Understanding User Intent
The seemingly simple phrase “food near my current location” hides a surprising depth of user intent. Understanding this nuance is crucial for businesses leveraging location-based services and optimizing their online presence to attract hungry customers. The query isn’t just about finding sustenance; it’s a window into a user’s immediate needs, desires, and time constraints.
A user searching for “food near my current location” could be driven by a wide spectrum of motivations, each impacting their expectations and preferences. Failing to account for this variety can lead to missed opportunities and frustrated users. Therefore, a deep dive into the potential meanings behind this seemingly straightforward request is essential for businesses in the food industry.
User Needs Implied by the Search Query
The user’s need is directly tied to their context and urgency. Are they desperately hungry and need a quick bite, or are they leisurely planning an evening meal? This dictates the type of establishment they’ll consider and their tolerance for wait times. For example, someone on a lunch break will prioritize speed and convenience, perhaps opting for a fast-food chain or a quick-service restaurant. Conversely, someone planning a romantic dinner will likely seek a more upscale and leisurely dining experience. This distinction is critical for targeted advertising and search engine optimization ().
Contextual Interpretations of the Search Query
The context in which the search is performed heavily influences its meaning. A search during lunchtime (12pm-2pm) suggests a need for a quick and efficient meal. A search in the evening (6pm-9pm) implies a more considered dining experience, possibly involving reservations. A late-night search (after 10pm) points to cravings and a desire for late-night options like pizza delivery or a 24-hour diner. These contextual clues are invaluable for businesses to tailor their marketing and optimize their online presence for peak demand periods.
Categorization Based on Urgency and Desired Food Type
We can categorize user intent based on two key factors: urgency and desired food type. High urgency searches often involve s like “fast,” “quick,” or “nearby.” These users need immediate gratification. Low urgency searches might involve more specific cuisine preferences or price points. For instance, someone searching “Italian food near me” exhibits a lower urgency, as they’re more focused on a specific type of cuisine. They’re likely planning ahead and have more time to consider options. The type of food desired also significantly influences the user’s expectations. A search for “pizza near me” suggests a different need than a search for “healthy food near me.”
Examples of User Intent Categories
Food near my current location – The following table illustrates different user intent categories based on urgency and food type:
Urgency | Food Type | Example Search Query | Implied User Need |
---|---|---|---|
High | Fast Food | “fast food near me” | Quick, inexpensive meal; limited time |
High | Any Cuisine | “food near me now” | Immediate hunger; convenience is paramount |
Low | Specific Cuisine | “Thai food near me” | Specific craving; willing to spend more time finding the right place |
Low | Fine Dining | “best restaurants near me” | Special occasion; prioritizing quality and ambiance |
Data Sources and Information Retrieval
:no_upscale()/cdn.vox-cdn.com/uploads/chorus_image/image/62457776/Nobu.0.0.0.0.0.jpg)
Finding the best food near you requires a robust system for gathering and processing information. This involves identifying relevant data points, sourcing them from reputable online platforms, and then integrating that data to provide a comprehensive and accurate result. The success of any location-based food recommendation system hinges on the quality and completeness of its underlying data.
The types of data necessary to effectively fulfill a “food near me” query are multifaceted and require a comprehensive approach. A simple list of restaurants wouldn’t suffice; users expect richer information to make informed decisions.
Data Requirements for Food Location Searches
To deliver a truly valuable service, the system needs to collect several key data points for each restaurant. These include the restaurant’s name, full address (including latitude and longitude for precise location mapping), a detailed menu (ideally with pricing), user ratings and reviews (from multiple sources), operating hours (including any variations for days of the week or special events), cuisine type(s) offered, and price range (e.g., $, $$, $$$). Additionally, incorporating images of the restaurant and its food significantly enhances the user experience.
