Understanding User Intent Behind “Places to Eat Near Us”
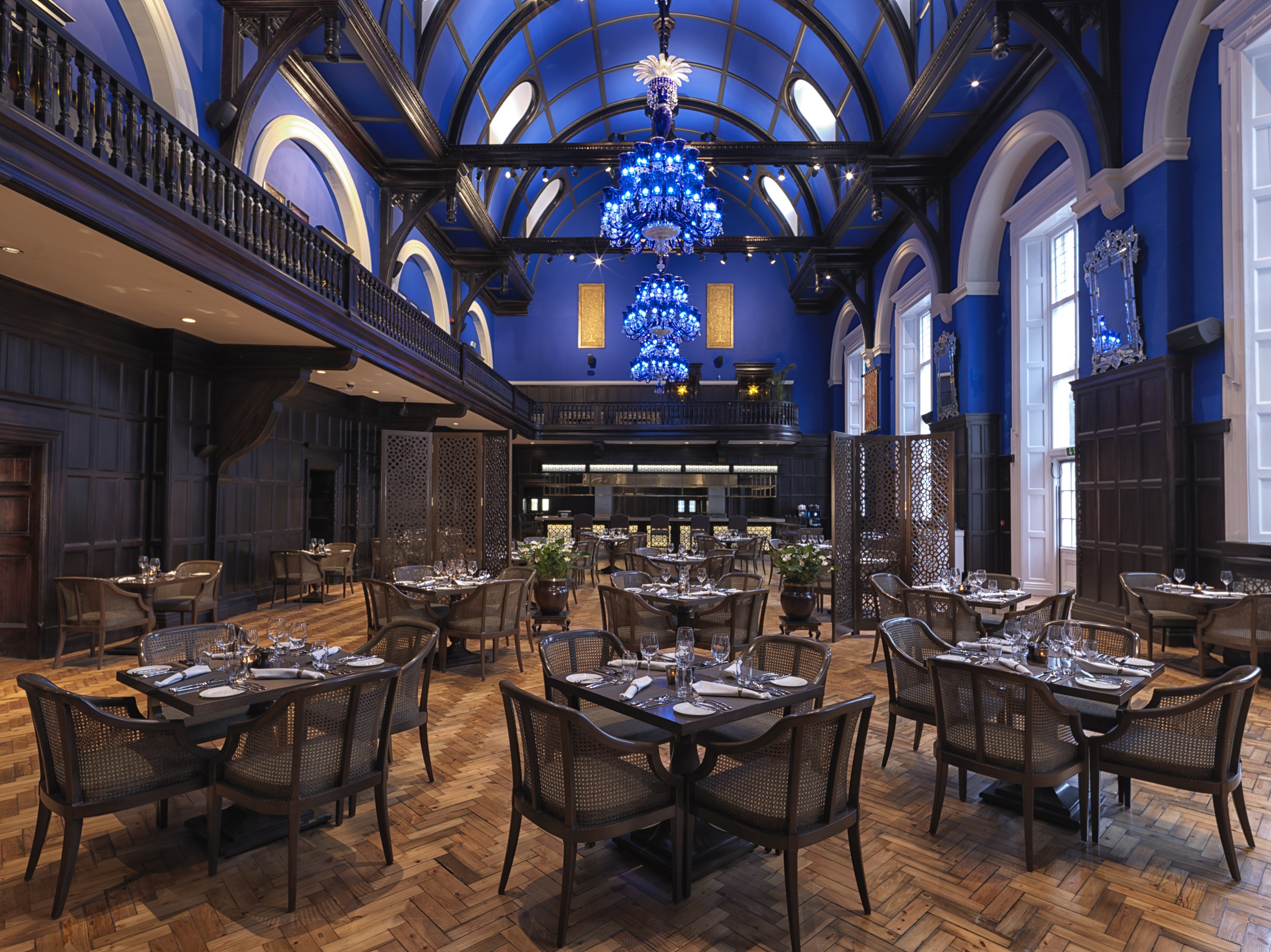
The seemingly simple search phrase “places to eat near us” reveals a surprising complexity of user intent. Understanding this nuance is crucial for businesses looking to attract customers through online search and optimize their online presence. Different users have varying needs and motivations, leading to a wide range of expectations when searching for a place to eat.
