Understanding User Intent

The seemingly simple search query “best food restaurants near me” hides a surprising depth of user intent. Understanding the nuances behind this phrase is crucial for optimizing restaurant listings and attracting the right clientele. The query isn’t just about finding any restaurant; it reflects a specific need and a set of expectations at a particular moment.
The interpretation of “best food restaurants near me” varies wildly depending on the user’s context. It’s not a monolithic request, but rather a spectrum of needs and desires. For example, a business lunch requires a different type of restaurant than a romantic dinner, and a family outing has different priorities than a quick solo meal.
User Needs Implied by the Search, Best food restaurants near me
The user’s search implies a need for convenience, quality, and a specific type of dining experience. They’re looking for something within a reasonable proximity, suggesting a lack of time or desire for extensive travel. The term “best” indicates a desire for high quality, whether that means exceptional cuisine, excellent service, a unique atmosphere, or a combination of these factors.
For example, a user searching on a weekday lunch break might prioritize speed and affordability, seeking a restaurant with quick service and a reasonable price point. In contrast, a user searching on a weekend evening might prioritize ambiance and a more leisurely dining experience, perhaps opting for a restaurant with a lively atmosphere and a curated menu. A family with young children might prioritize kid-friendly options, high chairs, and a relaxed environment.
Factors Influencing Restaurant Choice
Numerous factors influence a user’s final restaurant selection. These can be broadly categorized into:
- Cuisine Type: The user might be craving Italian, Mexican, Thai, or any other specific cuisine. This is a primary driver of their choice.
- Price Range: Budget is a significant constraint. The search implies a willingness to pay a certain amount, which varies greatly depending on the occasion and the user’s financial situation. A business lunch might have a higher budget than a casual weeknight dinner.
- Location and Accessibility: Proximity is paramount. “Near me” implies a desire for convenience, considering factors like driving distance, parking availability, and public transportation access.
- Reviews and Ratings: Online reviews and star ratings heavily influence user decisions. Positive reviews build trust and confidence, while negative reviews can be a significant deterrent.
- Ambiance and Atmosphere: The desired atmosphere can range from casual and family-friendly to romantic and upscale. The occasion and the user’s preferences heavily impact this choice.
- Dietary Restrictions and Preferences: Users might have specific dietary needs or preferences, such as vegetarian, vegan, gluten-free, or allergy-related considerations. Restaurants catering to these needs are highly sought after.
- Special Offers and Promotions: Discounts, deals, and happy hour specials can sway a user’s decision, especially when budget is a factor.
Understanding these varied interpretations and influencing factors is crucial for restaurants to effectively target their marketing efforts and improve their online visibility. Optimizing online profiles to highlight relevant details and cater to specific user needs is key to attracting the right customers.
Data Sources and Collection
Finding the best restaurants near you requires more than just a hunch; it demands a strategic approach to data gathering. This involves leveraging multiple sources and employing critical evaluation to ensure the information you’re using is accurate and reliable, leading you to truly exceptional dining experiences. Think of it as conducting market research, but for your stomach.
To build a robust and accurate database of local restaurants, you need a multi-pronged approach. This isn’t about simply Googling “best restaurants near me” and taking the top results at face value. Instead, it’s about intelligently combining data from various sources to get a comprehensive picture.
Online Review Platforms
Online review platforms like Yelp, Google Reviews, TripAdvisor, and Zomato are goldmines of user-generated data. These platforms offer a vast amount of information, including ratings, reviews, photos, and menus. However, it’s crucial to remember that user reviews can be subjective and potentially biased. Some users might be more prone to leaving reviews than others, skewing the overall rating. Furthermore, businesses can sometimes incentivize positive reviews or try to suppress negative ones, compromising the objectivity of the data. Analyzing review trends over time and comparing ratings across multiple platforms can help mitigate this bias. For example, a restaurant consistently receiving high ratings across various platforms is likely more reliable than one with a few exceptionally high ratings on a single platform.
