Understanding User Intent Behind “Food Near Me That’s Open”
The seemingly simple search query, “food near me that’s open,” reveals a complex tapestry of user needs and intentions. Understanding these nuances is crucial for businesses aiming to capture this high-intent traffic. The urgency inherent in the phrase “that’s open” significantly impacts the user’s decision-making process and their expectations of the search results.
The phrase reflects a user’s immediate need for sustenance, often driven by hunger, a specific craving, or a planned meal. The proximity (“near me”) indicates a desire for convenience and speed, minimizing travel time and effort. This is a high-conversion search query, implying a strong purchase intent.
User Locations and Contexts
The user’s location significantly influences their search. They could be at home, seeking a quick and easy dinner option; at work, looking for a lunchtime escape; or traveling, in need of a reliable and readily available meal. Consider a business traveler arriving late at night in an unfamiliar city – their need for “food near me that’s open” is intensely urgent and location-specific. Conversely, someone at home might have more flexibility in their choice of restaurant and be willing to travel a bit further.
Urgency Implied by “That’s Open”
The addition of “that’s open” underscores the time-sensitive nature of the search. Users aren’t browsing; they’re actively seeking an immediate solution to their hunger. This implies a strong preference for establishments with confirmed operating hours and a desire to avoid wasted time and effort. Imagine a family on a road trip, their stomachs rumbling – the “that’s open” element becomes paramount in their search. They need assurance that the restaurant will be there, ready to serve them.
Types of Food Sought
The type of food desired is highly variable and depends on numerous factors, including time of day, personal preferences, dietary restrictions, and budget. Users might be searching for anything from a quick burger and fries to a fine-dining experience. They might be seeking specific cuisines, like Italian, Mexican, or Thai, or looking for something healthier, like a salad or a vegetarian option. The search query itself doesn’t reveal these preferences, making it crucial for businesses to optimize their online presence to cater to this diverse range of needs. For example, a late-night pizza place will attract a different user than a high-end sushi restaurant, even though both would appear in a “food near me that’s open” search.
Data Sources for Finding Open Restaurants: Food Near Me Thats Open
Finding real-time information about restaurants’ operating hours is crucial for any food delivery or discovery app. The accuracy and reliability of this information directly impact user experience and satisfaction. A robust system needs to integrate multiple data sources to compensate for individual limitations and ensure the most up-to-date information is available.
Food near me thats open – Leveraging diverse data sources is essential for building a reliable system. Each source offers unique strengths and weaknesses, necessitating a thoughtful approach to integration and data validation.
Potential Data Sources
Several sources can provide real-time restaurant information, each with varying levels of accuracy and coverage. Strategic integration of these sources is key to maximizing the accuracy of your system.
- Restaurant APIs: Many restaurant chains and aggregators offer APIs that provide their operating hours and other relevant data. Examples include Yelp’s Fusion API, or APIs offered directly by restaurant chains themselves. These APIs generally offer high accuracy for the specific restaurants they cover but lack comprehensive coverage.
- Third-Party Data Providers: Companies specialize in aggregating restaurant data from various sources, including direct partnerships with restaurants, scraping publicly available information, and user contributions. These providers often offer broader coverage than individual restaurant APIs but might have lower accuracy due to the reliance on aggregated data. Examples include Foursquare and similar location data providers.
- Google Places API: This API provides information on businesses, including restaurants, and can include operating hours. However, the accuracy relies heavily on the accuracy of the information submitted by the businesses themselves or users, which can be inconsistent.
- Direct Restaurant Websites: Scraping data from restaurant websites is another option. However, this approach requires significant effort in terms of web scraping technology and maintaining compatibility with ever-changing website structures. Furthermore, data extraction is time-consuming and may not be reliably consistent across different restaurant websites.
- User-Generated Data: Incorporating user-submitted information, such as check-ins or reviews, can help supplement other data sources. However, this approach requires robust moderation and validation mechanisms to filter out inaccurate or misleading information.
Accuracy and Reliability Comparison
The accuracy and reliability of different data sources vary significantly. Direct restaurant APIs generally offer the highest accuracy for the restaurants they cover, while user-generated data tends to be less reliable but can provide valuable supplemental information.
Data Source | Accuracy | Reliability | Coverage |
---|---|---|---|
Restaurant APIs | High (for covered restaurants) | High | Limited |
Third-Party Data Providers | Medium | Medium | Broad |
Google Places API | Medium to Low | Medium to Low | Broad |
Restaurant Websites (Scraping) | Medium to Low | Low | Variable |
User-Generated Data | Low | Low | Broad (but potentially biased) |
Challenges in Maintaining Up-to-Date Information
Maintaining accurate and real-time restaurant operating hours is an ongoing challenge. Restaurants frequently change their hours due to various factors, including holidays, special events, and staffing issues. This necessitates a robust system for updating information frequently.
