Understanding User Intent
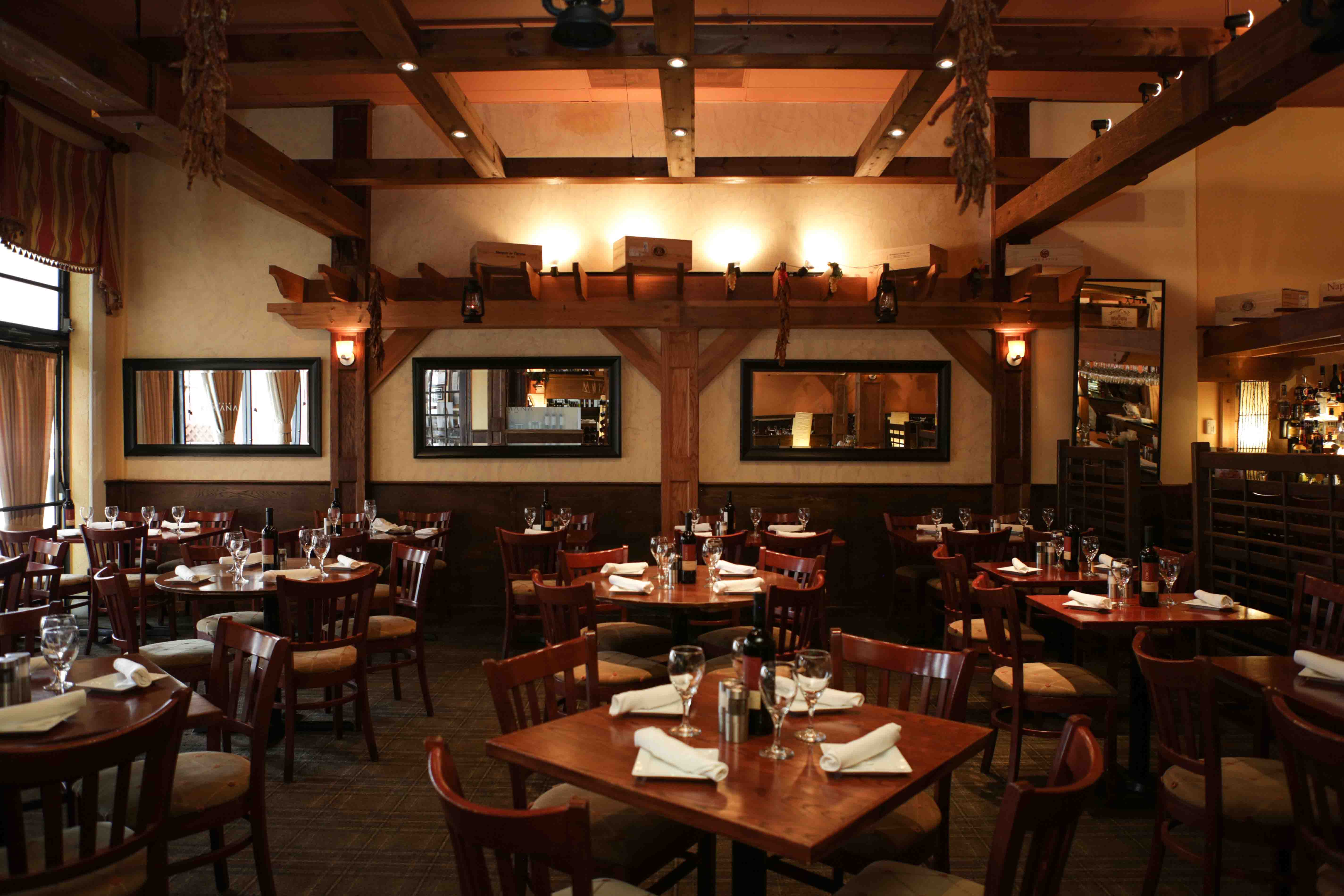
The seemingly simple query, “nice place for lunch near me,” reveals a surprising depth of user intent. It’s not just about finding *a* restaurant; it’s about finding the *right* restaurant for a specific need and occasion. Understanding this nuance is crucial for businesses aiming to capture this valuable local search traffic.