Social Media Data
Social media platforms such as Instagram, Facebook, and TikTok provide another rich source of information. Restaurants often post photos of their food, menus, and ambiance. User-generated content, including posts, stories, and check-ins, offers valuable insights into customer experiences and preferences. Analyzing the sentiment expressed in these posts—positive, negative, or neutral—can provide a nuanced understanding of public opinion. For example, a high volume of positive posts with engaging photos indicates a potentially popular restaurant. However, similar to online reviews, social media data requires careful analysis to account for potential biases and marketing efforts. Look for genuine, unsponsored reviews and posts to gain a clearer picture.
Local Directories and Business Listings
Local directories like Yellow Pages (though less popular now), Nextdoor, and even dedicated city or region websites often list local businesses, including restaurants. These listings usually include basic information like address, phone number, operating hours, and sometimes menus. While less detailed than review platforms, they provide a crucial layer of verification and can help identify restaurants not prominently featured on review sites. Cross-referencing information from different sources can improve the accuracy and completeness of your restaurant database. For example, verifying the address and operating hours from a local directory with information found on a review platform can confirm the accuracy of the data.
Criteria for Evaluating Restaurant Data Reliability
Evaluating the reliability of restaurant data requires a multi-faceted approach. Consider the following criteria:
- Consistency Across Platforms: A restaurant with consistently high ratings across multiple platforms is more likely to be reliable than one with widely varying ratings.
- Volume of Reviews/Posts: A large volume of reviews or social media posts suggests a higher level of engagement and provides a more statistically significant sample size.
- Recency of Reviews/Posts: Recent reviews and posts are more relevant than older ones, as they reflect current customer experiences.
- Diversity of Reviews/Posts: A diverse range of reviews and posts, encompassing both positive and negative feedback, suggests a more objective representation of customer experiences.
- Verification of Information: Cross-referencing information from multiple sources, such as review platforms, social media, and local directories, can help verify the accuracy of the data.
Restaurant Ranking and Selection
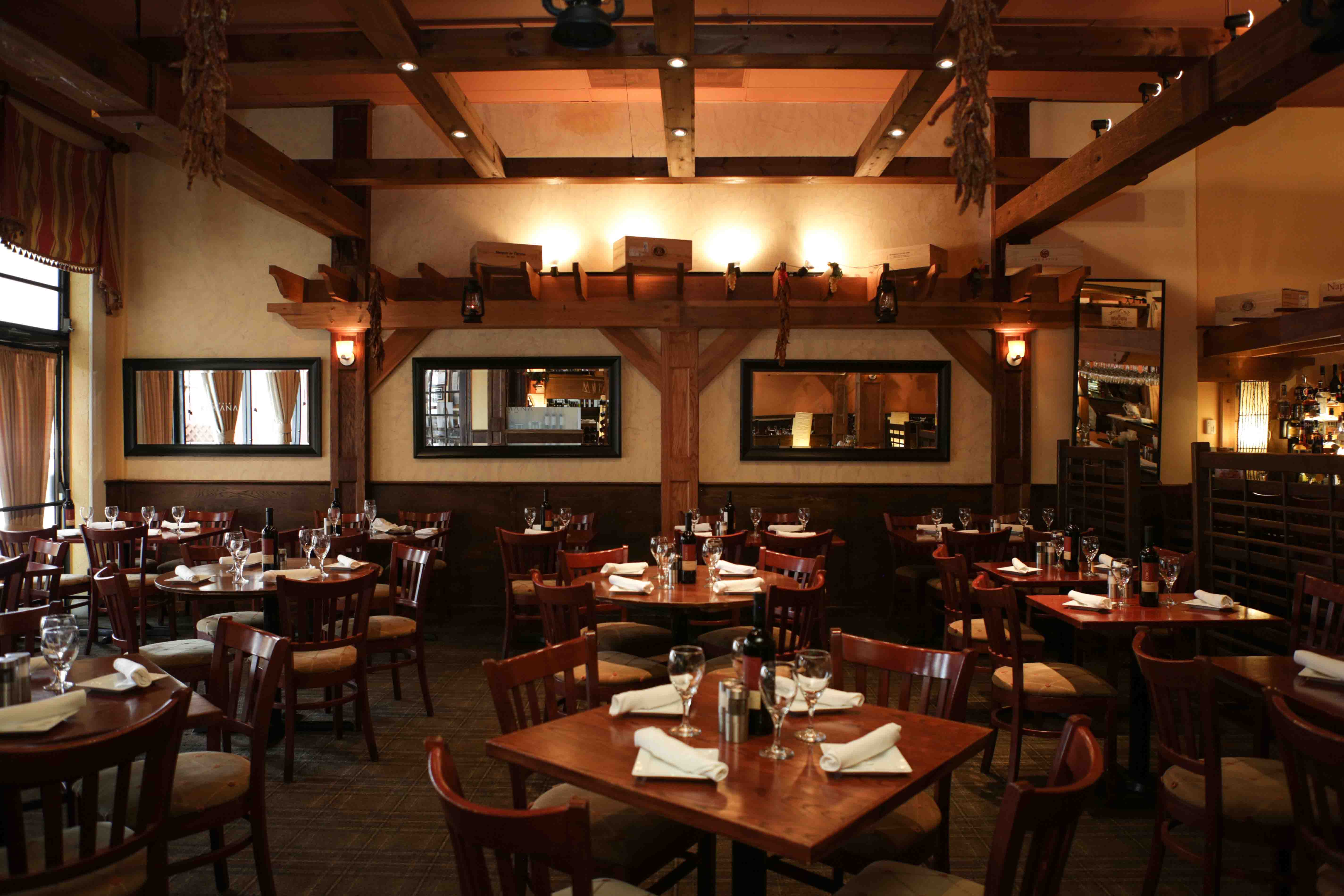
