Understanding User Intent Behind “Good Restaurants Nearby”

The seemingly simple search phrase “good restaurants nearby” masks a surprising complexity of user needs and expectations. Understanding these nuances is crucial for businesses aiming to attract customers through online search and optimize their online presence. By dissecting the various factors influencing a user’s perception of “good,” we can better target marketing efforts and improve customer satisfaction.
The factors driving a user’s definition of a “good” restaurant are multifaceted and highly personalized. Price point, cuisine type, ambiance, reviews, and even proximity all play a significant role. A business lunch requires a vastly different restaurant experience than a romantic dinner, highlighting the contextual nature of the search.
Three Distinct User Needs Implied by the Search Phrase
The search “good restaurants nearby” often reflects one of three core user needs: convenience, specific culinary desires, or a desire for a particular dining experience. Understanding these distinct needs allows businesses to tailor their marketing and online presence accordingly.
- Convenience-focused search: This user prioritizes proximity and ease of access. They might be looking for a quick lunch break, a casual dinner after work, or a place to grab a bite before an event. Speed of service and ease of ordering are key factors.
- Cuisine-specific search: This user is looking for a particular type of food. They may be craving Italian, Thai, or Mexican cuisine and are using the search to find highly-rated options nearby. The user’s definition of “good” is heavily influenced by their specific culinary preferences.
- Experience-driven search: This user is looking for a particular dining experience, such as a romantic atmosphere, a family-friendly environment, or a lively social setting. Reviews mentioning ambiance, service quality, and overall atmosphere are critical for this user segment.
Factors Influencing a User’s Perception of “Good”
A user’s perception of “good” is subjective and shaped by a variety of factors. These factors interact to create a complex decision-making process that goes beyond simple proximity.
- Online Reviews and Ratings: Star ratings and reviews on platforms like Yelp, Google Maps, and TripAdvisor significantly influence a user’s decision. Positive reviews build trust and credibility, while negative reviews can deter potential customers.
- Price Range: Budget plays a crucial role. A user searching for “good restaurants nearby” may have a specific price range in mind, eliminating options that fall outside their budget.
- Cuisine Type and Dietary Restrictions: The type of food offered and the availability of vegetarian, vegan, or gluten-free options are essential considerations for many users.
- Ambiance and Atmosphere: The overall atmosphere of the restaurant—casual, formal, romantic, family-friendly—significantly impacts the user’s perception of “good.”
- Location and Accessibility: Proximity, parking availability, and accessibility for people with disabilities are important practical factors.
Three User Personas Representing Different Search Intentions
To illustrate these diverse needs, let’s create three user personas:
- Persona 1: The Busy Professional (Convenience-focused): Sarah, a 35-year-old marketing manager, searches “good restaurants nearby” during her lunch break. She needs a quick, affordable, and convenient option with high ratings and fast service. She prioritizes efficiency and value for money.
- Persona 2: The Foodie Explorer (Cuisine-specific): David, a 28-year-old software engineer, searches “good restaurants nearby” looking for authentic Thai food. He’s willing to travel a bit further for high-quality cuisine and is actively seeking recommendations based on specific dishes and authentic preparation methods. His definition of “good” is heavily influenced by culinary expertise and authenticity.
- Persona 3: The Romantic Couple (Experience-driven): Maria and John, a couple in their late 20s, search “good restaurants nearby” planning a special anniversary dinner. They prioritize ambiance, excellent service, and a memorable dining experience. Reviews mentioning romantic settings, exceptional food presentation, and attentive service are highly influential in their decision.
Data Sources for Restaurant Information

Finding the perfect restaurant can feel like navigating a culinary maze. To help you find your ideal dining experience, we rely on various online platforms that aggregate and present restaurant information. Understanding the strengths and weaknesses of these data sources is crucial for making informed decisions. This section will compare and contrast three major players – Yelp, Google Maps, and TripAdvisor – analyzing their data collection methods, potential biases, and how user reviews shape their rankings.
Yelp, Google Maps, and TripAdvisor represent distinct approaches to compiling and presenting restaurant data. While all three platforms offer user reviews, ratings, and basic restaurant information, their algorithms, user bases, and overall focus differ significantly, leading to variations in how restaurants are presented and ranked.
Yelp’s Data Collection and Ranking System, Good restaurants nearby
Yelp primarily relies on user-generated content. Users submit reviews, ratings, and photos, which contribute significantly to a restaurant’s overall score and ranking. Yelp’s algorithm considers factors like the number of reviews, the average rating, the recency of reviews, and the consistency of positive and negative feedback. However, Yelp’s algorithm is notoriously opaque, making it difficult to understand precisely how a restaurant’s ranking is determined. This lack of transparency can lead to concerns about manipulation, especially given past controversies surrounding Yelp’s review filtering practices. A potential bias exists towards businesses that actively engage with their Yelp profiles and encourage reviews, potentially favoring larger chains over smaller, independent restaurants. Furthermore, the user base itself introduces a bias; Yelp’s demographic skews towards a specific age range and tech-savviness, potentially impacting the representation of diverse culinary preferences.
