Understanding User Intent

Unlocking the true potential of a “good places for lunch near me” search requires a deep dive into user intent. Understanding what drives these searches is crucial for businesses aiming to capture this valuable traffic. We need to move beyond simple analysis and explore the nuanced desires and expectations behind each query.
The seemingly simple phrase “good places for lunch near me” masks a wide spectrum of individual needs and preferences. Users aren’t just looking for *any* lunch spot; they’re seeking an experience tailored to their specific circumstances and desires. This necessitates a granular understanding of their motivations.
Lunch Preference Diversity
Users searching for lunch options exhibit diverse preferences. These preferences often intersect and influence each other, creating a complex decision-making process. For instance, a business professional might prioritize speed and efficiency, while a family might focus on kid-friendly options and value. A tourist might prioritize unique local experiences. Understanding these varied motivations is key to effective targeting. A quick lunch for a busy professional is vastly different from a leisurely family brunch. The same can represent vastly different needs.
Factors Influencing Lunch Location Choice
Several key factors significantly impact a user’s choice of lunch location. These factors often interact, creating a layered decision-making process. Price is a major consideration; a budget-conscious individual will have very different options compared to someone with a more flexible budget. Cuisine type plays a crucial role; some might crave Italian, others Mexican, and others might be looking for a healthier, vegan option. The ambiance of the restaurant is also a significant factor; some prefer a quiet and intimate setting, while others prefer a lively and bustling atmosphere. Finally, proximity is paramount; users typically want options within a reasonable distance from their current location. This isn’t just about physical distance but also about accessibility – ease of parking, public transport access, etc.
User Persona: The Busy Professional
Let’s create a persona to illustrate this complexity. Meet Sarah, a 35-year-old marketing manager. She’s busy, time-constrained, and values efficiency. Her lunch break is typically an hour, and she needs a quick, healthy, and reasonably priced option. Sarah doesn’t want a long wait, prefers a place with decent Wi-Fi for catching up on emails, and appreciates a clean and professional atmosphere. She might be willing to pay a slightly higher price for convenience and quality, but budget is still a factor. Her ideal lunch spot is a cafe or quick-service restaurant with healthy options, fast service, and reliable Wi-Fi, located within a 10-minute walk from her office. Understanding Sarah’s needs – and those of countless others like her – allows businesses to tailor their offerings and marketing messages for maximum impact. This granular understanding is the key to success in this competitive landscape.
Data Sources and Information Gathering
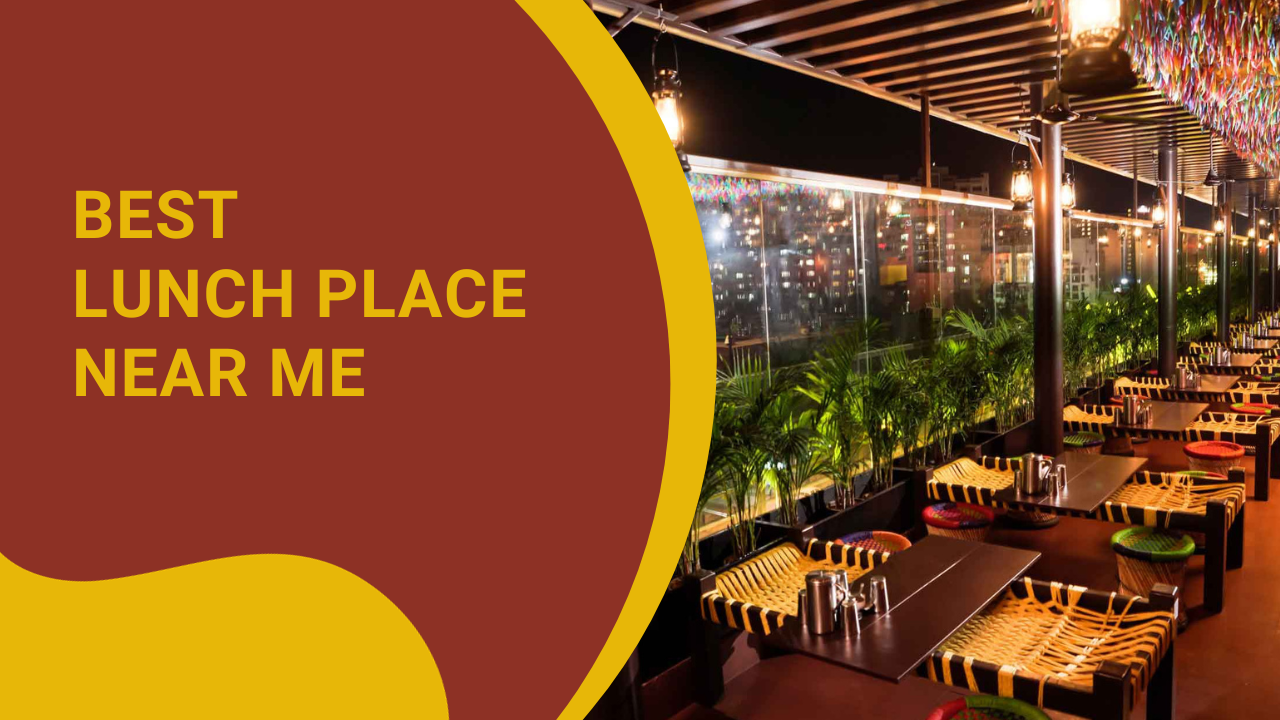