Finding the perfect restaurant near you shouldn’t feel like navigating a maze. A robust ranking system, leveraging data and sophisticated algorithms, is key to delivering a seamless and accurate user experience. This system needs to go beyond simple star ratings, considering various factors that influence a diner’s choice.
Best food restaurants near me – Our ranking system prioritizes a multi-faceted approach, combining quantitative data with qualitative feedback to provide a comprehensive and nuanced restaurant ranking. This ensures that the results are not only accurate but also reflect the diverse preferences of our users.
Restaurant Ranking Algorithm
The core of our restaurant ranking algorithm lies in a weighted scoring system. Each factor—user reviews, ratings, cuisine type, price range, and location—contributes to a final score, but not equally. We’ve carefully determined weighting based on user behavior data and industry best practices. The weighting allows us to prioritize factors most important to users while still providing a comprehensive picture of each restaurant.
For example, user reviews and ratings carry a heavier weight (60%) as they directly reflect diner satisfaction. Cuisine type and price range contribute 20% each, allowing users to filter results based on their preferences. Location is weighted at 10%, ensuring that restaurants closest to the user are given preference. This system is dynamic and can be adjusted based on ongoing analysis of user behavior and feedback.
Sample Restaurant Data
The following table showcases how our ranking system works in practice. Each restaurant’s score is calculated using the weighted algorithm described above. Note that these are sample data points and not reflective of any specific establishment.
Restaurant Name | Cuisine | Average Rating | Price Range | Location | Ranking Score |
---|---|---|---|---|---|
The Golden Spoon | Italian | 4.5 | $$ | Downtown | 87 |
Spice Fusion | Indian | 4.2 | $$$ | Suburbs | 78 |
Burger Bliss | American | 4.0 | $ | Downtown | 72 |
Sushi Sensation | Japanese | 4.8 | $$$$ | Suburbs | 85 |
Restaurant Feature Comparison: Best Food Restaurants Near Me
Choosing the perfect restaurant involves more than just looking at menus. A truly informed decision requires a nuanced understanding of each establishment’s unique strengths and weaknesses. This comparison focuses on key features to help you find the best fit for your needs and preferences. We’ll analyze aspects like ambiance, service quality, menu diversity, and accommodation of dietary restrictions, highlighting crucial differentiators to guide your selection.
Understanding these differences is critical for maximizing your dining experience. For example, a romantic dinner calls for a different atmosphere than a casual lunch with friends. Similarly, a family with young children might prioritize kid-friendly menus and relaxed service over a Michelin-starred experience.
