Understanding User Intent Behind “Highest Rated Restaurants Near Me”
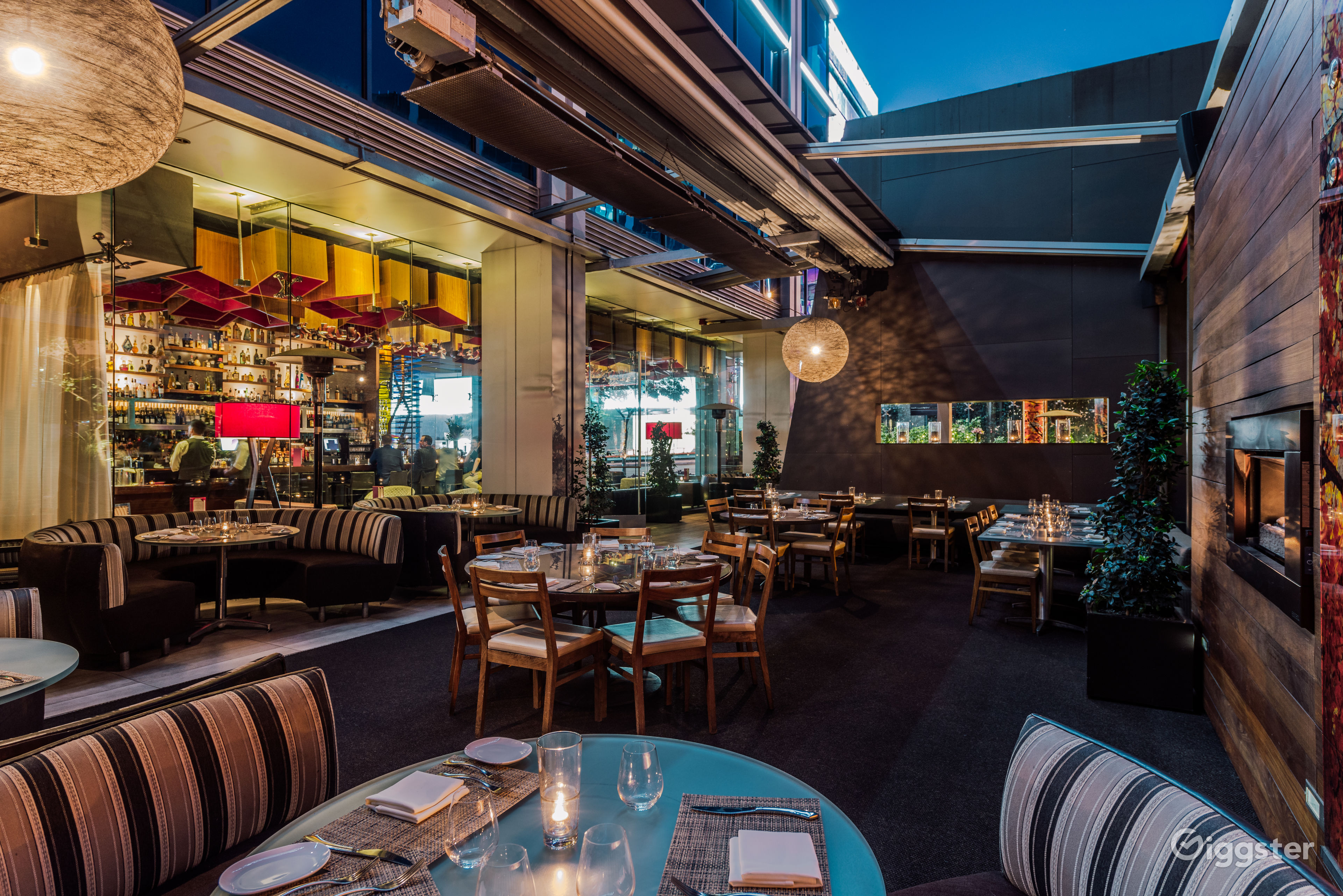
The search query “highest rated restaurants near me” reveals a user’s immediate need for a dining recommendation, prioritizing quality and convenience. This seemingly simple phrase, however, masks a diverse range of underlying motivations and expectations. Understanding these nuances is crucial for businesses aiming to capture this valuable traffic.
The primary user need is straightforward: to discover highly-regarded restaurants in their current vicinity. This implies a desire for a positive dining experience, driven by factors ranging from a craving for a specific type of food to a celebratory occasion. The convenience aspect is paramount; users aren’t looking to travel extensively for a meal.