- Data Latency: There’s always a delay between a restaurant changing its hours and that change being reflected in data sources.
- Inconsistent Updates: Restaurants may not always update their information consistently across all platforms.
- Data Errors: Human error in data entry can lead to inaccuracies.
- Unexpected Closures: Sudden closures due to unforeseen circumstances (e.g., power outages, emergencies) are difficult to predict and reflect in real-time.
System Architecture for Integrating Multiple Data Sources
A robust system should employ a multi-layered approach to integrate various data sources and ensure data consistency and accuracy. This involves data aggregation, validation, and conflict resolution.
A potential architecture would involve a central data repository that receives data feeds from multiple sources. A data validation layer would then cleanse and verify the incoming data, identifying and resolving inconsistencies. A conflict resolution mechanism would prioritize data from more reliable sources. Finally, a caching layer would improve performance by storing frequently accessed data.
A well-designed system will prioritize real-time data, incorporate automated updates, and implement a robust error-handling mechanism to manage inconsistencies and unexpected data issues.
Presenting the Information Effectively
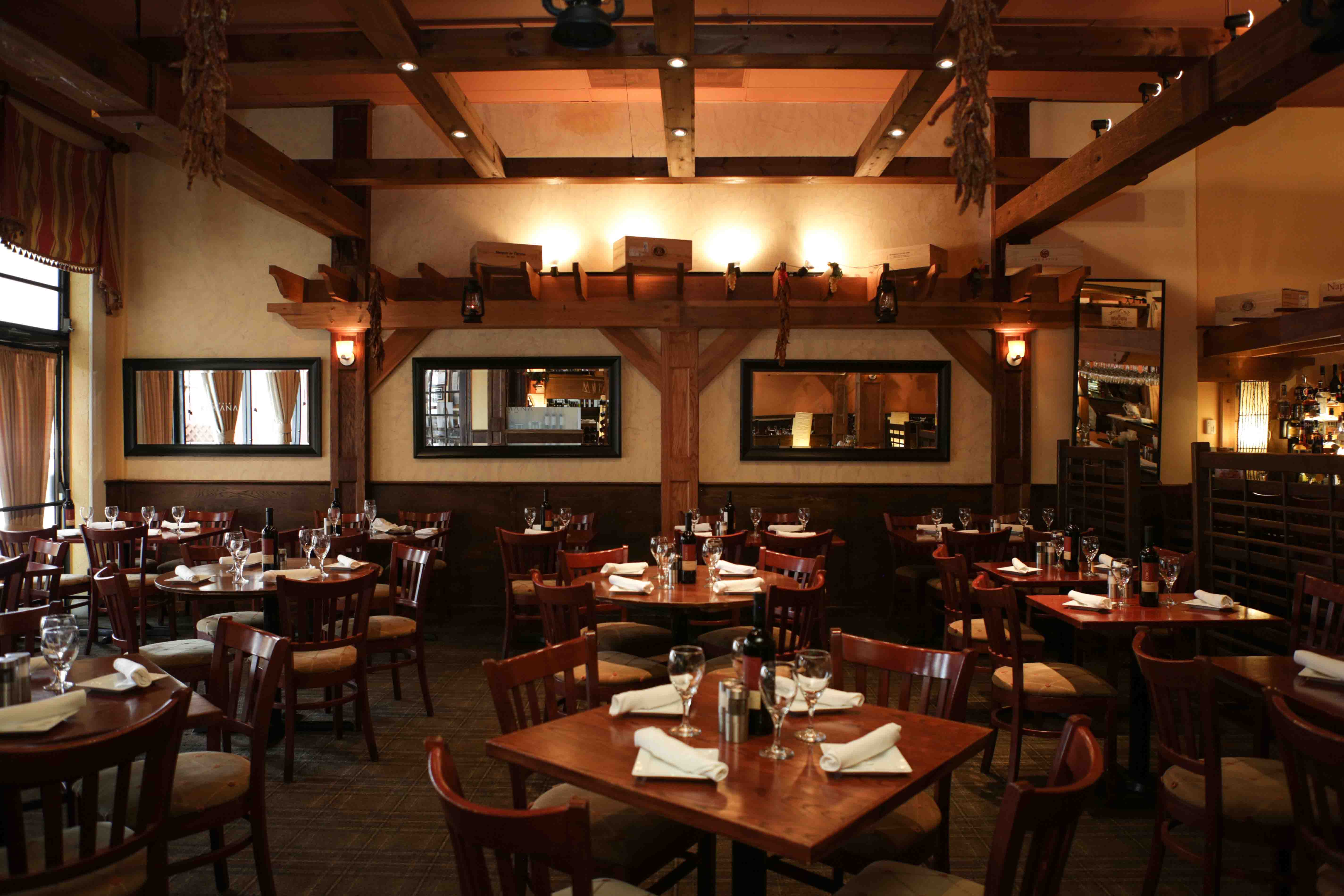
Delivering a compelling user experience when presenting “food near me” results hinges on clear, concise, and visually appealing information architecture. Users need to quickly grasp key details – restaurant name, cuisine type, distance, and operating hours – to make informed decisions. Failure to present this data effectively leads to frustration and lost potential customers for the restaurants themselves.