Ambiance and Atmosphere
The ambiance of a restaurant significantly impacts the overall dining experience. Consider factors like lighting, music, décor, and seating arrangements. A bustling, vibrant atmosphere might suit a lively group of friends, while a quieter, more intimate setting is ideal for a romantic date. For example, imagine comparing a brightly lit, modern bistro with a dimly lit, candlelit Italian trattoria. The former offers a more energetic vibe, perfect for a quick lunch or casual gathering, while the latter provides a sophisticated and romantic ambiance. The key differentiator here is the desired mood and the type of social gathering.
Service Quality and Efficiency
Exceptional service elevates any dining experience. This includes factors such as attentiveness of staff, speed of service, knowledge of the menu, and overall professionalism. A restaurant with highly trained, friendly servers who anticipate needs will offer a superior experience compared to one with slow or inattentive staff. For instance, a fine-dining establishment is expected to have impeccable service, with servers knowledgeable about wine pairings and menu details. Conversely, a fast-casual restaurant might prioritize speed and efficiency over extensive personalized service. The differentiator here lies in the level of service expected and the overall dining experience priority.
Menu Options and Dietary Restrictions
The menu is paramount. Consider the breadth and depth of offerings, including vegetarian, vegan, gluten-free, and other dietary options. A restaurant with a diverse menu catering to various dietary needs will attract a wider customer base. For example, a restaurant boasting extensive vegetarian and vegan options will appeal to a broader audience than one with limited choices. A restaurant specializing in gluten-free options will attract customers with celiac disease or gluten intolerance. The key differentiator here is the inclusivity and adaptability of the menu to accommodate diverse dietary requirements.
Price Point and Value for Money
The price point is a significant factor influencing restaurant selection. It’s crucial to consider not only the cost of the meal but also the overall value provided. A high-priced restaurant might offer exceptional quality and service justifying the cost, while a more affordable option might provide excellent value without compromising quality. For example, a high-end steakhouse will likely have higher prices reflecting the quality of ingredients and service, while a local diner might offer a more budget-friendly meal with satisfactory quality. The key differentiator is the balance between price and the overall dining experience received.
Visual Representation of Data
Data visualization is crucial for transforming complex restaurant information into easily digestible insights for users. A well-designed visual representation can significantly improve user engagement and decision-making, leading to higher conversion rates and a better overall user experience. Think of it as the difference between sifting through a spreadsheet and glancing at a beautifully crafted map highlighting the best pizza places near you.
Effective visual representation of restaurant data should prioritize clarity, conciseness, and intuitive understanding. This means avoiding clutter and focusing on the most important information—location, ratings, and perhaps a few key features like price range or cuisine type. The goal is to make it effortless for users to identify restaurants that meet their needs.
Interactive Map with Rating Overlays
An interactive map provides the most intuitive way to display restaurant locations. Imagine a Google Maps-style interface where each restaurant marker is color-coded based on its average rating. For example, restaurants with a 4.5-star rating and above could be represented by vibrant green markers, those with 3.5 to 4.4 stars by yellow markers, and those below 3.5 stars by red markers. The color scheme should be intuitive and universally understood—green for good, red for bad. Each marker should display a small pop-up window with the restaurant’s name, rating, and perhaps a thumbnail image. Users could then zoom in and out, pan across the map, and click on individual markers to get more details. This visual approach immediately conveys location and quality, allowing users to quickly identify potential dining options in their desired area.
Rating Distribution Chart
To provide a more granular view of restaurant ratings, a histogram or bar chart showing the distribution of ratings across all restaurants could be beneficial. The horizontal axis would represent the rating range (e.g., 1-1.5 stars, 1.5-2 stars, and so on), while the vertical axis would represent the number of restaurants falling within each rating range. This visualization helps users understand the overall quality distribution of restaurants in their area, revealing whether most restaurants are highly rated or if there’s a wider spread of quality. A simple, clean color scheme—perhaps shades of blue progressing from light to dark with increasing rating—would make the chart easy to read. Clear labeling of axes and data points is crucial for easy interpretation. This chart provides valuable context beyond individual restaurant ratings.