Finding the perfect lunch spot requires more than just a hunch; it demands a strategic approach to data collection. To build a truly effective lunch recommendation system, we need to leverage the wealth of information available online and offline. This involves identifying reliable sources, extracting key details, and structuring that data for insightful analysis. The process, while seemingly simple, can significantly impact the accuracy and usefulness of your final recommendations.
Good places for lunch near me – Effective data gathering is the cornerstone of any successful location-based service. Without a robust and reliable data pipeline, your recommendations will be flimsy at best and misleading at worst. The following sections detail the crucial data sources and the methods for extracting the most valuable information.
Potential Data Sources for Lunch Place Discovery
The digital landscape offers a plethora of resources for discovering lunch spots. These sources provide diverse perspectives and data points, allowing for a comprehensive understanding of each establishment. Utilizing a multi-source approach is key to minimizing bias and maximizing the accuracy of your recommendations.
- Online Review Platforms: Yelp, Google Maps, TripAdvisor, and Zomato are invaluable sources, offering user reviews, ratings, photos, and business details. These platforms provide a vast amount of user-generated content, reflecting real-world experiences.
- Social Media: Platforms like Instagram, Facebook, and TikTok can reveal hidden gems. Hashtags, location tagging, and user reviews offer insights into popular spots, often showcasing visual aspects like food presentation and ambiance.
- Business Directories: Sources such as Yellow Pages, Yelp’s business directory, and even Google My Business listings provide structured data including business hours, contact information, and sometimes menus. This information is crucial for verifying details and providing comprehensive information to users.
- Restaurant Websites: Many restaurants maintain their own websites, offering menus, pricing, hours of operation, and often high-quality images. This is a direct source of information, although it might be biased towards positive representation.
Methods for Extracting Relevant Information
Extracting relevant information requires a systematic approach to ensure consistency and accuracy. The goal is to capture key data points that directly impact user decisions regarding lunch choices.
- Location: Precise address, latitude and longitude coordinates are essential for mapping and proximity-based recommendations. This information is readily available on most online platforms.
- Cuisine: Identifying the type of food served (e.g., Italian, Mexican, American) is crucial for filtering and personalization. This often requires careful analysis of menu descriptions and user reviews.
- Price: Determining the price range (e.g., $, $$, $$$) requires examining menus, online reviews mentioning price points, or using third-party price estimation tools. This helps users find places that fit their budget.
- Ratings: Aggregating ratings from multiple sources (Yelp, Google, etc.) provides a more robust and less biased overall rating. Consider weighting ratings based on the number of reviews to account for the volume of feedback.
- Reviews: Analyzing text reviews requires natural language processing (NLP) techniques to extract sentiments, common themes, and specific mentions of food quality, service, and ambiance. This qualitative data adds significant value to quantitative ratings.