Google Maps’ Data Collection and Ranking System
Google Maps utilizes a multifaceted approach to restaurant data. It incorporates user reviews, but also integrates data from various other sources, including its own Google My Business platform, third-party data providers, and its own search algorithms. This integration allows for a broader and potentially more comprehensive dataset. Google’s ranking algorithm is also less transparent than Yelp’s, but generally emphasizes factors like relevance, prominence, and user engagement. Google Maps tends to prioritize businesses with complete and accurate information on their Google My Business profiles, potentially favoring established restaurants with robust online presence. The bias here might lie in favoring larger, well-established businesses that have the resources to optimize their Google My Business profiles. Google’s vast user base helps mitigate the risk of demographic bias, but geographic variations in user density could still impact local restaurant rankings.
TripAdvisor’s Data Collection and Ranking System
TripAdvisor relies heavily on user reviews and ratings, similar to Yelp. However, TripAdvisor focuses more on the overall travel experience, including accommodations, attractions, and restaurants. Its ranking algorithm considers factors like the number of reviews, the average rating, and the recency of reviews. Like Yelp, TripAdvisor’s algorithm is proprietary and not fully transparent. A potential bias exists towards popular tourist destinations and well-known establishments, as these tend to receive more reviews and higher visibility. TripAdvisor’s user base is largely composed of travelers, introducing a bias towards restaurants catering to tourists rather than local residents. This might overlook hidden gems that are beloved by locals but less known to tourists. Furthermore, the emphasis on overall travel experience can sometimes overshadow the purely culinary aspects of a restaurant.
Analyzing Restaurant Attributes
Understanding what drives user choices when searching for “good restaurants nearby” is crucial for optimizing your restaurant’s online presence. By analyzing key attributes, we can build a more effective search and recommendation system, ultimately driving more customers to your establishment. This involves understanding the specific details users prioritize when making their dining decisions.
Good restaurants nearby – Users don’t just search for “restaurants”; they’re looking for a specific experience. To effectively cater to this need, we must dissect the various factors influencing their decisions. This involves going beyond simple location-based searches and delving into the specific preferences and expectations users have when choosing a place to eat.
Key Restaurant Attributes and Visual Presentation
Five key attributes consistently emerge as critical factors in user restaurant evaluations: cuisine type, price range, ambiance, location, and reviews. Effectively presenting these attributes visually significantly improves user experience and drives informed decisions. The following table Artikels these attributes and suggests visual representations for optimal user engagement.
Attribute | Description | Visual Presentation Example | Implementation Notes |
---|---|---|---|
Cuisine | Type of food served (e.g., Italian, Mexican, Thai). | Use high-quality images of signature dishes. Include small icons representing relevant cuisines. For example, a small pizza icon for Italian, a taco icon for Mexican. Clearly label each cuisine type in a visually appealing font. | Ensure images are high-resolution and accurately reflect the food quality. Use consistent iconography for clear identification. |
Price Range | Cost of meals (e.g., $, $$, $$$). | Use a simple dollar sign system ($, $$, $$$) or a visual price slider indicating price points. Consider adding average price ranges (e.g., $10-20, $20-30, $30+). | Maintain consistency in dollar sign usage. Clearly define what each dollar sign represents in terms of price. A slider offers a more granular and interactive experience. |
Ambiance | Atmosphere and overall feel (e.g., casual, romantic, upscale). | Use evocative imagery. For a romantic ambiance, show a picture of candlelit tables. For a casual setting, depict a lively, friendly environment. Use descriptive words like “cozy,” “modern,” or “upscale.” | Images should accurately reflect the ambiance. Use descriptive words that evoke the desired feeling. |
Location | Restaurant’s geographical location and accessibility. | Integrate a map showing the restaurant’s precise location. Include clear directions and distance from the user’s current location. Display nearby landmarks for better context. | Use a well-known mapping service for accurate and reliable location data. Clearly display distance in a user-friendly format (e.g., miles or kilometers). |
Reviews | User ratings and comments. | Display an aggregated star rating prominently. Show a snippet of recent positive reviews. Consider incorporating a review count for credibility. | Use a clear and concise star rating system. Highlight positive reviews to showcase the restaurant’s strengths. Display the total number of reviews. |
Visualizing Restaurant Recommendations
Data is only powerful when it’s easily digestible. Raw restaurant information – addresses, cuisines, ratings – is useless unless presented in a way that helps users quickly make decisions. Visualizing this data is key to a successful restaurant recommendation system, transforming complex information into an intuitive and engaging experience. This involves strategic use of maps, imagery, and clear visual representations of key attributes.
Effective visualization translates directly to higher user engagement and ultimately, more restaurant bookings. A well-designed interface provides at-a-glance information, allowing users to quickly filter and compare options without sifting through endless lists. This section details how to achieve this through smart design and strategic visual cues.