Example of Effective Visual Elements
Consider a restaurant listing that displays a large, high-quality image of a signature dish alongside the restaurant’s name, rating (using a star rating system), price range (represented by dollar signs), and cuisine type (using clear icons). This combination of visual and textual information creates a compelling and informative representation, making it easy for users to quickly assess the restaurant’s appeal. Furthermore, incorporating user reviews (perhaps as short snippets or a summary) adds social proof and builds trust. The overall design should be clean, modern, and consistent with the overall aesthetic of the application or website.
User Experience Considerations
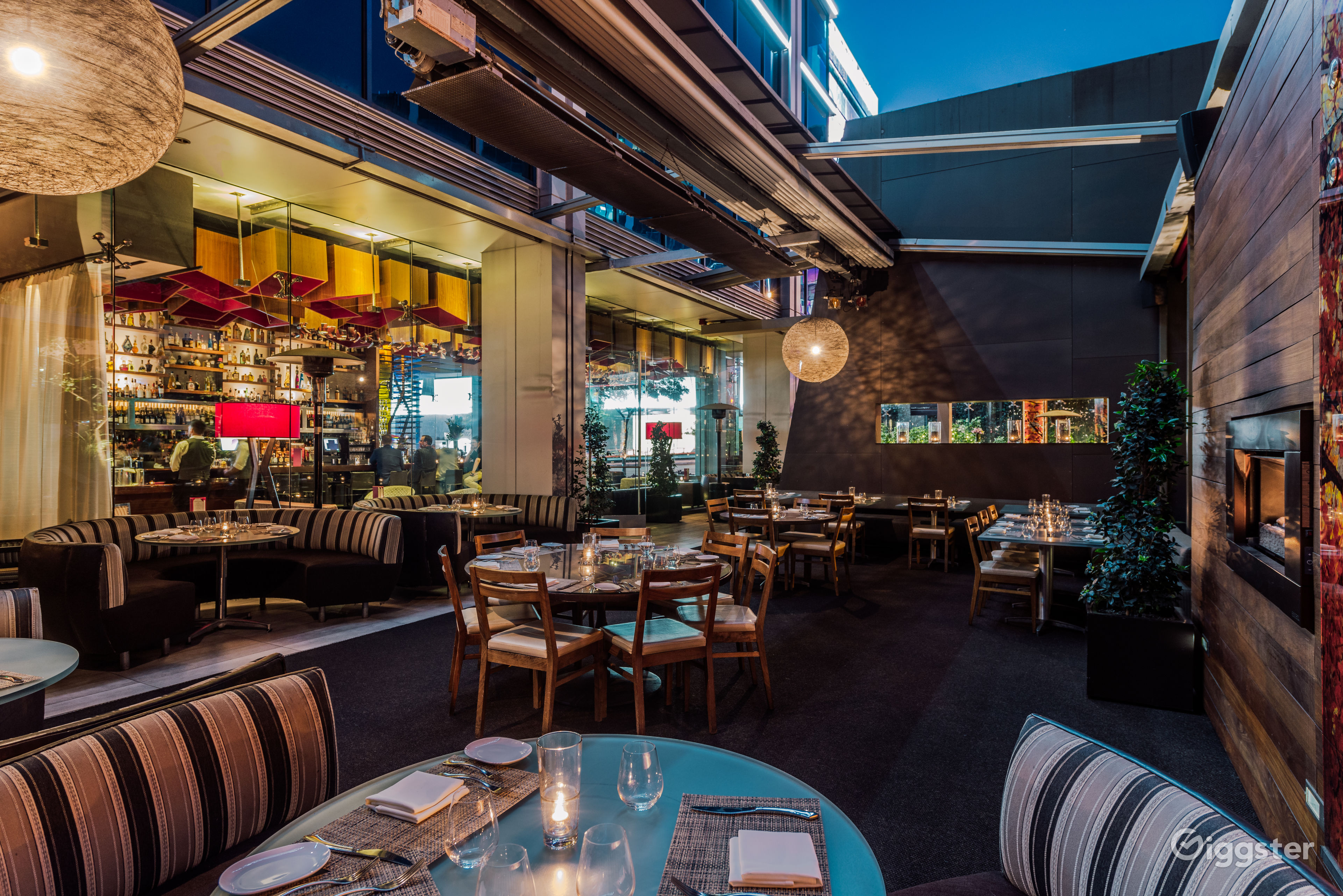
A seamless and enjoyable user experience is paramount for any successful online tool, and a restaurant finder is no exception. Without a user-friendly interface, even the most comprehensive data will fail to attract and retain users. The key is to present information clearly, intuitively, and efficiently, transforming a potentially tedious search into a delightful discovery. This section details the critical aspects of UX design that contribute to a superior user experience in a restaurant recommendation system.
