easily accessible. Second, the term “good” indicates a desire for a positive dining experience, encompassing factors like food quality, service, and atmosphere. Third, the implicit context of “dinner” suggests a particular type of meal and potentially a specific atmosphere, formality, or cuisine preference. These needs are interconnected and influence the user’s final choice.
Factors Influencing the Definition of “Good”
A user’s perception of a “good” dinner place is subjective and heavily influenced by various factors. Price plays a significant role; some users seek budget-friendly options, while others prioritize fine dining experiences. Ambiance is another crucial element; the desired atmosphere might range from casual and relaxed to sophisticated and romantic. Cuisine preferences significantly impact the choice; a user might be searching for Italian, Mexican, or a specific type of cuisine. Finally, reviews and ratings from other diners often heavily influence the perception of “good,” providing social proof and validation. For example, a highly-rated steakhouse might be considered “good” by a user seeking a special occasion dinner, while a casual burger joint with high ratings might be considered “good” by a user seeking a quick and satisfying meal.
The Importance of Proximity and its Interpretation
The phrase “near me” highlights the crucial role of location in the user’s search. The definition of “near” is highly subjective and context-dependent. It can range from a few blocks away for a user in a densely populated urban area to several miles for someone in a suburban or rural setting. The user’s mode of transportation (walking, driving, public transit) further influences their perception of proximity. Furthermore, “near me” can be interpreted literally based on the user’s GPS location, or it might encompass a broader area defined by the user’s mental map of their neighborhood or familiar surroundings. For instance, a user might consider a restaurant a 15-minute drive “near” if they are accustomed to longer commutes. Conversely, a user in a walkable city center might only consider restaurants within a 5-minute walk as “near.”
Data Sources for Restaurant Information
Finding the perfect dinner spot requires more than just a hunch. Leveraging the right data sources is crucial for making informed decisions, ensuring a delightful culinary experience, and avoiding potential disappointments. This involves understanding the strengths and weaknesses of various platforms to build a comprehensive picture of local restaurants.
Good dinner places near me – The digital age offers a wealth of information about restaurants, far exceeding the limitations of traditional methods. By strategically utilizing multiple sources, you can cross-reference details, verify accuracy, and obtain a holistic view of a restaurant’s offerings, quality, and overall appeal. This approach allows you to make choices based on reliable data rather than relying on chance or limited personal experiences.
Potential Data Sources for Restaurant Information
Several online and offline platforms provide valuable data about restaurants. Choosing the right sources depends on your specific needs and priorities. Some sources offer broader overviews, while others focus on specific aspects like reviews or menus.
- Online Review Sites (e.g., Yelp, Google Maps, TripAdvisor): These platforms aggregate user reviews, ratings, and photos, providing a user-generated perspective on a restaurant’s quality and atmosphere.
- Restaurant Websites: Official restaurant websites often provide detailed menus, hours of operation, contact information, and sometimes even online ordering capabilities.
- Social Media Platforms (e.g., Instagram, Facebook): Social media can offer a glimpse into a restaurant’s ambiance, food presentation, and customer interactions through photos, videos, and posts.
- Restaurant Aggregators (e.g., OpenTable, Resy): These platforms specialize in restaurant reservations and often include menus, photos, and user ratings.
- Local Directories and Guides: Print or online local directories (like city guides or magazines) may list restaurants with basic information and sometimes editorial reviews.
Comparison of Data Sources: Yelp vs. Restaurant Websites
Comparing different data sources highlights their unique strengths and limitations. Let’s analyze Yelp and restaurant websites as examples. Both offer valuable information, but their approaches and resulting data differ significantly in accuracy and comprehensiveness.
Yelp: Strengths include a vast user-generated database providing diverse opinions and experiences. Weaknesses include the potential for biased or fake reviews, and the lack of control over information accuracy. The sheer volume of reviews can also make it challenging to discern genuine feedback from outliers.
Restaurant Websites: Strengths lie in the control and accuracy of information provided directly by the restaurant. Weaknesses include potential for self-promotion bias and limited customer perspectives. Information may not always be up-to-date, especially concerning menu changes or special offers.
Hypothetical Data Structure for Restaurant Information
To effectively manage and utilize restaurant data, a structured approach is essential. The following table Artikels a hypothetical data structure that could be used to store key information for effective analysis and recommendation.