The search query’s success hinges on accurately interpreting the user’s underlying needs and desires. By understanding these diverse motivations, businesses can tailor their online profiles and marketing strategies to better target their ideal customer base, ultimately driving more traffic and revenue.
User Segmentation Based on Search Intent, Places to eat near us
Users searching for “places to eat near us” fall into several distinct segments, each with unique needs and preferences. Failing to recognize these differences can lead to missed opportunities and ineffective marketing.
- Tourists: Often looking for authentic local experiences, tourists prioritize reviews, location convenience, and potentially unique culinary offerings. Their budget can vary widely, from budget-friendly street food to upscale dining experiences.
- Locals: Locals tend to have established preferences, seeking familiar favorites, new discoveries, or specific cuisines. Their choices are frequently influenced by price, convenience, and personal recommendations. They may be searching for a quick lunch, a casual dinner, or a special occasion restaurant.
- Business Travelers: Business travelers often prioritize convenience and efficiency. Their needs frequently include quick service, reliable Wi-Fi, and perhaps a business-friendly atmosphere. They may be looking for a quick lunch meeting or a more formal dinner setting.
User Needs and Priorities
The specific needs of each user segment significantly impact their restaurant selection. Understanding these variations is critical for effective targeting.
- Quick Lunch: Users needing a fast and efficient meal often prioritize speed of service, affordability, and convenient location. Fast-casual restaurants, cafes, and sandwich shops often meet this demand.
- Fine Dining: Users seeking a special occasion or luxurious experience prioritize ambiance, high-quality food, and exceptional service. These users are less concerned with price and more focused on the overall experience.
- Budget-Friendly Options: Price is a primary concern for budget-conscious users. They may seek affordable chains, ethnic eateries, or lunch specials to maximize value.
Factors Influencing Restaurant Choice
Numerous factors beyond simple location influence the final restaurant selection. These considerations are often intertwined and vary significantly based on the user’s segment and individual preferences.
- Cuisine Type: Users often have specific culinary preferences, ranging from Italian and Mexican to Thai and Indian. Understanding these preferences is key for effective marketing and search optimization.
- Price Range: Budget significantly impacts restaurant selection. Users will often filter search results based on price, indicating a clear preference for affordability or luxury.
- Reviews and Ratings: Online reviews play a critical role in shaping user perceptions and influencing their final decision. Positive reviews build trust and credibility, driving traffic to highly-rated establishments.
- Ambiance and Atmosphere: The overall atmosphere of a restaurant significantly impacts the dining experience. Users seek environments that align with their mood and the occasion, whether it’s a romantic dinner, a family gathering, or a business lunch.
Data Sources for Restaurant Information
Finding accurate and comprehensive restaurant data is crucial for any location-based service, whether it’s a simple recommendation engine or a complex food delivery platform. The sheer volume of restaurants, coupled with constantly changing menus, hours, and reviews, necessitates leveraging multiple data sources to build a robust and reliable system. This involves understanding the strengths and weaknesses of each source and employing efficient methods to extract the information you need.
The accuracy and comprehensiveness of restaurant data vary significantly across different sources. Choosing the right sources depends heavily on your specific needs – are you looking for a broad overview of restaurants in a given area, or are you focused on specific types of cuisine or price ranges? The approach to data extraction will also vary, ranging from simple API calls to more complex web scraping techniques.
Google Maps
Google Maps is a ubiquitous source of restaurant information, boasting a massive database encompassing millions of establishments worldwide. Its strength lies in its broad coverage and relatively high accuracy regarding location data. However, the comprehensiveness of information beyond location (e.g., menus, hours, reviews) can be inconsistent, varying greatly from one restaurant to another. Google provides a well-documented Places API, allowing developers to programmatically access and extract restaurant details. This API provides structured data, making it easier to integrate into applications. Data extraction is relatively straightforward, requiring authentication and well-structured API calls. However, relying solely on Google Maps might miss smaller, locally-owned restaurants not yet indexed.