The factors driving a user’s choice are multifaceted and intertwined. A simple search hides a complex decision-making process influenced by a variety of personalized preferences. We need to unpack these layers to effectively target potential customers.
Types of Lunch Experiences
The phrase “nice place for lunch” can encompass a wide spectrum of dining experiences. It could imply a quick, casual bite, a leisurely business lunch, a romantic date, or a celebratory meal with friends. Each scenario demands a different type of establishment, from a fast-casual eatery to a fine-dining restaurant. The user’s unspoken expectations are shaped by the context of their lunch. A quick lunch during a busy workday will prioritize speed and convenience, while a celebratory lunch might prioritize ambiance and quality.
Factors Influencing Lunch Location Choice
Several key factors heavily influence a user’s decision. Price range is paramount; some users are looking for budget-friendly options, while others are willing to spend more for a premium experience. Cuisine type is another crucial factor, with preferences ranging from familiar comfort food to exotic international cuisine. The ambiance of the restaurant is also important; some users prefer a bustling, lively atmosphere, while others seek a quiet, intimate setting. Finally, location, implied by “near me,” plays a significant role, prioritizing convenience and proximity.
Geographical Limitations of “Near Me”
The term “near me” is inherently subjective and context-dependent. Its interpretation is heavily influenced by the user’s current location and their personal definition of “near.” For a user in a densely populated urban area, “near me” might encompass a radius of several miles. Conversely, in a rural area, it might be limited to a much smaller radius. The accuracy of the “near me” search relies on the device’s location services and the user’s understanding of their surroundings. This ambiguity necessitates a robust location-based search algorithm to accurately interpret and fulfill the user’s request.
Examples of Lunch Location Preferences
The following table illustrates the diverse range of lunch preferences implied by the search query. Note that these are examples and actual options will vary based on location.
Cuisine Type | Price Range | Ambiance | Example Location |
---|---|---|---|
Italian | $$ | Casual | Neighborhood Trattoria |
Mexican | $ | Lively | Popular Taco Joint |
Seafood | $$$ | Upscale | Oceanfront Restaurant |
American | $$ | Relaxed | Gastropub |
Location-Based Search
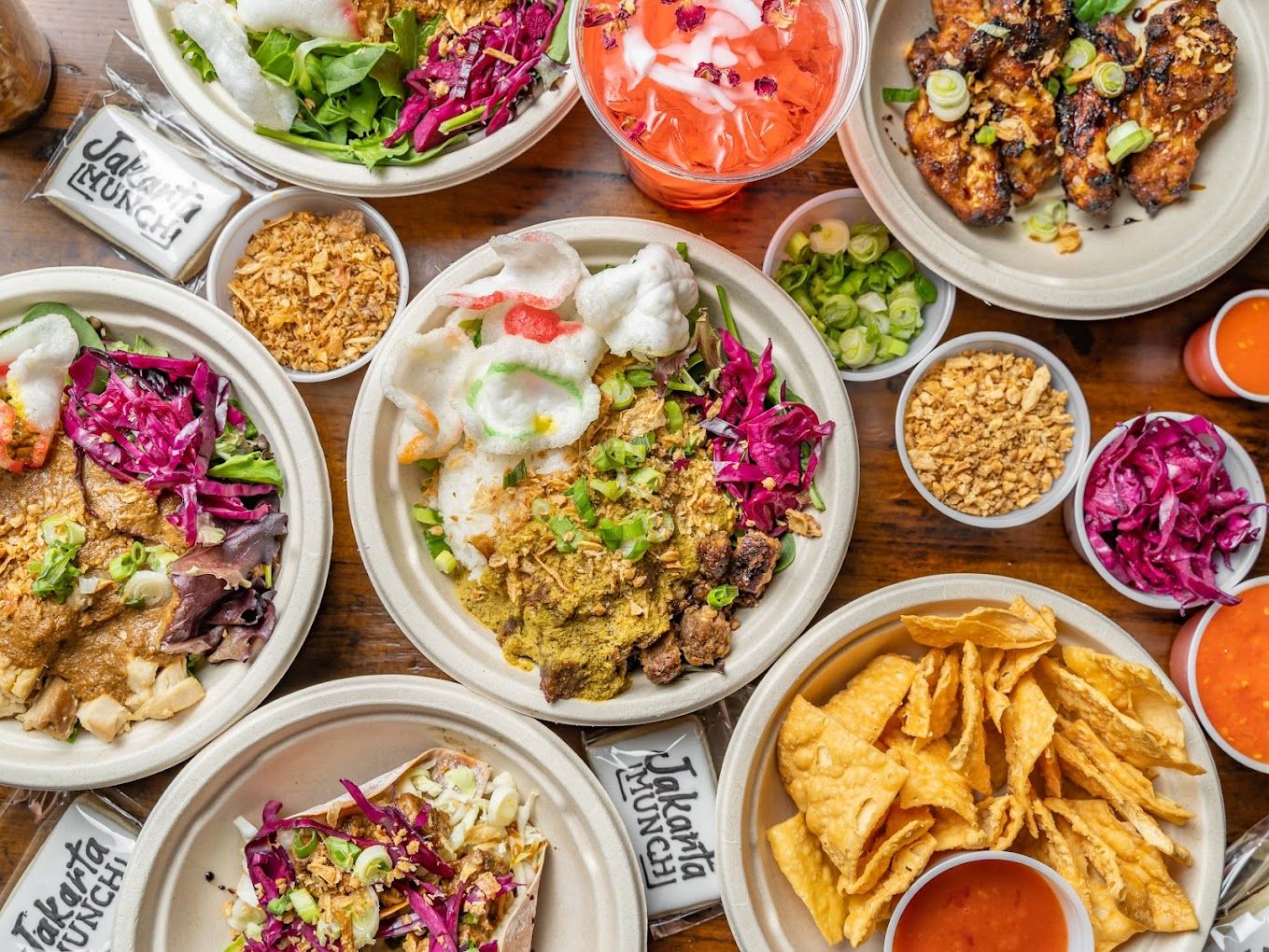
In today’s hyper-connected world, location data is no longer a mere add-on; it’s the cornerstone of a truly effective search experience. For a query like “nice place for lunch near me,” location is paramount. Without it, the search engine is essentially blind, unable to deliver relevant results that truly meet the user’s immediate needs. Understanding this fundamental dependency is critical to building a superior search system.