Restaurant Name | Cuisine | Price Range | User Rating (Average) |
---|---|---|---|
The Italian Place | Italian | $$ | 4.5 |
Spicy Tacos | Mexican | $ | 4.2 |
Sushi House | Japanese | $$$ | 4.8 |
Restaurant Recommendation Strategies
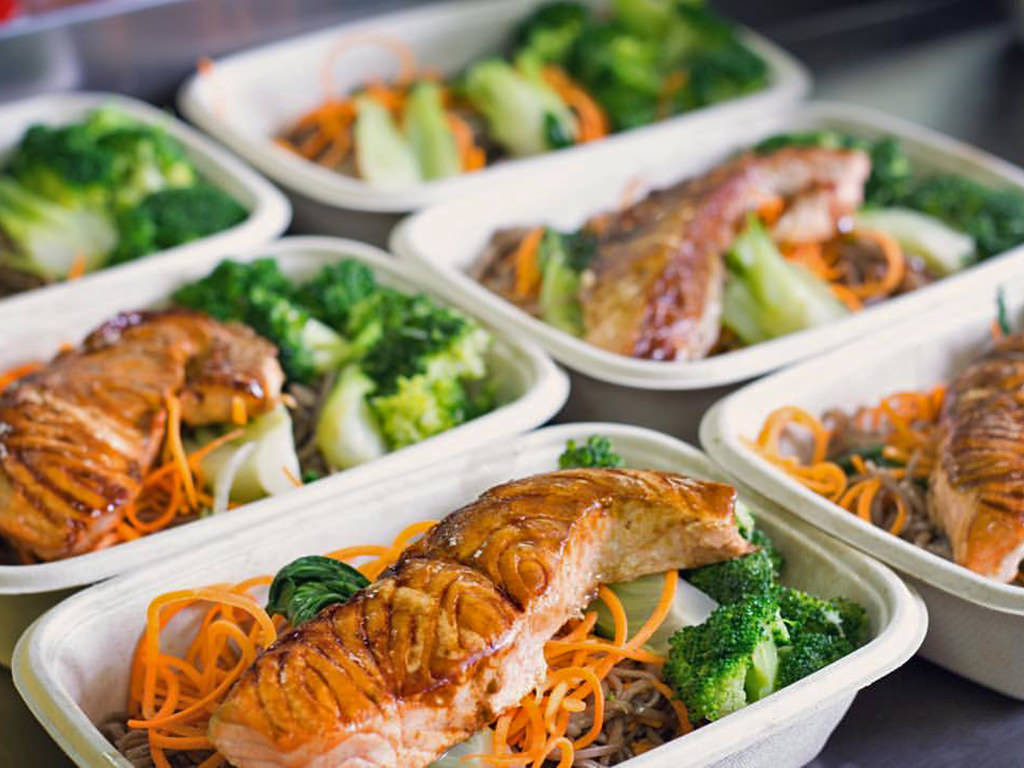
Optimizing restaurant recommendations requires a multi-faceted approach that leverages user data, sophisticated algorithms, and a deep understanding of user behavior. The goal isn’t just to find *a* restaurant, but to find the *right* restaurant for each individual user, maximizing satisfaction and driving repeat business. This involves intelligent filtering, personalized suggestions, and the seamless integration of user feedback.
Content-Based Filtering
Content-based filtering recommends restaurants similar to those a user has liked in the past. This approach analyzes the characteristics of restaurants the user has rated highly – cuisine type, price range, location, ambiance (e.g., casual vs. fine dining), and even specific dishes – and identifies restaurants with similar attributes. For example, if a user consistently rates highly-rated Italian restaurants in a specific price range, the system will prioritize similar options. The algorithm uses a vector representation of each restaurant’s features, calculating similarity scores between the user’s preference profile and the restaurant profiles. A high similarity score indicates a strong recommendation. This method works well for users with established preferences, providing familiar and comfortable choices. However, it can lead to a “filter bubble,” limiting exposure to new and potentially exciting options.