Yelp
Yelp is a user-review-focused platform with a significant amount of restaurant data. Its strength lies in its user-generated reviews, providing valuable insights into customer experiences. Yelp’s data is often quite comprehensive, including menus, photos, hours, and price ranges. However, the accuracy of information can be variable due to the nature of user-submitted content; outdated or inaccurate information may exist. Yelp offers a business API for accessing data, but it requires careful consideration of rate limits and API usage policies. Web scraping is an alternative, but it’s crucial to respect Yelp’s terms of service to avoid legal issues. The structure of Yelp’s website makes web scraping relatively complex, requiring sophisticated techniques to parse the HTML and extract relevant information.
TripAdvisor
TripAdvisor focuses on travel-related information, including restaurants. It’s known for its extensive user reviews and ratings, providing a good overview of restaurant popularity and quality. Similar to Yelp, the accuracy of information can be inconsistent, relying heavily on user contributions. TripAdvisor also provides an API, but its functionality might be more limited than Google’s or Yelp’s, possibly lacking specific data points you might require. Web scraping is also possible but requires similar caution as with Yelp regarding terms of service and website structure.
Restaurant Websites
Accessing information directly from restaurant websites offers the most accurate and up-to-date data, as restaurants have direct control over the content. However, this approach is labor-intensive, requiring individual visits to each website. Data extraction methods range from manual copy-pasting to automated web scraping using tools like Python’s Beautiful Soup library. The challenge lies in the variability of website structures; each restaurant’s website may have a unique design, requiring custom scraping solutions for each. This method is best suited for a small number of restaurants or when very specific and reliable information is needed.
Organizing Restaurant Information
Effective organization of restaurant data is crucial for a seamless user experience. A well-structured presentation allows users to quickly find relevant information and make informed decisions about where to dine. This involves choosing the right data structures and presentation methods to best serve the user’s needs. We’ll explore several approaches to ensure your restaurant listings are easily navigable and insightful.
Places to eat near us – The key to successful restaurant data organization is to prioritize user experience. Consider how users search for restaurants – often by cuisine, price, location, or rating. Your organizational strategy should reflect these common search patterns to minimize friction and maximize findability.
Restaurant Data in a Responsive HTML Table
A well-designed HTML table provides a clear and concise way to display restaurant information. The use of responsive design ensures the table adapts seamlessly to various screen sizes, enhancing the user experience across devices.
Name | Address | Cuisine | Average Price |
---|---|---|---|
The Italian Place | 123 Main Street, Anytown | Italian | $25 |
Spicy Sichuan | 456 Oak Avenue, Anytown | Sichuan | $18 |
Burger Bliss | 789 Pine Lane, Anytown | American | $15 |
Sushi Sensation | 101 Maple Drive, Anytown | Japanese | $30 |
Alternative Methods for Presenting Restaurant Information
While HTML tables are effective, alternative presentation methods can enhance user engagement and information discovery. These options provide different ways to filter and browse restaurant listings, catering to diverse user preferences and search patterns.
- Categorized Lists: Organize restaurants into categories based on cuisine type (e.g., Italian, Mexican, Chinese) or other relevant attributes. This allows users to quickly browse restaurants within their preferred culinary style.
- Map Integration: Integrating a map allows users to visually locate restaurants and see their proximity to their current location or a specified address. This is particularly helpful for users prioritizing location convenience.
- Filter and Sort Options: Providing filter options (e.g., cuisine, price range, rating) and sorting capabilities (e.g., by rating, price, distance) enables users to refine their search and quickly find the most relevant results.
- Gallery View: A visual approach using images of restaurant exteriors or signature dishes can enhance user engagement and provide a quick overview of each establishment’s style and ambiance.