Location data directly impacts the relevance of search results. The power of location-based search lies in its ability to filter and prioritize results based on proximity. Imagine searching for a restaurant without specifying your location; you’d be overwhelmed by irrelevant results spanning the entire globe. Conversely, a location-aware search instantly narrows down the options to establishments within a reasonable distance, making the search far more efficient and user-friendly.
Proximity and User Location Influence on Search Results
The influence of proximity is governed by a complex algorithm that weighs various factors. The most obvious is the straight-line distance between the user’s location and the potential result. However, this is often refined by considering factors like traffic conditions (longer travel time might downgrade a closer option), user preferences (e.g., a preference for walking distance over driving), and even opening hours (a restaurant that’s very close but closed is less useful). This prioritization ensures that the most relevant and accessible options appear at the top of the search results. For instance, Google Maps considers these factors when ranking restaurants, prioritizing those that are both geographically close and operationally accessible at the time of the search.
Designing a Location-Based Search Result Prioritization System
A robust location-based search prioritization system requires a multi-faceted approach. It starts with accurately determining the user’s location, which can be obtained through GPS, IP address, or manual input. Next, a distance calculation algorithm is applied – typically using the Haversine formula for accurate great-circle distances. This distance is then weighted against user preferences, which might be explicitly stated (e.g., “within 1 mile”) or implicitly inferred from past behavior (e.g., frequent searches for restaurants in a specific neighborhood). Finally, a scoring system combines distance, user preferences, and other relevant factors (like ratings, reviews, and opening hours) to rank the results. A simple example could be: Score = (1/distance) * (preference weight) * (rating). This system prioritizes closer, preferred, and highly-rated options.
Representing Location Data
Several methods exist for representing location data, each with its strengths and weaknesses. Geographical coordinates (latitude and longitude) provide a precise, universally understood representation. Addresses, while less precise (due to variations in address formats and building layouts), are more user-friendly and easily understood. Postal codes offer a coarser level of granularity, useful for broader regional searches. Choosing the right representation depends on the specific application and the level of precision required. For example, a ride-sharing app relies heavily on precise coordinates for accurate pick-up and drop-off, while a search for nearby cafes might use postal codes or addresses for a less precise, but still useful, location filter.