Potential Data Sources, Food near my current location
Several online platforms offer rich datasets relevant to this query. Google Maps and other mapping services provide location data, addresses, operating hours, and often user ratings. Restaurant review sites like Yelp, TripAdvisor, and Zomato are treasure troves of user reviews, ratings, menus, and photos. Business directories such as Yellow Pages and local government websites can offer supplementary information, though their data might be less comprehensive or up-to-date. Finally, direct integration with restaurant websites, where available, can provide the most accurate and current menu information.
Data Accuracy and Completeness
The accuracy and completeness of data vary significantly across different sources. While Google Maps generally provides reliable location and operating hours data, the detail and frequency of updates can fluctuate. Review sites like Yelp offer abundant user-generated content, but the reliability of reviews can be subjective and potentially influenced by factors such as incentivized reviews or biased opinions. Business directories often lag behind in updating information, leading to potential inaccuracies regarding operating hours or even whether a restaurant is still in business. Directly accessing restaurant websites provides the most accurate and up-to-date menu information, but this requires significant technical effort to integrate diverse website structures. A successful system must account for these inconsistencies and implement strategies for data validation and reconciliation.
Hypothetical Data Pipeline
A robust data pipeline would involve several stages. First, data is collected from multiple sources using APIs or web scraping techniques. Data cleaning and standardization are crucial steps to ensure consistency in formatting and data types. Next, data from different sources is matched and merged based on restaurant name and address. Conflicting information (e.g., differing operating hours) needs to be resolved using a weighted averaging approach, prioritizing more reliable sources. Finally, the integrated data is stored in a database optimized for fast retrieval and querying, allowing for efficient response to user location-based searches. This pipeline would continuously monitor data sources for updates and incorporate mechanisms for detecting and handling inconsistencies. For example, if a restaurant’s operating hours change on Google Maps, the system should automatically update its database to reflect the change. This continuous data refresh ensures the information presented to users is as current and accurate as possible.
Presentation of Results: Food Near My Current Location
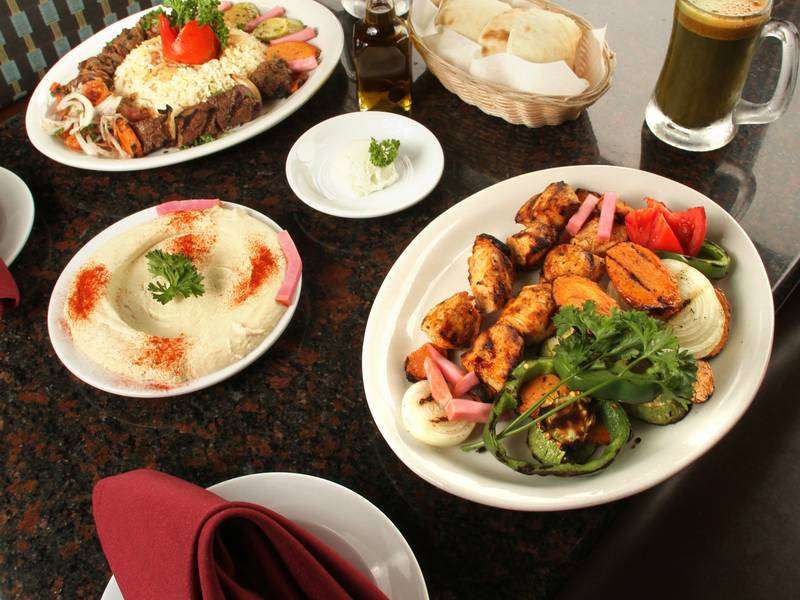
The presentation of search results is crucial for user experience and conversion. A poorly designed interface, regardless of how accurate your data is, will drive users away. We need to present restaurant information clearly, concisely, and in a visually appealing manner, optimizing for speed and ease of understanding. This ensures users find what they need quickly and efficiently, increasing the likelihood of them choosing a restaurant and making a reservation or placing an order.
Effective presentation involves selecting the right format, prioritizing relevant information, and incorporating visual cues to enhance readability and engagement. Consider the user’s journey and aim to minimize friction at every step.