Organizing Restaurants by Cuisine, Price, and Rating
Organizing restaurants by cuisine, price range, and user rating allows for highly targeted filtering and sorting. This approach prioritizes the most common user search criteria, ensuring efficient information retrieval.
For example, a user might first filter by “Italian cuisine,” then refine their search by selecting a specific price range (e.g., “$15-$25”). Finally, they might sort the results by user rating to find the highest-rated Italian restaurants within their budget. This layered approach empowers users to control their search and discover relevant options quickly.
Presenting Restaurant Recommendations
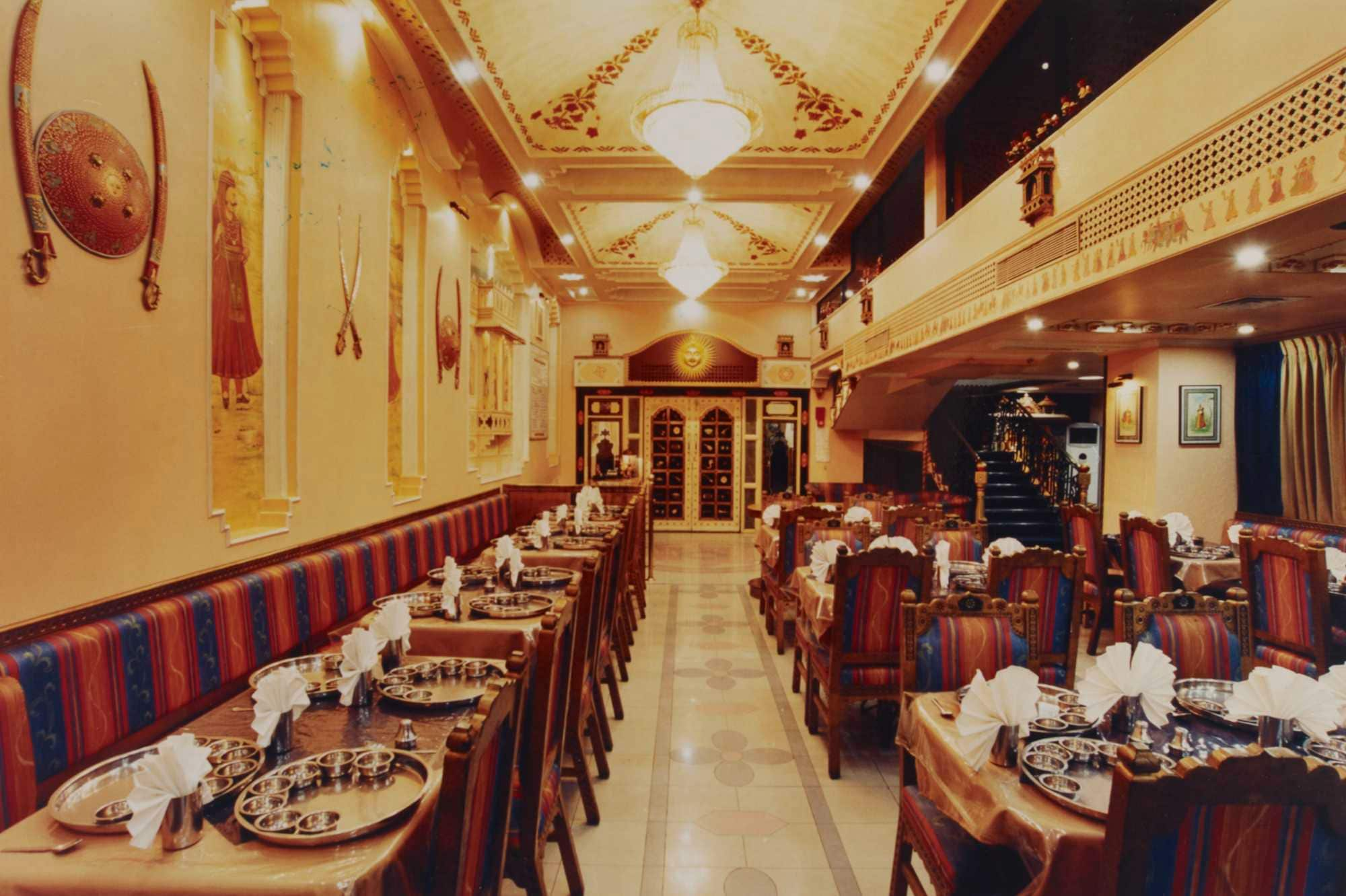
The presentation of restaurant recommendations is crucial for user engagement and ultimately, driving conversions – whether that’s a reservation or a direct visit. A poorly designed interface or confusing information can lead to users abandoning your platform and seeking alternatives. Think of it like this: you’re a personal concierge guiding users to their perfect culinary experience. Your presentation must be as polished and efficient as your recommendations are insightful.