Clear and concise presentation of restaurant information is crucial for quick decision-making. Users shouldn’t have to wade through excessive text or confusing layouts to find essential details. Imagine searching for a quick lunch – you need the restaurant’s name, location, cuisine type, price range, and maybe a couple of high-quality images, all readily accessible. Anything beyond that, while potentially useful, should be easily collapsible or expandable based on user preference. Think of Amazon’s product pages – concise, yet comprehensive.
Intuitive Interface Design for Filtering and Sorting
The ability to filter and sort restaurant results is essential for efficient searching. A well-designed interface allows users to quickly narrow down their options based on various criteria, such as cuisine type, price range, location, rating, and dietary restrictions. Consider implementing a series of dropdown menus or checkboxes for each filter category. These should be clearly labeled and easy to understand, allowing users to combine multiple filters to refine their search results with ease. For example, a user might filter for “Italian,” “$$,” “within 1 mile,” and “4-star rating or higher” simultaneously. The sorting mechanism should allow users to order results by relevance, distance, rating, price, or other relevant factors. This granular control empowers users to personalize their search and find exactly what they’re looking for.
Potential User Interface Improvements
To further enhance the user experience, several interface improvements can be implemented.
These improvements can significantly impact user satisfaction and engagement. Prioritizing these enhancements can transform a functional tool into a truly exceptional user experience.
- Interactive Map Integration: Allow users to visually explore restaurants on a map, identifying locations and distances intuitively. Color-coding could highlight different cuisine types or price ranges directly on the map.
- Advanced Search Functionality: Implement a powerful search bar that supports searches, allowing users to search by dish names, specific ingredients, or even dietary restrictions (e.g., “gluten-free pasta”).
- Personalized Recommendations: Leverage user history and preferences to suggest restaurants they might enjoy. This personalized approach enhances engagement and increases the likelihood of users finding restaurants that perfectly suit their tastes.
- High-Quality Imagery and Videos: Showcase restaurants with professional-quality photos and videos of their food and ambiance. Visual appeal is critical in enticing users to choose a particular restaurant.
- User Reviews and Ratings: Incorporate a robust review system that allows users to share their experiences and rate restaurants. This social aspect enhances trust and provides valuable insights to other users.
- Offline Functionality (Optional): For users who may not always have internet access, consider allowing users to save their favorite restaurants or searches for offline viewing.
Handling Diverse Cuisine Preferences
Catering to diverse culinary tastes is paramount for a successful food recommendation system. Understanding the nuances of different cuisines and providing effective filtering options is key to delivering a personalized and satisfying user experience. Ignoring this aspect can lead to frustrated users and a diminished user base. We’ll explore common cuisine types, their characteristics, and effective categorization strategies.
Cuisine categorization should be both broad and detailed enough to capture user preferences accurately. A simple approach using broad categories like “American,” “Italian,” or “Mexican” might suffice for some, but a more sophisticated system needs to accommodate finer distinctions within each category. For example, within “Italian,” you might differentiate between Roman, Neapolitan, or Sicilian styles. This granularity significantly enhances the precision of recommendations.