Restaurant Information Table
A well-structured table is an excellent way to present key restaurant details. This allows for a quick comparison of multiple options. Responsive design ensures readability across different devices.
Restaurant Name | Address | Cuisine | Rating |
---|---|---|---|
The Italian Place | 123 Main Street, Anytown | Italian | 4.5 stars |
Spicy Sichuan | 456 Oak Avenue, Anytown | Sichuan | 4.0 stars |
Burger Bliss | 789 Pine Lane, Anytown | American | 3.8 stars |
Sushi Sensations | 101 Maple Drive, Anytown | Japanese | 4.2 stars |
Alternative Presentation Formats
Tables aren’t the only option. Alternative formats cater to different user preferences and offer diverse ways to explore results.
A list with brief descriptions, for instance, could highlight key features like “Upscale Italian with outdoor seating” or “Casual Mexican, great for families.” This provides a quick overview, allowing users to scan and select based on initial interest. A map with markers, showing restaurant locations, is particularly useful for users prioritizing proximity. Imagine a Google Maps style interface with color-coded pins representing different cuisines or price ranges.
Prioritization and Filtering of Results
Prioritizing and filtering results based on user preferences is critical for providing a relevant and personalized experience. This dramatically improves user satisfaction and reduces search time.
Filtering options should include distance from the user’s location (e.g., within 5 miles, 10 miles), cuisine type (e.g., Italian, Mexican, Chinese), price range (e.g., $, $$, $$$), and possibly even dietary restrictions (vegetarian, vegan, gluten-free). Prioritization could be based on a combination of factors, such as rating, proximity, and user reviews. For example, restaurants with higher ratings and closer proximity could be displayed first.
Visually Appealing Presentation
Visual elements significantly impact user engagement. High-quality images of restaurant exteriors and signature dishes are highly effective. Consider using star ratings for quick assessment of popularity. Color-coding could be used to represent price ranges (e.g., green for $, yellow for $$, red for $$$). Clear and concise typography, ample white space, and a consistent design language are essential for a polished and professional look. A well-designed layout improves readability and makes the information easily digestible. Think of the visual appeal of sites like Yelp or TripAdvisor; they are designed to inspire and inform.
Handling Ambiguity and User Preferences
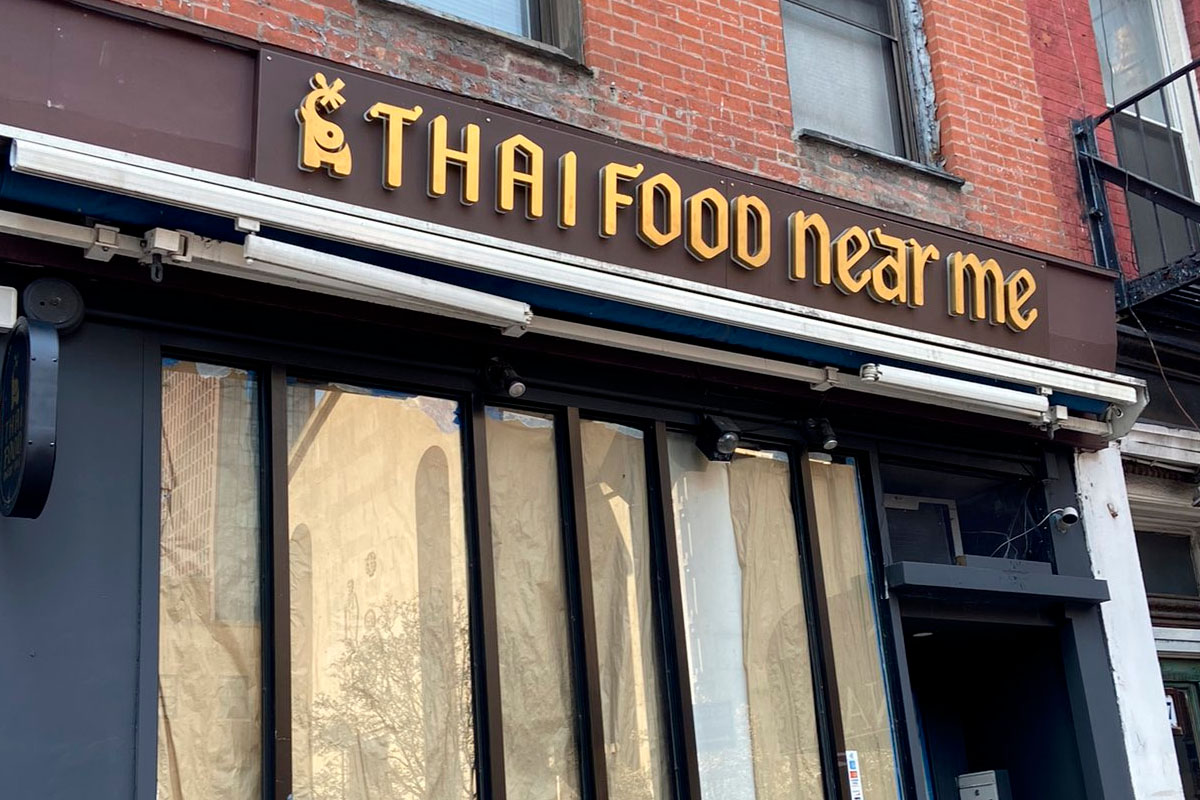
Finding the perfect meal near you shouldn’t be a culinary quest riddled with frustration. A robust food discovery app needs to gracefully handle ambiguous location data and cater to diverse user preferences to deliver truly relevant results. This requires sophisticated techniques beyond simple matching.