Collaborative Filtering
Collaborative filtering leverages the preferences of other users with similar tastes. This approach identifies users with similar rating patterns and recommends restaurants that those users have enjoyed. For instance, if users A and B both highly rate specific sushi restaurants and Italian trattorias, and user A has also rated a particular Mexican restaurant highly, the system might recommend that Mexican restaurant to user B. This method excels at discovering hidden gems and expanding user horizons beyond their established preferences. However, it requires a substantial amount of user data to function effectively. The “cold start problem” – recommending restaurants to new users with limited rating history – is a significant challenge. Popular implementations include user-based and item-based collaborative filtering, each with its own strengths and weaknesses.
Hybrid Recommendation System
A hybrid recommendation system combines content-based and collaborative filtering approaches to leverage the strengths of both. This integrated strategy can address the limitations of each individual approach. For example, content-based filtering could initially provide a baseline set of recommendations based on a user’s explicit preferences. Then, collaborative filtering could refine these recommendations by incorporating the preferences of similar users. This layered approach provides a more robust and comprehensive recommendation engine, capable of handling both new users and established users with well-defined preferences. The weighting of each filtering technique can be adjusted based on factors such as data availability and user behavior. This allows for a dynamic and adaptive system that continuously learns and improves its recommendations.
Incorporating User Reviews and Ratings
User reviews and ratings are crucial for building a robust and trustworthy restaurant recommendation system. These data points provide valuable qualitative and quantitative insights into the user experience. Numerical ratings (e.g., star ratings) can be directly incorporated into recommendation algorithms, influencing the overall score or ranking of a restaurant. Textual reviews can be analyzed using natural language processing (NLP) techniques to extract sentiment, identify key themes (e.g., food quality, service, ambiance), and categorize reviews based on positive or negative aspects. This allows the system to understand the nuances of user experiences beyond simple numerical scores. For example, a restaurant might receive high average ratings but negative reviews mentioning long wait times, which the system can then incorporate into its recommendations, prioritizing restaurants with both high ratings and positive reviews related to wait times.
Filtering Restaurant Options Based on Specific Criteria, Good dinner places near me
A step-by-step procedure for filtering restaurant options typically involves:
1. Gathering User Preferences: Obtain user input regarding cuisine type, price range, dietary restrictions (vegetarian, vegan, gluten-free, allergies), desired ambiance (casual, fine dining), and other preferences.
2. Database Query: Construct a database query that filters the restaurant database based on the specified criteria. This involves using logical operators (AND, OR) to combine multiple conditions. For example, a query might filter for Italian restaurants within a specific price range that offer vegetarian options.
3. Sorting and Ranking: Sort the filtered results based on factors such as user ratings, proximity to the user’s location, and other relevant criteria. This step is crucial for presenting the most relevant and appealing options to the user.
4. Presentation: Present the filtered and ranked results to the user in a clear and user-friendly manner, including relevant information such as restaurant name, address, ratings, and user reviews. Visual elements like images and maps can enhance the user experience.
Presenting Restaurant Information Effectively

Driving traffic to your restaurant’s online presence is only half the battle. Once users land on your site or app, you need to convert them into paying customers. This means presenting your restaurant’s information clearly, concisely, and compellingly. Think of it as your restaurant’s online storefront – it needs to be visually appealing and effortlessly informative. A poorly designed presentation can lead to lost opportunities, while a well-crafted one can significantly boost your bottom line.
Effective presentation of restaurant information hinges on understanding your target audience and tailoring the information to their needs. Are you targeting families, young professionals, or foodies? The language, imagery, and emphasis on specific features should reflect this understanding. Consider A/B testing different presentation styles to optimize your conversion rates.
Sample Restaurant Descriptions
Crafting compelling descriptions requires highlighting what makes your restaurant unique. Below are examples demonstrating how to showcase different restaurant styles:
- Fine Dining: “Experience an evening of unparalleled elegance at ‘The Gilded Lily’. Our award-winning chef crafts exquisite dishes using only the freshest, locally sourced ingredients. Indulge in our seasonally inspired tasting menu, expertly paired with an extensive wine list. Enjoy impeccable service in our sophisticated ambiance, perfect for romantic dinners or special celebrations.”
- Casual Dining: “‘The Cozy Corner Cafe’ offers a warm and inviting atmosphere, perfect for a relaxed meal with friends and family. Our menu features classic American comfort food with a modern twist, using high-quality ingredients at affordable prices. Enjoy daily specials, happy hour deals, and a family-friendly environment.”