Cuisine Type Categorization and Filtering
Effective categorization involves structuring cuisine types hierarchically. Top-level categories can represent broad geographical areas or culinary traditions (e.g., Asian, European, American). Subcategories can then represent more specific cuisine types (e.g., under Asian: Chinese, Japanese, Thai, Indian; under European: Italian, French, Spanish). This hierarchical structure enables users to refine their searches progressively, moving from broad to specific preferences. Filters should allow users to select multiple cuisines simultaneously, accommodating those with varied preferences. For instance, a user might select “Italian” and “Mexican” simultaneously, showcasing the system’s flexibility.
Sample Cuisine Types and Restaurant Examples
The following list illustrates a sample of cuisine types and example restaurants. Remember that specific restaurant names are illustrative and will vary based on location. The goal is to showcase the breadth of options and the level of detail achievable in a well-structured system.
- American: Classic Diner, Steakhouse, BBQ Joint
- Italian: Pizzeria Napoletana, Trattoria Romana, Pasta House
- Mexican: Taqueria, Cantina, Mole Restaurant
- Chinese: Cantonese Restaurant, Sichuan Restaurant, Peking Duck House
- Japanese: Sushi Restaurant, Ramen Shop, Izakaya
- Indian: North Indian Restaurant, South Indian Restaurant, Indo-Chinese Restaurant
- French: Bistro, Brasserie, Haute Cuisine Restaurant
- Thai: Thai Street Food, Northern Thai Restaurant, Southern Thai Restaurant
Addressing User Location Accuracy
Pinpointing a user’s precise location is paramount for delivering truly relevant restaurant recommendations. Inaccurate location data leads to frustration, wasted time, and ultimately, a poor user experience. Getting this right is crucial for the success of any location-based service, especially one focused on the highly competitive food and beverage industry. We need to leverage multiple methods to ensure accuracy and gracefully handle situations where precise location information isn’t readily available.
The challenges associated with imprecise location data are multifaceted. GPS signals can be weak or unreliable indoors, leading to significant location errors. Network-based location services can also be inaccurate, particularly in areas with poor cellular coverage or ambiguous IP addresses. These inaccuracies directly impact the recommendations; a user searching for “best Italian near me” might receive results miles away from their actual location, leading to dissatisfaction. Further complicating the issue is the potential for users to intentionally or unintentionally provide inaccurate location information.
Methods for Accurate Location Determination
To combat these challenges, a multi-pronged approach is necessary. We should prioritize using multiple location services simultaneously. This might involve combining GPS data with Wi-Fi triangulation and IP address geolocation. Each method has its strengths and weaknesses; combining them helps to compensate for individual limitations. For example, GPS is highly accurate outdoors but unreliable indoors, while Wi-Fi triangulation excels in indoor environments but requires access to a sufficient number of Wi-Fi networks. By intelligently weighting the results from each method, we can achieve a higher degree of accuracy. Furthermore, we can employ techniques like location history and user confirmation to refine the location estimate over time. For instance, if a user consistently orders from restaurants in a particular area, the system can adjust the location based on this behavior.
Handling Users Who Do Not Share Their Location
Users may choose not to share their location for privacy reasons, which is a legitimate concern that must be respected. However, not providing location information significantly limits the functionality of a location-based service. Therefore, we need a robust strategy to provide a useful experience even without precise location data. One approach is to offer a manual location input. Users can type in their address or search for a specific landmark. Another method is to provide a broader search radius, showing results from a larger area. This approach sacrifices precision but ensures the user still receives relevant recommendations. We can also guide users to explicitly enable location services within the app, clearly explaining the benefits of providing location data while emphasizing the respect for their privacy. Transparency and user control are essential here. A clear and concise privacy policy outlining how location data is used and protected is crucial for building trust.
Addressing Location Error and Correction
Location errors are inevitable. To mitigate the impact of such errors, the system should incorporate mechanisms for user feedback. Allowing users to correct their location or report inaccurate results is critical. This feedback loop is invaluable for improving the accuracy of the location services and enhancing the user experience. We can also implement algorithms that identify and flag potentially inaccurate locations based on patterns of inconsistent user behavior or conflicts between different location sources. For example, if a user repeatedly reports results as being too far away, the system can investigate potential issues with the location data. Furthermore, regular audits and updates of location databases and algorithms are essential for maintaining accuracy and addressing emerging challenges.