Ambiguous location data and unavailable user location information present significant challenges. Without precise location data, the system must intelligently infer user intent. For example, if a user searches for “best pizza,” without specifying a location, the system could default to the user’s last known location (if available and consented to), or present a prompt to refine the search geographically, perhaps through an interactive map or address entry. Another strategy involves leveraging IP geolocation, though this is less precise and prone to error. However, combining IP geolocation with other signals, such as the user’s device settings, can improve accuracy. Furthermore, understanding the context of the search query can be helpful. For instance, a search for “pizza near the airport” strongly suggests a location preference, even if the user hasn’t explicitly stated their current location.
Handling Ambiguous Locations
Addressing ambiguous locations requires a multi-pronged approach. Firstly, the system should prioritize using the most precise location data available. If GPS coordinates are available, they should be used. If not, the system should fall back to IP geolocation, but with a clear understanding of its limitations. Secondly, the application should offer clear prompts to the user to clarify their location if the system detects ambiguity. This could involve suggesting nearby landmarks or allowing users to manually input an address or zip code. Finally, the system should employ robust error handling to gracefully manage situations where no location data is available. In such cases, it might default to a broader geographic area or prompt the user to provide a location.
Refining Search Criteria
Allowing users to refine their search is crucial for delivering personalized results. This can be achieved by providing various filtering options. For example, users should be able to specify cuisine type (e.g., Italian, Mexican, Indian), price range (e.g., $, $$, $$$), dietary restrictions (e.g., vegetarian, vegan, gluten-free), and even ambiance (e.g., casual, fine dining). Implementing these filters requires a well-structured database that allows for efficient querying and filtering. A robust search system should also allow for the combination of these filters to enable granular control over search results. For instance, a user might search for “vegetarian Indian restaurants under $20 near me.”
Personalizing Search Results
Personalization significantly enhances user experience. This can be achieved by leveraging user history and preferences. For example, if a user frequently orders Thai food, the system can prioritize Thai restaurants in future searches. Similarly, if a user has previously rated a restaurant highly, that restaurant should be prominently featured in subsequent searches. This requires a sophisticated recommendation engine that analyzes user behavior and preferences. Furthermore, the system can also leverage machine learning algorithms to predict user preferences based on their past behavior and demographic data. For instance, if a user is consistently ordering healthier options, the system could proactively suggest similar restaurants in the future. This proactive approach ensures that users are consistently presented with options tailored to their needs and preferences.