Effective presentation hinges on a clear understanding of visual hierarchy, concise information architecture, and the strategic integration of user-generated content. We’ll explore these key elements to ensure your restaurant recommendations stand out and convert.
Visually Appealing Layouts and Concise Descriptions
A visually appealing layout is paramount. Imagine a grid-based system displaying high-quality images of each restaurant’s signature dishes or ambiance. Each restaurant listing should occupy a consistent amount of screen real estate, preventing overwhelming the user with too much information at once. Concise descriptions, focusing on the restaurant’s key selling points (e.g., “Upscale Italian with a romantic atmosphere,” or “Casual Mexican joint known for its authentic tacos”), are essential. Avoid lengthy paragraphs; instead, use bullet points to highlight key features such as price range, cuisine type, and any special offers. Consider incorporating color-coding to categorize restaurants by cuisine or price point for quick scanning and filtering. For example, a vibrant red might represent spicy cuisines, while a calming blue could represent seafood restaurants.
Incorporating User Reviews and Ratings
User reviews and ratings are invaluable social proof. Displaying a star rating prominently next to each restaurant is a must. Additionally, show a concise summary of recent reviews, highlighting both positive and negative feedback. This transparency builds trust and allows users to make informed decisions based on the experiences of others. For example, you might display three to five short snippets of recent reviews, carefully selected to showcase a balanced perspective. This approach prevents overwhelming users with lengthy reviews while still providing a representative sample of user opinions. Remember to display the number of total reviews to give users context for the star rating.
Clear and Concise Restaurant Descriptions
Clear and concise descriptions are vital for guiding user decisions. Focus on providing essential information such as the restaurant’s cuisine, price range, location (with a map link), operating hours, and any special features (e.g., outdoor seating, vegetarian options, happy hour specials). Use strong action verbs and descriptive adjectives to paint a vivid picture of the dining experience. For example, instead of saying “They have pasta,” say “They serve handmade pasta dishes with fresh, seasonal ingredients.” The use of s relevant to the user’s search query will also help improve discoverability. A well-structured description, concise yet descriptive, will help users quickly assess whether a restaurant matches their preferences.