Restaurant Attributes & Filtering: Nice Place For Lunch Near Me
Finding the perfect lunch spot shouldn’t feel like navigating a labyrinth. A streamlined search experience hinges on the ability to filter and refine results based on crucial restaurant attributes. This allows users to quickly zero in on places that precisely meet their needs and preferences, ultimately leading to higher user satisfaction and increased conversions, whether that’s a click-through to a restaurant’s website or a reservation made directly through your platform.
Effective filtering requires a strategic approach to categorizing and presenting restaurant information. By organizing data intelligently, you empower users to make informed decisions, minimizing the time spent sifting through irrelevant options. This translates to a more efficient and enjoyable user experience, crucial for any business aiming to maximize engagement.
Restaurant Attribute Categories
To create a truly effective filtering system, we need to categorize restaurant attributes logically. This allows users to easily find what they’re looking for, without being overwhelmed by too many options. Consider these categories:
- Essentials: This includes the restaurant’s name, address, phone number, and hours of operation. This fundamental information is crucial for any search result and should always be prominently displayed.
- User Reviews & Ratings: Aggregate star ratings (e.g., from Google, Yelp, etc.) provide a quick visual indicator of overall satisfaction. Displaying a summary of recent reviews (positive and negative) allows users to gauge the overall dining experience.
- Cuisine & Dietary Options: Allow users to filter by specific cuisines (e.g., Italian, Mexican, Thai) and dietary restrictions (e.g., vegetarian, vegan, gluten-free). Clearly indicating the availability of these options is essential for a large segment of diners.
- Price Range: Offer price filtering options (e.g., $, $$, $$$) to help users quickly identify restaurants within their budget. This is a critical factor for many diners.
- Amenities & Atmosphere: Include filters for amenities like outdoor seating, Wi-Fi, parking, and kid-friendliness. Allow users to filter by atmosphere (e.g., casual, fine dining, romantic). This adds a layer of personalization to the search.
- Visuals: High-quality photos of the restaurant’s exterior, interior, and food are crucial. These visuals help users assess the ambiance and quality of the food before making a decision. Consider showcasing a slideshow or gallery of images.
Filter Impact on Search Results
The way users interact with filters directly shapes the search results. Let’s look at how different filter combinations might affect the results:
- Filtering by Price: Selecting a “$” price range will significantly narrow the results to budget-friendly options, excluding more expensive restaurants. Similarly, choosing “$$$+” will only show high-end establishments.
- Filtering by Cuisine: Choosing “Italian” will eliminate all restaurants that don’t serve Italian food. Combining this with a price filter (e.g., “Italian” and “$”) will further refine the results to affordable Italian restaurants.
- Filtering by Rating: Selecting a high rating (e.g., 4 stars and above) will only show highly-rated restaurants, potentially missing out on some hidden gems with lower ratings but potentially excellent food.
- Combined Filtering: Combining multiple filters (e.g., “Mexican,” “$$,” “outdoor seating”) will significantly reduce the number of results, providing a highly targeted selection based on specific preferences. This precision is key to a satisfying user experience.
Visual Presentation of Results
The visual presentation of search results is paramount in a user’s experience. A poorly designed interface, regardless of how accurate the underlying data is, will drive users away. Think of it like this: you’ve got the best restaurant recommendations in the world, but if nobody can find them easily, your service is useless. The key is to create a visually appealing and intuitive layout that prioritizes information clarity and user engagement. This is where you convert casual browsers into loyal customers.
The ideal layout emphasizes speed and efficiency. Users shouldn’t have to hunt for what they need. We need to present the information in a digestible and easily scannable format. This involves a strategic blend of text, images, and design elements working in harmony.
Restaurant Result Card Design
Each restaurant result should be presented as a visually compelling “card.” This card should be easily distinguishable from others, perhaps with a subtle shadow or border. Consider a clean, modern design using ample white space to avoid overwhelming the user. The card should be designed to be easily scannable at a glance.