Organizing Information into a Structured Format
Once the data is extracted, organizing it into a structured format is essential for analysis and efficient use. This involves defining a consistent schema to ensure data integrity and ease of processing.
Restaurant Name | Address | Latitude | Longitude | Cuisine | Price Range | Average Rating | Number of Reviews | Sample Reviews (Sentiment Analysis) |
---|---|---|---|---|---|---|---|---|
Example Restaurant 1 | 123 Main St | 34.0522 | -118.2437 | Italian | $$ | 4.2 | 250 | Positive: “Great pasta!”, Negative: “Slow service” |
Example Restaurant 2 | 456 Oak Ave | 34.0522 | -118.2437 | Mexican | $ | 3.8 | 100 | Positive: “Delicious tacos!”, Negative: “Small portions” |
Location-Based Filtering and Ranking
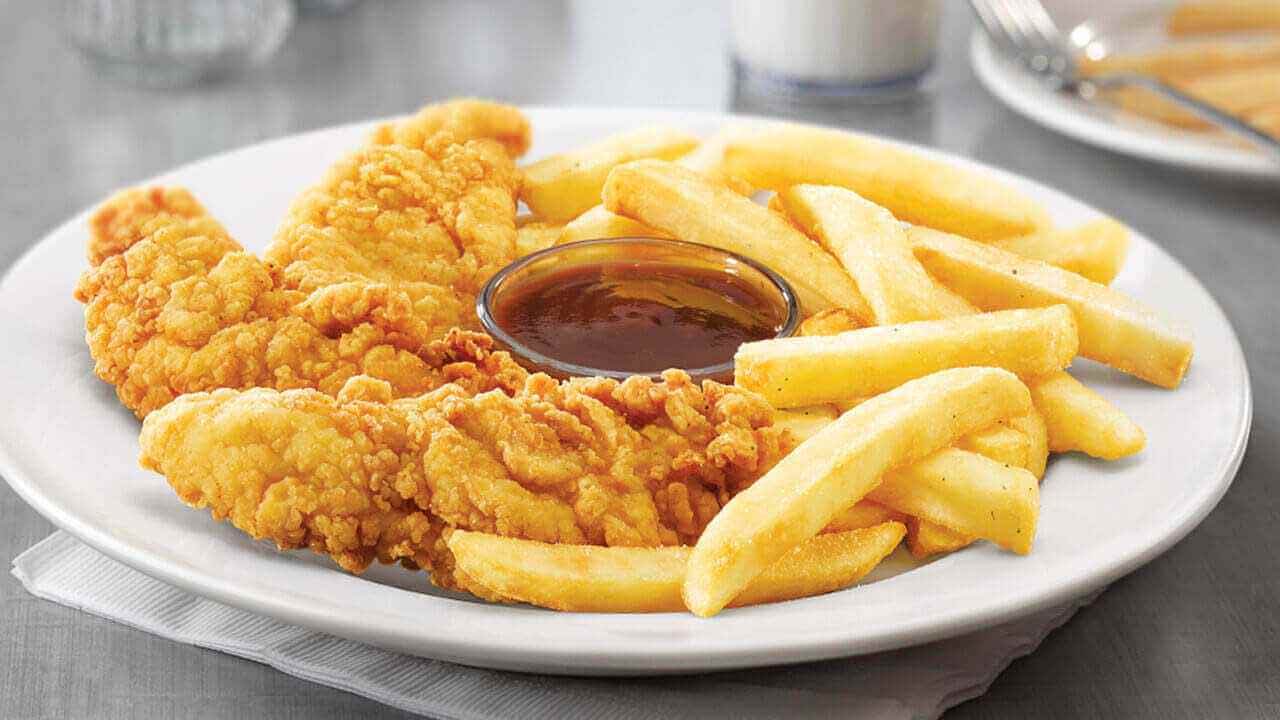
Finding the perfect lunch spot shouldn’t feel like searching for a needle in a haystack. Leveraging location data and smart ranking algorithms is key to delivering a seamless and satisfying user experience. This process transforms a potentially overwhelming task into a personalized and efficient lunch discovery.
Location-based filtering and ranking are crucial for any successful food discovery platform. By prioritizing relevant results based on proximity and user preferences, you drastically improve user engagement and satisfaction. This section details the precise mechanics of implementing this functionality.