Effective presentation goes beyond simply listing data; it involves strategic organization and thoughtful consideration of various screen sizes and potential data gaps. We’ll explore techniques to optimize the display of this information, ensuring a seamless user experience regardless of device.
Responsive HTML Table for Restaurant Data
A well-structured HTML table is the ideal method for displaying restaurant information. The table should be responsive, adapting gracefully to different screen sizes. Consider using CSS to control column widths and potentially hide less critical information (like operating hours) on smaller screens to maintain readability.
Restaurant Name | Cuisine | Distance | Operating Hours |
---|---|---|---|
Luigi’s Pizza | Italian | 0.5 miles | 11:00 AM – 10:00 PM |
Thai Basil | Thai | 1.2 miles | 12:00 PM – 9:00 PM |
The Burger Joint | American | 2.0 miles | 11:00 AM – 11:00 PM |
Sakura Sushi | Japanese | 0.8 miles |
Handling Missing Data
Missing data, such as unavailable operating hours, should be handled gracefully. Instead of leaving empty cells, use a placeholder like “Hours not available” or “Check website for hours.” This prevents confusion and maintains a consistent user experience. Consider adding a visual cue, such as a subtle icon, to highlight restaurants with incomplete information.
Prioritizing Restaurants
Prioritization is crucial for presenting the most relevant results first. A simple method is to prioritize by distance, followed by rating (if available). Restaurants closest to the user are listed first, and within each distance bracket, restaurants with higher ratings are ranked higher. This ensures users see the most convenient and highly-rated options initially. For example, a restaurant 0.2 miles away with a 4.5-star rating would appear before a restaurant 1.5 miles away with a 5-star rating.
Handling Specific User Needs

Creating a truly useful “food near me” application requires going beyond simply displaying open restaurants. Users have diverse needs and preferences, and catering to these is crucial for user satisfaction and engagement. This means intelligently filtering results based on a variety of factors, presenting information clearly, and prioritizing user experience.
Effectively handling specific user needs translates directly into higher user engagement and retention. By providing a personalized experience, you’re not just offering a list of restaurants; you’re providing a solution tailored to individual preferences. This personalized approach significantly increases the likelihood of users finding what they need quickly and efficiently, leading to greater satisfaction and repeat usage.
Dietary Restriction Filtering
Filtering for dietary restrictions like vegetarian, vegan, and gluten-free is paramount. This requires access to reliable data sources that accurately reflect a restaurant’s menu offerings. Imagine a user searching for a vegan restaurant; a poorly implemented filter could lead them to a restaurant that only offers a single vegan dish, causing frustration. Therefore, the ideal solution involves robust data integration with menu APIs or crowdsourced data that allows for detailed dietary information tagging. This allows users to confidently select restaurants that meet their needs, leading to higher conversion rates and positive user reviews. For instance, a robust system might allow users to filter for “vegan,” “vegetarian,” “gluten-free,” and even more specific options like “nut-free” or “dairy-free.”
Incorporating User Reviews and Ratings
User reviews and ratings provide invaluable social proof and significantly impact user decisions. Integrate these reviews prominently, displaying star ratings and concise summaries. Consider visually highlighting particularly positive or negative reviews to draw attention to key aspects of the dining experience. For example, you might showcase reviews mentioning excellent service, delicious food, or specific dietary accommodations. Displaying an aggregate rating alongside the number of reviews builds trust and allows users to quickly assess the overall quality of a restaurant. Furthermore, filtering options could allow users to view only restaurants with ratings above a certain threshold, further refining their search.