The Cozy Corner Bistro
French, Bistro
123 Main Street, Anytown
$$
The `restaurant-image` div contains a high-quality image of the restaurant’s interior, perhaps showcasing a bustling atmosphere or a beautifully decorated dining area. The `image-overlay` subtly displays the restaurant’s star rating, drawing the eye immediately to a key metric. The `restaurant-info` div then neatly presents the name, cuisine type, address, and price range. This layout is clean, concise, and instantly provides the user with the most relevant information.
Image Selection and Presentation
High-quality images are crucial. Think professional photography, not blurry snapshots. The images should accurately represent the restaurant’s ambiance and food quality. Images of the restaurant’s interior should evoke a sense of the atmosphere: a dimly lit, romantic setting might be shown with warm lighting and cozy furniture, while a vibrant, family-friendly restaurant could be shown with bright lighting and happy customers.
For food images, close-ups that showcase the textures, colors, and appetizing qualities of the dishes are essential. A perfectly plated dish, with steam rising or sauce glistening, is far more appealing than a generic, poorly lit photograph. Descriptive alt text is also critical for accessibility and . For example, instead of “food,” the alt text might read: “Close-up of a succulent grilled salmon fillet with roasted vegetables and lemon wedges.” This adds another layer of information, improving the user experience.
Sensory Detail in Image Descriptions
Images should appeal to more than just the visual sense. Descriptive text accompanying the images should evoke the other senses. For example, describing a restaurant’s ambiance might include phrases like: “The aroma of freshly baked bread wafts through the air,” or “The gentle hum of conversation creates a lively yet intimate atmosphere.” Describing a dish could include details like: “The rich, creamy texture of the pasta contrasts beautifully with the crisp, salty bacon,” or “The sweet and spicy aroma of the Thai curry fills the air.” This multi-sensory approach creates a more immersive and engaging experience for the user.
Handling Ambiguity and Variations
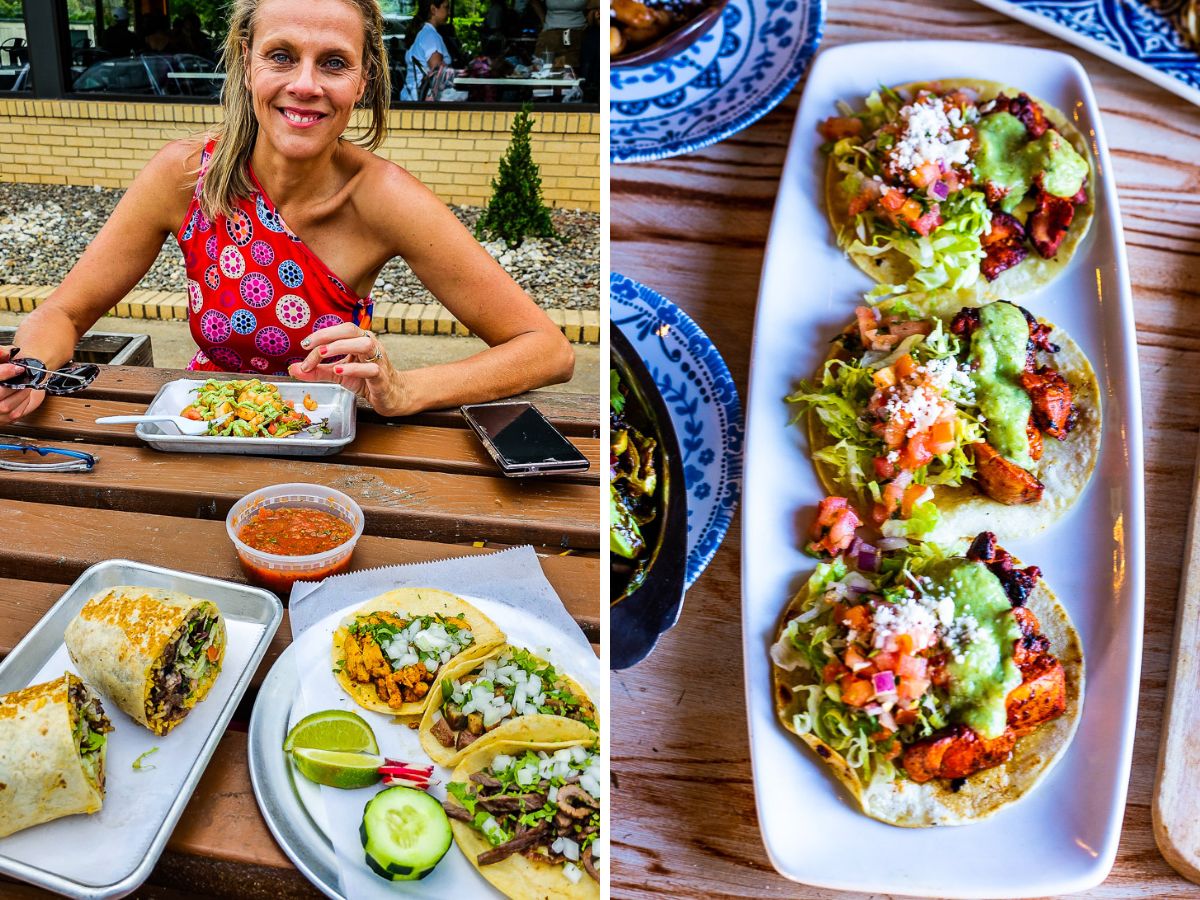
User queries, especially in the context of finding a nearby lunch spot, are rarely perfectly precise. They’re rife with variations in spelling, phrasing, and implied meaning. Successfully navigating these ambiguities is crucial for delivering a relevant and satisfying user experience. Ignoring these variations can lead to lost opportunities and frustrated users. A robust system must be able to understand the user’s intent even when the query is less than ideal.
The core challenge lies in bridging the gap between the user’s imprecise language and the structured data representing restaurants. This requires a multi-pronged approach that combines sophisticated natural language processing (NLP) techniques with carefully designed data structures and algorithms. The goal is not just to find *a* result, but the *most relevant* result, based on a nuanced understanding of what the user truly wants.