User Segmentation
Different user types employ this search query with varying expectations. Tourists, for instance, often lack local knowledge and rely heavily on online reviews to navigate unfamiliar culinary landscapes. They might prioritize highly-rated options for a memorable experience, less concerned about price within a reasonable range. Locals, conversely, might be searching for a new culinary adventure or a reliable go-to spot for a specific occasion. Budget-conscious diners will be particularly attuned to price-performance ratios, seeking highly-rated restaurants that align with their spending limits. Conversely, luxury seekers will prioritize ambiance, exclusivity, and exceptional service, often accepting higher prices for the elevated experience.
Implicit Expectations Regarding Restaurant Attributes
The search query inherently implies certain expectations about the restaurant’s characteristics. High ratings suggest a combination of factors including quality of food, service levels, and overall atmosphere. Users implicitly anticipate accurate and up-to-date information on menus, pricing, and operating hours. Cuisine type is often a significant factor; the search might be refined by implicit context like “highest rated Italian restaurants near me,” showcasing a specific culinary preference. Price range is another implicit expectation; the user’s decision to search for “highest rated” rather than “cheap restaurants” indicates a willingness to pay a fair price for quality, although the exact price point varies greatly across user segments. Ambiance plays a crucial role, especially for special occasions or romantic dinners. A high rating often implies a pleasing atmosphere, appropriate for the intended dining experience.
Typical User Persona
Let’s create a persona to represent a typical searcher: Meet Sarah, a 32-year-old marketing professional. She’s visiting a new city for a business conference and wants to celebrate a successful presentation with colleagues. She’s open to various cuisines but prefers a sophisticated ambiance and doesn’t mind spending a bit more for a high-quality dining experience. Sarah relies heavily on online reviews and ratings to make informed decisions, especially in unfamiliar environments. Her search for “highest rated restaurants near me” reflects her desire for a memorable and reliable dining experience, balancing quality, location, and atmosphere. This persona highlights the blend of convenience, quality, and experience that drives this specific search query.
Data Sources for Restaurant Ratings and Reviews
Finding the best restaurants near you often hinges on reliable ratings and reviews. But not all data sources are created equal. Understanding the strengths and weaknesses of different platforms is crucial for making informed dining decisions. This analysis dives into the key players in the restaurant review landscape, examining their data collection methods, user bases, and overall reliability.
Comparison of Restaurant Review Platforms
Choosing the right platform for restaurant reviews depends on your priorities. Different sources cater to different demographics and have varying strengths in terms of accuracy and breadth of coverage. Consider these factors when evaluating restaurant ratings.
Name | Data Type | Reliability | Coverage Area |
---|---|---|---|
Yelp | User-generated reviews, ratings, photos, and business information. | Generally high, but susceptible to bias and fake reviews. Yelp actively combats fake reviews, but some slip through. | Extensive, particularly strong in the US and Canada. |
Google Maps | User-generated reviews, ratings, photos, business information, and integration with Google services. | High reliability due to Google’s scale and review filtering mechanisms. However, the sheer volume of reviews can dilute the signal. | Global coverage, particularly strong in areas with high internet penetration. |
TripAdvisor | User-generated reviews, ratings, photos, and travel-related information. Focuses on travel experiences, including restaurants. | High reliability due to a large user base and robust moderation processes. Stronger for tourist destinations and higher-end establishments. | Global coverage, with a stronger presence in popular tourist destinations. |
Zomato | User-generated reviews, ratings, photos, menus, and restaurant information. Stronger presence in Asia and other emerging markets. | Reliability varies geographically. Generally high in regions with a large user base, but may be less reliable in areas with less active users. | Global coverage, with a particularly strong presence in India, the Middle East, and parts of Asia. |
Data Discrepancies Across Platforms
It’s not uncommon to see significant differences in ratings and reviews for the same restaurant across different platforms. For example, a restaurant might receive overwhelmingly positive reviews on Yelp but mixed reviews on TripAdvisor. This discrepancy could be due to several factors, including:
* User Demographics: Each platform attracts a different user base. Yelp users, for instance, tend to be younger and more tech-savvy, while TripAdvisor users may be older and more focused on travel experiences. This can lead to different perspectives and rating patterns.