Addressing Data Errors and Inconsistencies
Data quality is paramount. Inaccurate or inconsistent data can lead to irrelevant or misleading search results. To mitigate this, a robust data validation process is essential. This involves regular data cleaning and updates, ensuring data consistency across various sources, and implementing mechanisms to detect and correct errors. This might involve employing data quality tools, regular manual reviews of the data, and incorporating feedback mechanisms to allow users to report errors or inconsistencies. For example, if a restaurant’s hours are incorrect, a user could report it, and the system could flag the data for review and correction. Moreover, implementing data redundancy and backup mechanisms ensures that data loss does not significantly impact the system’s functionality.
Advanced Features and Considerations
Building a truly exceptional food-finding app requires more than just basic location-based search. To stand out, you need to incorporate advanced features that deliver a seamless and personalized user experience, driving engagement and repeat usage. This means moving beyond simple listings and into the realm of real-time data, user-generated content, and robust data management.
Real-time features significantly enhance the user experience. Imagine searching for a nearby restaurant only to discover its current wait time, the availability of tables, and even the option to order online directly through the app. This proactive information empowers users to make informed decisions, saving them valuable time and frustration. Integrating such features requires partnerships with restaurants, using APIs to access their point-of-sale systems or dedicated waitlist management tools.
Real-time Data Integration
The integration of real-time data, such as wait times and availability, requires a multi-faceted approach. Firstly, secure partnerships with restaurants are crucial. This involves negotiating data sharing agreements, ensuring data accuracy and consistency. Secondly, a robust technical infrastructure is needed to handle the real-time data streams. This could involve using a message queue system like Kafka or RabbitMQ to handle the high volume of updates and ensure low latency. Finally, a user-friendly interface is essential to present this information clearly and concisely within the app, perhaps using color-coded indicators to visually represent wait times (e.g., green for short waits, red for long waits). For example, a restaurant showing a 30-minute wait time could be clearly indicated with a visual cue and a prominent “Check Wait Time” button that allows users to monitor updates. A restaurant with immediate availability could show a “Walk-in Available” tag.
User Reviews and Ratings Incorporation
User reviews and ratings are vital for building trust and providing valuable insights to potential customers. A well-designed system aggregates reviews from various sources, such as Yelp, Google Reviews, and even directly from the app itself. These reviews should be displayed prominently alongside restaurant listings, possibly using a star rating system and allowing users to filter results based on average rating. Furthermore, the system should moderate reviews to prevent spam or fake reviews, ensuring the integrity of the data. For example, a restaurant with a 4.5-star rating and numerous positive reviews about its excellent service and delicious food will attract more users than one with a lower rating and negative reviews.
Handling Diverse Restaurant Types
Adapting to the diverse landscape of food establishments is key. The system needs to seamlessly handle various restaurant types, including food trucks, pop-up restaurants, and home-based businesses. This requires flexible data models capable of storing different types of information, such as food truck locations (which may change frequently), pop-up restaurant dates and times, and the operating hours and contact information for home-based businesses. Geolocation data plays a critical role in locating food trucks and pop-up restaurants, and clear labeling is essential to distinguish these from traditional brick-and-mortar establishments. For instance, a map view could clearly identify food trucks with a distinct icon, while pop-up restaurants could be marked with temporary event markers showing the duration of their operation.
Restaurant Information Management and Updates
Maintaining the accuracy and timeliness of restaurant information is paramount. This requires a system for managing restaurant profiles, allowing restaurants to update their information (hours, menus, contact details, etc.) directly through the app or a web portal. The system should also incorporate automated checks, perhaps by periodically pinging restaurant websites or using third-party data providers to verify the accuracy of information. A robust moderation process is needed to review and approve updates before they are reflected in the app. Regular data audits and automated alerts for outdated or inconsistent information will help maintain data integrity. This could include alerts for restaurants that haven’t updated their information in a certain period, allowing for proactive intervention and ensuring the accuracy of the information presented to users.