Visual Representation of Restaurant Data: Places To Eat Near Us
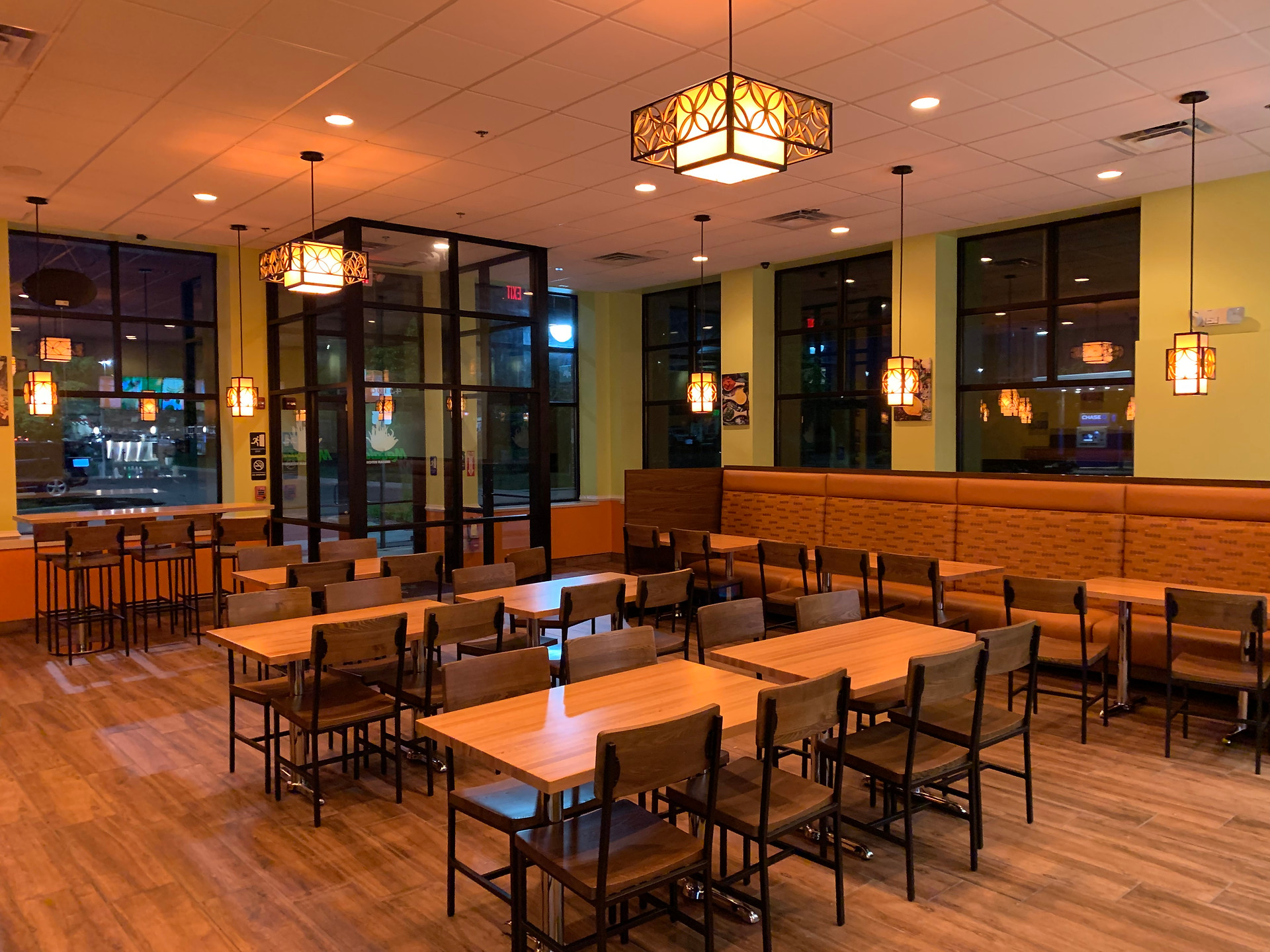
Data visualization is crucial for transforming raw restaurant information into actionable insights for users. Effective visuals can quickly communicate key details, enabling users to make informed decisions about where to eat. By leveraging the power of maps, charts, and detailed imagery, we can create a compelling and user-friendly experience that drives engagement and satisfaction.
Effective visual representation of restaurant data significantly improves the user experience. A well-designed visual interface allows users to quickly compare options, identify key features, and make informed decisions. This translates to higher user engagement and a more satisfying search experience.
Map with Color-Coded Price Ranges
Imagine a clean, interactive map centered on the user’s location. Each restaurant is represented by a pin, with the color of the pin indicating its price range. For instance, green pins could represent budget-friendly options (under $15 per person), yellow pins mid-range restaurants ($15-$30), and red pins higher-end establishments (over $30). The map itself would be highly customizable, allowing users to filter by cuisine type, rating, or other relevant criteria. This visual instantly provides a clear overview of dining options within their proximity and their respective price points, eliminating the need for tedious scrolling through lists. Furthermore, the map could incorporate street-view imagery to offer a quick visual preview of the restaurant’s exterior. This enhances the user’s understanding of the location and overall vibe, even before clicking on a specific marker.