* Review Filtering and Moderation: Different platforms have different algorithms and policies for filtering fake reviews and managing user-generated content. This can significantly impact the overall rating and the types of reviews that are visible.
* Restaurant Focus: Some platforms might cater more to specific types of restaurants. For example, TripAdvisor might have a stronger focus on fine dining establishments, while Yelp might have more reviews for casual eateries.
A restaurant might receive a 4.5-star rating on Google Maps, a 4-star rating on Yelp, and a 3.5-star rating on TripAdvisor. These differences highlight the importance of consulting multiple sources before making a dining decision.
Factors Influencing Restaurant Ratings: Highest Rated Restaurants Near Me

Online restaurant ratings are the lifeblood of modern dining. They dictate success, influence decisions, and ultimately shape a restaurant’s destiny. Understanding the factors that contribute to these ratings is crucial, not only for restaurant owners striving for five-star reviews but also for diners seeking the perfect culinary experience. This analysis delves into the key components that drive online restaurant ratings, examining their relative importance and the potential for bias.
Highest rated restaurants near me – A restaurant’s overall rating is a complex interplay of several critical factors. While individual preferences naturally influence perceptions, some elements consistently impact the aggregated scores found on platforms like Yelp, Google Reviews, and TripAdvisor. These factors aren’t always equally weighted; their significance varies depending on the diner’s priorities and expectations.
Food Quality and Taste
Unsurprisingly, the quality and taste of the food are paramount. This encompasses everything from the freshness of ingredients to the skill of the chef in preparing and presenting the dishes. A consistently delicious meal, prepared with care and attention to detail, is the foundation of any high-rated restaurant. Negative reviews often highlight issues such as bland food, undercooked items, or poor ingredient quality. For example, a Michelin-starred restaurant will be judged far more harshly on subtle nuances of flavor than a casual burger joint.
Service Quality and Attentiveness
Exceptional service can elevate a dining experience, while poor service can easily ruin it. This includes factors like attentiveness of staff, speed of service, friendliness and helpfulness of servers, and overall professionalism. A quick, efficient, and friendly interaction with the waitstaff significantly contributes to a positive review. Conversely, slow service, inattentive servers, or rude staff are frequently cited as reasons for lower ratings. Think of the difference between a bustling, yet smoothly-run establishment versus one where you’re constantly chasing down your server.
Ambiance and Atmosphere, Highest rated restaurants near me
The restaurant’s ambiance plays a significant role in the overall dining experience. This encompasses the decor, lighting, music, cleanliness, and overall feel of the establishment. A comfortable, inviting atmosphere encourages diners to relax and enjoy their meal, while a cramped, noisy, or dirty environment can detract from the experience. For example, a romantic dinner requires a different ambiance than a family-friendly restaurant, and these different expectations will influence the ratings.
Price and Value for Money
The price of the meal relative to its quality and quantity significantly influences customer perception. Diners expect a certain level of quality for the price they pay. A high-priced restaurant with subpar food will receive overwhelmingly negative reviews, while a reasonably priced restaurant offering good value will likely garner positive feedback. This is a crucial factor, especially in a competitive market where diners have many options.
Cleanliness and Hygiene
Cleanliness and hygiene are non-negotiable for most diners. A clean and well-maintained restaurant inspires confidence and trust, while a dirty or unhygienic establishment can be a major turn-off. This includes the cleanliness of the dining area, restrooms, and kitchen. A single negative review mentioning uncleanliness can severely impact a restaurant’s overall rating, as hygiene is a critical factor influencing customer trust and safety.
Impact of Biased Reviews
Online reviews are not always objective. Personal biases, past experiences, and even ulterior motives can influence the ratings and comments left by diners. A single negative review, even if unjustified, can significantly impact a restaurant’s overall rating. Conversely, a surge of positive reviews, potentially orchestrated or incentivized, can artificially inflate a restaurant’s score. Therefore, it’s crucial to consider the context and credibility of individual reviews when evaluating a restaurant’s overall rating.
Relative Importance of Factors Across User Segments
The relative importance of these factors can vary greatly depending on the diner’s demographics, preferences, and dining habits. For example, a young professional might prioritize ambiance and a lively atmosphere, while a family with young children might prioritize cleanliness and kid-friendly options. Similarly, a budget-conscious diner will likely weigh price more heavily than a luxury-seeking patron.