Location Filtering Using GPS Coordinates or Address
To filter lunch places based on user location, we first need to obtain the user’s location. This can be achieved through several methods, including GPS coordinates obtained directly from the user’s device or by using an address provided by the user. Once obtained, we need to convert this location data into a consistent format, typically latitude and longitude coordinates. Then, we use a distance calculation formula, such as the Haversine formula, to determine the distance between the user’s location and each lunch place in our database. The Haversine formula accounts for the curvature of the Earth, providing a more accurate distance calculation compared to simpler methods. We can then filter the results to display only those lunch places within a specified radius of the user’s location. This radius can be a configurable parameter, allowing users to adjust their search area. For example, a user might choose a 5-mile radius, showing all restaurants within that range.
Ranking Lunch Places Based on Proximity, User Ratings, and Popularity
Ranking lunch places requires a multi-faceted approach, combining several factors to provide a truly relevant and personalized list. A weighted algorithm is ideal. This algorithm would assign weights to different factors based on their importance. For instance:
Proximity: This is a critical factor. The closer a restaurant is, the higher it should rank. We can use a simple inverse distance function: Rank = 1 / distance. This ensures that closer restaurants receive a higher rank.
User Ratings: Average user ratings are a strong indicator of quality. A higher average rating should result in a higher rank. We can directly use the average rating score.
Popularity: The number of reviews or visits a restaurant receives reflects its popularity. More popular places should rank higher. We can use a logarithmic function to scale the popularity score to prevent extremely popular restaurants from dominating the rankings. For example, Rank = log(number of reviews + 1). The “+1” prevents issues with restaurants having zero reviews.
The final rank for a restaurant is calculated by a weighted sum of these factors. For example:
Final Rank = (0.4 * Proximity Rank) + (0.3 * User Rating) + (0.3 * Popularity Rank)
The weights (0.4, 0.3, 0.3) can be adjusted based on user preferences or A/B testing to optimize the ranking algorithm.
Presenting Ranked Results in an HTML Table
The ranked results should be presented clearly and concisely. An HTML table is an excellent way to achieve this. The table should be responsive, adapting to different screen sizes. Below is an example of how the data could be displayed:
Name | Cuisine | Price Range | Rating |
---|---|---|---|
The Burger Joint | American | $ | 4.5 |
Luigi’s Pizzeria | Italian | $$ | 4.2 |
Sushi Sensations | Japanese | $$$ | 4.8 |
Spice Route | Indian | $$ | 4.0 |
Cuisine and Price Range Filtering
Implementing robust filtering options is crucial for any location-based service, especially one focused on finding lunch spots. Users need a quick and efficient way to narrow down their choices based on their preferences and budget. This section details how to implement cuisine and price range filtering, enhancing user experience and driving engagement.
Filtering by cuisine and price allows users to quickly find restaurants that match their specific desires. Imagine searching for lunch and having to wade through hundreds of irrelevant results – frustrating, right? Effective filtering transforms this overwhelming task into a streamlined, personalized experience. By offering clear and intuitive filter options, you empower users to take control of their search and discover the perfect lunch spot faster.
Cuisine Type Filtering Implementation
To implement cuisine type filtering, we can use a simple dropdown menu. This menu will list various cuisine types, allowing users to select one or more options. The backend system will then filter the restaurant database accordingly. For example, a user might select “Italian” and “Mexican,” resulting in a list of restaurants serving either or both cuisines. The dropdown menu provides a clean and organized way to present numerous options without overwhelming the user. Consider using a searchable dropdown for a better user experience if you have a large number of cuisine options.
Price Range Filtering Implementation
Price range filtering can be implemented using a series of radio buttons or a slider. Radio buttons allow users to select a single price range (e.g., $, $$, $$$), while a slider offers more granular control, allowing users to specify a minimum and maximum price. Both methods are effective, and the best choice depends on the specific design and user experience goals. A visual representation of the price ranges (e.g., using dollar signs) improves clarity and understanding.