Comparison Chart of Ratings and Price Points
This chart would provide a concise side-by-side comparison of selected restaurants. Each restaurant would occupy a row, with columns displaying key metrics such as average rating (on a star scale, perhaps from Google Reviews or Yelp), price range (using the color-coding from the map), cuisine type, and perhaps a short descriptor of the restaurant’s style (e.g., “casual,” “fine dining”). This allows users to quickly compare options based on their preferred price point and desired level of quality, facilitating a faster decision-making process. For example, a user could easily see that Restaurant A has a 4.5-star rating and a mid-range price point, while Restaurant B boasts a 4-star rating but is more budget-friendly. This comparison chart would empower users to make informed choices based on their priorities.
Restaurant Ambiance: Interior and Exterior Imagery
Instead of relying solely on text descriptions, imagine high-quality photographs showcasing both the interior and exterior of each restaurant. For the exterior, a wide shot would highlight the building’s architecture, landscaping, and overall curb appeal. Interior shots could showcase different areas of the restaurant – the dining room, bar area, patio – to give users a complete picture of the ambiance. High-resolution images, possibly even interactive 360° views, would allow users to virtually “walk through” the restaurant, helping them assess whether the atmosphere aligns with their preferences. For instance, a photo might show a bustling, lively atmosphere in one restaurant, contrasting with a more quiet and intimate setting in another. This visual element is critical in allowing users to connect with the restaurant on an emotional level and make a selection that suits their mood and dining preferences.
Handling User Preferences and Location
Precisely pinpointing a user’s location and understanding their preferences are paramount to delivering relevant and valuable restaurant recommendations. Ignoring these crucial factors results in a frustrating user experience, driving traffic away and hindering your app’s success. By effectively integrating location data and preference filters, you dramatically increase user engagement and satisfaction.
Leveraging user location data and preferences transforms a generic restaurant listing into a personalized culinary journey. This approach isn’t just about convenience; it’s about providing a tailored experience that caters to individual needs and desires, fostering loyalty and repeat usage. We’ll explore how to seamlessly incorporate these elements to elevate your restaurant recommendation system.
User Location Data Integration
Integrating user location data refines search results significantly. This typically involves requesting permission to access the user’s location through their device’s GPS or IP address. Accuracy varies; GPS provides precise coordinates, while IP addresses offer a broader approximation. Regardless of the method, the location data serves as the central point for determining proximity to restaurants. We can then use algorithms to calculate distances and filter results based on a user-defined radius (e.g., “restaurants within 5 miles”). Consider implementing options for users to manually input their location if automatic location services are unavailable or inaccurate. This fallback ensures accessibility for all users.
Handling Dietary Restrictions and Cuisine Preferences
User preferences extend beyond location; dietary restrictions and cuisine preferences are equally important. This requires a robust system for collecting and processing this information. A clear and intuitive interface is essential. Users should be able to easily select from pre-defined options (e.g., vegetarian, vegan, gluten-free, halal) or provide custom details. The system should then filter the restaurant database to display only those establishments that cater to these specified preferences. For example, a user specifying “vegetarian” should only see restaurants offering vegetarian options, while a user specifying “Italian” would only see Italian restaurants. This requires accurate and up-to-date information about each restaurant’s menu and offerings.
Filtering and Sorting Restaurant Results
Once location and preferences are factored in, the results need to be presented in a clear and organized manner. Filtering allows users to narrow down the results further based on specific criteria, such as price range, rating, or specific amenities (e.g., outdoor seating, delivery options). Sorting options, such as sorting by distance, rating, or price, allow users to prioritize results according to their preferences. A well-designed filtering and sorting system empowers users to fine-tune their search and discover the perfect restaurant for their needs. For instance, a user could filter for Italian restaurants within a 2-mile radius, then sort the results by rating to see the highest-rated options first. This level of control is crucial for a positive user experience.