Factor | Relative Importance (Percentage) |
---|---|
Food Quality | 40% |
Service Quality | 25% |
Ambiance | 15% |
Price/Value | 10% |
Cleanliness | 10% |
Visualizing Restaurant Data
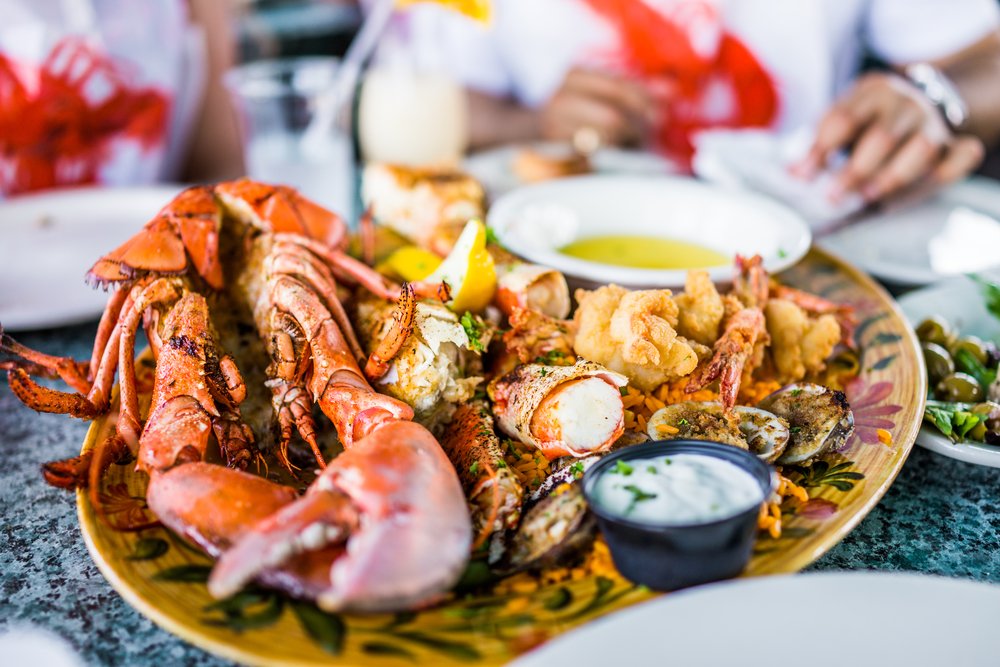
Data visualization is crucial for making sense of the vast amount of restaurant information available online. By effectively presenting ratings, reviews, and other key data points, you can create a compelling user experience and help people quickly identify the perfect dining spot. This involves leveraging maps, charts, and other visual tools to communicate complex information in an intuitive and engaging manner.
Mapping Restaurant Ratings and Reviews
A map visualization provides an excellent way to showcase restaurant data geographically. Each restaurant can be represented as a marker on the map, with the size or color of the marker reflecting its average rating. Larger, brighter markers could indicate higher-rated restaurants, immediately drawing the user’s attention. Hovering over a marker could then display a pop-up window containing additional details such as the restaurant’s name, address, cuisine type, price range, and a summary of user reviews. This interactive approach allows users to quickly scan the map for restaurants in their desired area and then drill down for more information. For example, a map of New York City could show Michelin-starred restaurants as large, gold markers, while casual eateries might be smaller, blue markers.
Incorporating Additional Information into Map Visualizations
Beyond basic ratings, incorporating additional data enhances the map’s utility. Cuisine type can be represented through color-coding (e.g., Italian restaurants in green, Mexican in red). Price range can be indicated by different marker shapes (e.g., circles for budget-friendly, squares for mid-range, triangles for upscale). User ratings can be displayed as a numerical score or a star rating within the marker pop-up. Consider also incorporating user-generated photos directly onto the map markers for a richer visual experience. Imagine a map of San Francisco where each marker not only shows the rating and cuisine but also displays a thumbnail image of the restaurant’s most popular dish.
Visual Representation of Restaurant Rating Distribution
Understanding the distribution of restaurant ratings provides valuable insights. A histogram is an effective way to visualize this. The x-axis represents the rating range (e.g., 1-5 stars), and the y-axis represents the number of restaurants falling within each rating range. A tall bar at the 4-5 star range would indicate a concentration of highly-rated establishments. Alternatively, a box plot displays the median, quartiles, and outliers of the rating distribution, providing a concise summary of the data’s central tendency and spread. For instance, a box plot could clearly show if most restaurants cluster around a 3.5-star average or if there’s a significant skew towards higher or lower ratings.