User Interface Design
A well-designed user interface for these filters should be intuitive and visually appealing. Consider placing the filters prominently on the page, perhaps in a sidebar or at the top of the search results. The labels should be clear and concise (e.g., “Cuisine Type,” “Price Range”). The visual design should be consistent with the overall website design.
For example, a sample interface might include a dropdown menu for cuisine type with options such as “American,” “Italian,” “Mexican,” “Chinese,” “Indian,” etc. Next to it, a slider could allow users to select a price range, visually represented with dollar signs ($, $$, $$$) corresponding to price brackets. This setup is easy to understand and use.
Structured Data Representation
Representing these filter options in a structured data format, such as JSON, is essential for efficient data processing and integration with other systems.
For example, a JSON representation of filter selections might look like this:
“cuisine”: [“Italian”, “Mexican”],
“price_range”:
“min”: 10,
“max”: 25
This JSON object clearly represents the user’s selection of Italian and Mexican cuisine and a price range between $10 and $25. This structured format simplifies data handling and allows for easy integration with various backend systems and APIs. This structured data is crucial for efficient database querying and returning relevant results. A well-structured database, combined with efficient filtering, significantly reduces the response time for user queries.
Visual Representation of Results
Creating a compelling visual representation of nearby lunch spots is crucial for user engagement and effective information delivery. A well-designed map interface not only displays locations but also transforms raw data into an easily digestible and actionable format, significantly improving the user experience. Think of it as transforming a spreadsheet of addresses into a vibrant, interactive city guide.
The most effective way to present this information is through a map integrated directly into the search results. This allows users to quickly grasp the spatial relationships between different restaurants, identify clusters of options, and easily assess distances from their current location. Imagine a clean, modern map, perhaps using a familiar service like Google Maps, but customized for our specific needs.
Map Integration and Marker Implementation
The map should prominently display markers representing each restaurant. Each marker should be clearly identifiable, perhaps using a consistent design, such as a stylized fork and knife icon. The size and color of the marker could subtly reflect factors like popularity (larger, brighter for higher-rated restaurants) or even price range (larger markers for more expensive options, smaller for budget-friendly choices). Crucially, each marker should be clearly labeled with the restaurant’s name, making it instantly recognizable. Consider using a tooltip or pop-up box that appears when a user hovers over a marker, providing a brief preview of the restaurant’s name and rating.
Cuisine and Price Range Representation
Color-coding is an extremely effective way to categorize restaurants by cuisine type or price range. For example, Italian restaurants could be represented by red markers, Mexican by green, and so on. Similarly, price ranges could be indicated by the shade of the marker; a darker shade for more expensive restaurants and lighter shades for budget-friendly choices. This visual cue allows users to instantly filter their search based on preferred cuisine or budget, without needing to examine individual restaurant details. Consider a legend clearly explaining the color-coding scheme, making the system intuitive and user-friendly.
Interactive User Reviews
A key element to building trust and providing valuable information is incorporating user reviews. When a user clicks on a restaurant marker, a pop-up window should appear, displaying a concise summary of user reviews. This summary could include the average rating, a short snippet of positive and negative feedback, and perhaps even a link to view all reviews on a dedicated review platform. This interactive element adds depth and credibility to the results, empowering users to make informed decisions based on the experiences of others. For example, a pop-up could show “4.5 stars – Delicious pasta, but slow service,” providing a quick snapshot of the user experience.
Handling Missing or Inconsistent Data
Data quality is paramount when building a robust lunch recommendation system. Missing or inconsistent data can significantly skew results, leading to inaccurate suggestions and a frustrating user experience. Therefore, a robust strategy for handling these issues is crucial for delivering reliable and valuable information. This involves proactive data validation, cleaning, and imputation techniques to ensure the integrity of our dataset.
Data quality directly impacts the accuracy and reliability of our lunch recommendations. Incomplete addresses, missing ratings, or inconsistent pricing formats can lead to inaccurate filtering and sorting, ultimately delivering poor user experience. To mitigate this, we must implement a multi-pronged approach encompassing data validation, cleaning, and imputation strategies.