- Fast Casual: “‘Quick Bites’ is your go-to spot for delicious, healthy, and affordable meals on the go. We offer a customizable menu with fresh salads, wraps, and bowls, prepared quickly and efficiently. Our online ordering system makes it easy to grab your meal and head out the door. Perfect for busy individuals or those seeking a quick and satisfying lunch or dinner.”
User Interface for Restaurant Information
A clean and intuitive user interface is crucial for a positive user experience. Consider using a consistent layout across all your restaurant listings, emphasizing key information like name, location, cuisine type, and customer rating. Here’s an example using HTML blockquotes to showcase different restaurant styles:
The Gilded Lily
Fine Dining | $$$$ | 4.8 stars
Award-winning cuisine, exquisite ambiance.
The Cozy Corner Cafe
Casual Dining | $$ | 4.5 stars
Comfort food with a modern twist, family-friendly.
Quick Bites
Fast Casual | $ | 4.2 stars
Healthy and customizable meals, quick service.
Using Bullet Points to Highlight Key Features
Bullet points are a highly effective way to present key information concisely and attractively. They improve readability and allow users to quickly scan and identify information relevant to their needs.
Here’s how to use bullet points to showcase a restaurant’s key features:
- Menu Highlights: * Locally sourced ingredients * Vegetarian options * Gluten-free choices * Signature dishes (e.g., “Award-winning Lobster Bisque,” “Famous BBQ Ribs”)
- Special Offers: * Happy hour specials (e.g., “Half-price appetizers from 4-6pm”) * Lunch specials * Family meal deals * Seasonal promotions (e.g., “Summer Patio Specials”)
- Hours of Operation: * Monday-Friday: 11am-9pm * Saturday: 10am-10pm * Sunday: 12pm-8pm * Note any exceptions or special holiday hours.
Visual Representation of Restaurant Data: Good Dinner Places Near Me

Data visualization is crucial for transforming raw restaurant information into actionable insights for users seeking “good dinner places near me.” Effective visuals not only present the data but also enhance understanding and decision-making, ultimately leading to higher user engagement and satisfaction. A well-designed visual representation can significantly improve the user experience by making the search for the perfect restaurant intuitive and enjoyable.
Geographic Distribution of Restaurants
A heatmap is an ideal visual representation for displaying the distribution of restaurants across a geographic area. Imagine a map of the city or region, with different shades of a color, say, red, indicating the density of restaurants. Darker shades of red would represent areas with a high concentration of restaurants, while lighter shades would show areas with fewer options. Key map elements should include prominent landmarks, major roads, and potentially neighborhood boundaries for better contextualization. Users can quickly identify restaurant clusters and areas with diverse culinary offerings at a glance. This provides an immediate spatial understanding, helping users narrow their search based on proximity and area preference.
Visual Representation of User Ratings and Reviews
User ratings can be effectively displayed using a combination of star ratings and visual progress bars. Each restaurant listing can feature a prominent star rating (e.g., 4.5 out of 5 stars) alongside a horizontal progress bar that visually represents the percentage of positive, neutral, and negative reviews. For example, a predominantly green progress bar would indicate mostly positive reviews, while a red-dominated bar would highlight negative feedback. This allows users to quickly assess the overall sentiment and gauge the quality of a restaurant based on collective user experiences. A tooltip or hover effect could reveal the number of reviews behind each rating and percentage, adding further detail on demand.
Visual Communication of Price Range and Cuisine Type
A scatter plot is a suitable choice for visually representing the price range and cuisine type of restaurants. The x-axis could represent the price range (categorized as $, $$, $$$, $$$$), and the y-axis could represent different cuisine types (e.g., Italian, Mexican, Indian, American). Each restaurant would be represented by a data point on the plot, with color-coding used to distinguish cuisine types. For instance, Italian restaurants could be represented by green dots, Mexican by red, and so on. This allows users to quickly identify restaurants that fit both their budget and culinary preferences. Users can visually compare restaurants across both dimensions, facilitating a more informed choice. Adding interactive elements, such as the ability to filter by price range or cuisine, would further enhance user experience and search efficiency.