Hypothetical Map Visualization Description
Imagine a map of downtown Chicago. Restaurants are represented by circular markers. The size of the marker is proportional to the average rating (larger circle = higher rating). Color-coding denotes cuisine: Italian restaurants are green, Mexican are red, and American are blue. A legend in the map’s corner clearly explains the color and size scheme. Hovering over a green, large circle representing a highly-rated Italian restaurant reveals a pop-up window displaying its name, address, price range (indicated by a dollar sign icon), average rating (4.8 stars), and a brief summary of user reviews praising its authentic pasta dishes. This clear and intuitive visualization enables users to quickly identify high-quality restaurants matching their preferences and location.
Analyzing Location-Based Search Results
Understanding how location impacts your “highest rated restaurants near me” search is crucial for maximizing its effectiveness. The algorithms behind these searches are sophisticated, balancing proximity with user ratings and other factors to deliver the most relevant results. Ignoring this interplay means missing out on potentially amazing dining experiences just because they weren’t listed first.
Proximity’s Influence on Search Results
The most immediate factor influencing your search results is, unsurprisingly, your location. Search engines utilize your IP address, GPS data (if enabled), or your manually entered location to define a geographical center. Results are then ranked primarily by distance, with the closest highly-rated establishments appearing at the top. This is a fundamental aspect of location-based services (LBS), prioritizing convenience and minimizing travel time. The radius of this search can vary, often expanding as you scroll down the results page, revealing more options further away. Think of it as concentric circles radiating from your location, with the highest-rated restaurants within each ring progressively revealed.
Search Engine Algorithms and Location-Based Ranking
Search engines use complex algorithms to rank restaurants based on both location and rating. These algorithms are proprietary and not publicly available in their entirety, but we can infer key components. A simplified model might involve a weighted average combining distance and rating score. For example, a restaurant with a 4.8-star rating 1 mile away might rank higher than a 5-star restaurant 10 miles away, assuming the weight given to proximity is significant. Other factors, such as user reviews’ recency, the restaurant’s online presence (website, social media engagement), and even the time of day (lunch vs. dinner demand), can also contribute to the final ranking. This means that the precise algorithm is dynamic and adapts to a multitude of variables. Google’s algorithm, for instance, is famously complex and constantly evolving, making precise modeling difficult but fundamentally reliant on user experience optimization.
Impact of Additional Search Criteria
Adding criteria like “Italian” to your search query significantly alters the results. The search engine now filters the initial pool of nearby restaurants, retaining only those that match the “Italian” cuisine type. The ranking algorithm then re-evaluates the remaining restaurants based on their ratings and proximity to your location. A highly-rated Italian restaurant far away might be lower in the rankings than a moderately-rated Italian restaurant nearby, showcasing the continuing interplay between rating and proximity, even with specific criteria. This demonstrates how specifying your preferences refines the search and can unearth hidden gems that might have been overshadowed in a broader search. Imagine searching for “highest-rated vegan restaurants near me” – the results would drastically differ from a simple “highest-rated restaurants near me” search.
Potential Biases in Location-Based Search Results
Several biases can affect location-based search results. Understanding these biases is critical for interpreting the results effectively and not misinterpreting the rankings as absolute indicators of quality.
- Algorithmic Bias: The algorithms themselves might unintentionally favor certain types of restaurants or locations. For example, if the algorithm prioritizes restaurants with a large number of recent reviews, newer establishments might be favored over older, equally good ones with fewer reviews. This is a common issue with many machine learning algorithms.
- Data Bias: The data used to train and inform the algorithm, such as user reviews, might be skewed. For example, a restaurant might receive disproportionately negative reviews due to a temporary staffing issue, unfairly impacting its long-term ranking. Similarly, areas with higher smartphone penetration might have more reviews, giving them an advantage over less digitally connected areas.
- Geographical Bias: The algorithm might inadvertently favor restaurants in densely populated areas with more data points. A highly-rated restaurant in a sparsely populated area might be overlooked compared to a similarly rated restaurant in a city center, simply due to the volume of data available.
- Review Manipulation: Fake reviews or review manipulation can artificially inflate or deflate a restaurant’s rating, significantly influencing its ranking in search results. This is a serious problem that search engines actively combat but can still affect results.