Handling Different Restaurant Categories, Food near me thats open
Different restaurant categories, from fast food to fine dining, require nuanced presentation. High-end restaurants might benefit from larger images and detailed descriptions, while fast-food options might require a simpler, more concise presentation. The categorization should be clear and intuitive, allowing users to easily navigate between different types of establishments. Consider using visual cues like icons or color-coding to differentiate categories at a glance. For example, a small burger icon could represent fast food, while a formal silverware image could denote fine dining. This visual hierarchy makes it easier for users to find the type of restaurant they’re looking for quickly and efficiently.
Presenting Options for Different Price Ranges
Price is a significant factor for many diners. Clearly displaying price ranges, perhaps using a dollar sign system ($, $$, $$$), allows users to filter results based on their budget. This is especially useful in areas with a wide range of dining options, from budget-friendly cafes to upscale restaurants. Ensure the price range accurately reflects the average cost of a meal, and consider providing additional information, such as whether the price represents a meal for one person or for two. This price transparency enhances the user experience by allowing for informed decision-making and prevents unpleasant surprises. For example, a user looking for a budget-friendly lunch could easily filter results to show only restaurants with a single dollar sign ($) next to their name.
Illustrative Examples

Understanding the user experience is paramount to building a successful “food near me” application. Let’s explore several illustrative examples to solidify these concepts. We’ll delve into UI design, data integration, location-based services, and error handling, showcasing how these elements work together to provide a seamless and informative experience.
Search Results User Interface
Imagine a clean, minimalist search results page. The background is a soft, off-white (#F8F8F8) to avoid eye strain. The font is a clear, legible sans-serif like Roboto, sized appropriately for easy reading on various devices. Restaurant names are displayed prominently in a bold, slightly larger font size (perhaps 18px) in a dark gray (#333333). Below each restaurant name, the address is shown in a smaller, regular weight font (14px) in a lighter gray (#666666). A concise distance from the user’s location (e.g., “0.5 miles”) is displayed next to the address. Restaurant ratings (out of 5 stars) are visually prominent using a standardized star rating system, and operating hours are clearly presented in a concise format (e.g., “Open until 10 PM”). A high-quality image of each restaurant’s food or storefront adds visual appeal, enhancing the user experience and encouraging clicks. A subtle, consistent color palette maintains a professional and user-friendly feel.
Data Integration and Processing
Data from multiple sources—such as Yelp, Google Places, and Foursquare—flows into a central processing unit. This unit uses APIs to access real-time data on restaurant locations, operating hours, menus, ratings, and user reviews. This raw data undergoes a series of transformations. Data cleansing removes inconsistencies and errors (e.g., correcting typos in addresses). Data standardization ensures consistent formats across different sources. Data enrichment adds valuable contextual information (e.g., cuisine type, price range) extracted from restaurant websites or menus. Finally, the processed data is stored in a database optimized for quick retrieval and display on the user interface. The entire process is designed to minimize latency, ensuring users receive up-to-date information quickly. A visual representation might show a flowchart, illustrating the flow of data from different sources, through the transformation steps, and finally to the database and user interface.
Location-Based Search During Travel
A user on a business trip in Chicago searches for “food near me that’s open” at 8 PM. Their mobile device’s location services pinpoint their precise location within a downtown hotel. The application integrates with a mapping service (e.g., Google Maps) to display nearby restaurants on an interactive map, color-coded to indicate proximity and operating hours. Restaurants open at that time are highlighted, and their distance from the user’s location is clearly indicated. The user can zoom in and out, pan across the map, and filter results by cuisine type, price range, or rating. The application leverages the device’s GPS capabilities to ensure accurate location detection and provide relevant search results in real-time, dynamically updating as the user’s location changes.
Handling Inaccurate Operating Hours
Let’s say a popular Italian restaurant, “Bella Notte,” shows operating hours of “11 AM – 10 PM” in our database, sourced from Google Places. However, a user checks the restaurant at 9 PM and finds it closed. The application receives user feedback indicating the inaccurate hours. This feedback triggers a flagging mechanism. The application then compares the reported closure time with the stored operating hours. If the discrepancy is significant and multiple users report the same issue, the application automatically reduces the confidence score associated with the restaurant’s operating hours. This lower confidence score leads to a more prominent warning message displayed next to the restaurant’s information on the search results page, informing users of the potential inaccuracy and encouraging them to confirm the hours independently before visiting. This approach combines user feedback with automated checks to ensure the database remains as accurate as possible.