Spelling Error Correction and Synonym Handling
Accurate interpretation of user input requires robust mechanisms for handling spelling mistakes and synonyms. For instance, a user might type “itallian resturant” instead of “Italian restaurant.” Leveraging techniques like Levenshtein distance calculations can identify and correct common spelling errors. Similarly, a thesaurus or synonym database can map variations in wording (“lunch spot,” “eatery,” “restaurant”) to their common semantic core. These techniques work best when integrated with a system that considers context. For example, “cheap eats” should be handled differently than “cheap seats,” even though they share a common word. The algorithm needs to weigh context clues to arrive at the most likely interpretation. Consider a scenario where a user searches for “Mexican food near me.” The system should be able to handle variations like “Mex food,” “Mexican cuisine,” or even “tacos near me,” all pointing towards the same basic intent.
Ambiguity Resolution Techniques
Ambiguity arises when a query can have multiple valid interpretations. For example, “pizza” could refer to a specific restaurant named “Pizza Place,” a type of cuisine, or even a dish. To resolve this, the system needs to utilize contextual clues, such as the user’s location and past search history. If the user is near a restaurant called “Pizza Place,” that should rank higher. If their past searches have focused on Italian food, then the “pizza” query is more likely to refer to a restaurant serving that cuisine. Another common ambiguity involves proximity. “Near me” is inherently vague; a sophisticated system would employ geolocation data to determine a reasonable radius, perhaps offering the user the option to adjust this radius for more granular control. Incorporating user preferences, if available (e.g., preferred cuisine, price range), further enhances the accuracy of ambiguity resolution.
Suggesting Alternative Search Terms or Refinements, Nice place for lunch near me
When the system detects a potentially ambiguous or poorly-formed query, offering alternative search terms or refinements can greatly improve the user experience. This could involve suggesting similar terms (e.g., suggesting “Italian restaurant” for “itallian resturant”) or offering filters to narrow down the results (e.g., suggesting price range or cuisine filters). For instance, if a user searches for simply “lunch,” the system might suggest adding a cuisine type (“lunch, Italian”) or a location qualifier (“lunch, downtown”). These suggestions should be context-aware and tailored to the specific query, increasing the likelihood of a successful search. This proactive approach helps guide users towards more precise queries and ultimately more satisfying results.