Missing Data Imputation, Good places for lunch near me
Missing data is a common challenge in any dataset. In our context, this could involve missing restaurant ratings, incomplete addresses, or the absence of price information. Simply ignoring these entries isn’t an option, as it would reduce the size of our usable dataset and potentially bias our results. Instead, we’ll employ imputation techniques. For missing ratings, we can use the average rating of similar restaurants or a more sophisticated approach like K-Nearest Neighbors (KNN) to predict a missing rating based on the ratings of similar establishments. For incomplete addresses, we might attempt to geocode partial addresses or use reverse geocoding techniques to find a precise location based on nearby landmarks. Missing price ranges can be estimated based on the average price range of restaurants with similar cuisines and locations. These techniques minimize data loss and maintain the integrity of our dataset.
Inconsistent Data Handling
Inconsistent data presents another significant hurdle. This could manifest as various formats for price ranges (e.g., “$10-15,” “10-15 USD,” or “$$”), inconsistent units of measurement, or conflicting reviews. Standardization is key. We’ll create a standardized format for price ranges, converting all entries into a consistent numerical representation (e.g., a minimum and maximum price in USD). We will also implement a system for detecting and handling conflicting reviews, perhaps by using sentiment analysis to identify and flag potentially unreliable reviews. Furthermore, we can leverage data cleaning techniques to correct formatting errors and inconsistencies in other fields like cuisine types, ensuring uniform data representation across the entire dataset.
Data Validation and Cleaning Plan
A robust data validation and cleaning plan is the cornerstone of accurate and reliable results. This involves multiple steps. First, we’ll implement automated checks during data ingestion to identify and flag missing values, inconsistencies in data formats, and outliers. These checks will utilize regular expressions to validate data formats and identify anomalies. Secondly, we’ll employ data cleaning scripts to standardize data formats, correct errors, and handle missing values using the imputation techniques described above. Finally, we’ll conduct manual review of a sample of the data to ensure the accuracy of our automated processes and identify any remaining issues that require manual correction. This multi-layered approach guarantees data quality and minimizes the risk of erroneous recommendations.
Additional Features and Enhancements: Good Places For Lunch Near Me
Transforming a simple lunch-spot finder into a truly indispensable tool requires strategic enhancement. We need to move beyond basic location and cuisine filtering to create a seamless, personalized, and engaging user experience that drives repeat visits and builds loyalty. This involves integrating several key features that leverage the power of real-time data and user interaction.
Integrating Real-Time Availability, Online Ordering, and Photo Galleries significantly improves the user experience. These features provide users with immediate, actionable information, transforming the app from a simple directory into a complete lunch solution.
Real-Time Availability and Online Ordering
Imagine effortlessly checking if your favorite Thai place has a table available before even leaving the office. Real-time availability, integrated directly into the app, eliminates the frustrating guesswork of calling ahead. Furthermore, integrating online ordering allows users to place their lunch order directly through the app, streamlining the entire process and saving valuable time. This feature could be implemented using APIs from various restaurant platforms or by partnering directly with restaurants. For example, a user could see that “Thai Basil” has a 30-minute wait but “Spicy Noodle House” has immediate seating, and order takeout from either directly within the app.
User Reviews and Ratings
User-generated content is invaluable. Incorporating a robust review and rating system allows users to share their experiences, shaping the perception of each lunch spot. This system should be designed to filter out fake reviews and promote authentic feedback. Features like photo uploads alongside reviews could further enhance the user experience, providing visual confirmation of the food quality and restaurant ambiance. Consider implementing a system similar to Yelp or Google Reviews, allowing users to rate on aspects like food quality, service, price, and ambiance. This creates a dynamic and evolving view of each establishment, allowing for more informed decision-making.
Personalized Results Based on User Behavior
Personalization is key to engagement. By tracking user behavior—past searches, orders, ratings, and reviews—the app can learn individual preferences and tailor search results accordingly. For instance, a user who frequently orders from Italian restaurants with a 4-star rating or higher will see those types of restaurants prioritized in their search results. This data-driven approach ensures that each user’s experience is unique and relevant to their specific tastes. This also allows for the implementation of targeted recommendations, suggesting new lunch spots based on past preferences. For example, if a user frequently orders from a specific cuisine, the app can suggest similar restaurants nearby or ones with special offers.