Restaurant Recommendation Layout
The layout should prioritize ease of navigation and information clarity. A responsive design is crucial for optimal viewing across all devices. The following HTML snippet demonstrates a sample layout, incorporating a map, restaurant images, and key attributes. Remember, this is a simplified example; real-world implementations would require more robust styling and data integration.
<div class="restaurant-card">
<div class="restaurant-image">
<img src="restaurant-image.jpg" alt="Restaurant Image">
<div>
<div class="restaurant-info">
<h3>Restaurant Name</h3>
<div class="restaurant-rating">
<span class="stars">★★★★☆</span>
<span class="review-count">(123 reviews)</span>
<div>
<p class="restaurant-cuisine">Cuisine Type</p>
<p class="restaurant-price">Price Range: $$</p>
<p class="restaurant-address">Address</p>
<div>
</div>
<div id="map"></div>
The restaurant-card
div acts as a container. Each card displays an image, the restaurant name, a star rating, review count, cuisine type, price range, and address. The id="map"
div would dynamically load a map using a mapping API like Google Maps, centering on the restaurant’s location. Responsive design is achieved using CSS media queries, ensuring the layout adapts gracefully to different screen sizes.
Visual Representation of Restaurant Ratings
A clear and concise rating system is essential. The combination of a star rating and a summary of review sentiment provides a holistic view of customer experiences. For example, a restaurant might have a 4.5-star rating, but the sentiment summary could highlight predominantly positive reviews focusing on the quality of food, balanced by a few negative comments about slow service. This nuanced approach provides a more comprehensive picture than just a numerical rating.
Consider using different colors to represent the sentiment: green for positive, yellow for neutral, and red for negative. This visual cue immediately communicates the overall tone of reviews without the user needing to read individual comments. For instance, a predominantly green bar chart alongside the star rating would visually reinforce the positive sentiment.
Color-Coding Key Attributes
Color-coding can significantly improve the user experience by quickly highlighting key attributes like price range and cuisine type. Consistent color schemes are essential. For instance, you might use shades of green for affordable options, progressing to orange and red for more expensive choices. Similarly, you could assign specific colors to each cuisine type, making it easy to identify restaurants serving a particular type of food. For example, Italian might be represented by a deep red, while Mexican cuisine could be a vibrant yellow-orange. This ensures visual consistency and aids quick identification.
Think of it like a visual heatmap: users can instantly identify restaurants that fit their budget and culinary preferences based on color alone. This reduces cognitive load and allows users to quickly scan and compare options, enhancing overall efficiency and user satisfaction.
Handling Location-Based Queries
Accurately determining a user’s location is paramount for any location-based service, especially one recommending nearby restaurants. Inaccurate location data leads to irrelevant recommendations and a frustrating user experience – a recipe for disaster in the competitive online food scene. Getting this right is crucial for user engagement and retention.
Precise location handling involves gracefully managing various user inputs and leveraging multiple technologies to pinpoint their location as accurately as possible. This involves understanding the nuances of different location data formats and choosing the best approach depending on user input and available technologies.
User Location Input Variations
Handling diverse location inputs requires robust parsing and geocoding capabilities. Users might provide a zip code, a full street address, or even a less precise landmark. The system needs to intelligently interpret these variations and convert them into geographic coordinates (latitude and longitude). For example, a zip code like “90210” needs to be translated into a specific geographic area, while a landmark like “Times Square” requires reverse geocoding to identify its coordinates. A full address, such as “1600 Amphitheatre Parkway, Mountain View, CA 94043,” provides the most precise location information and is often the easiest to process. The system should prioritize accuracy and handle ambiguous inputs gracefully, perhaps prompting the user for clarification if necessary.
Determining User’s Current Location
Several technologies can be used to determine a user’s current location, each with its strengths and weaknesses. The most common are GPS (Global Positioning System), IP address geolocation, and user-provided location. GPS, when enabled, provides the most accurate location data. However, it relies on a clear view of the sky and can be inaccurate indoors or in areas with poor signal reception. IP address geolocation uses the user’s IP address to estimate their location. This is less precise than GPS but works even without GPS enabled. It’s important to note that IP geolocation is only approximate and may be inaccurate, especially for mobile users with dynamic IP addresses. Finally, users can manually provide their location, which might be the most reliable option if the other methods fail or provide inaccurate results. A well-designed system should seamlessly combine these methods, prioritizing accuracy and gracefully handling cases where some methods are unavailable or unreliable. For instance, if GPS is unavailable, the system might fall back to IP geolocation and then to a prompt asking the user to manually enter their location.
Calculating Distances Between Locations
Once the user’s location and the locations of nearby restaurants are known (represented as latitude and longitude coordinates), the distance between them needs to be calculated. The most common method is using the Haversine formula, which accounts for the curvature of the Earth. The Haversine formula provides a more accurate distance calculation than simpler methods that assume a flat Earth, especially for longer distances.
The Haversine formula: a = sin²(Δφ/2) + cos φ1 ⋅ cos φ2 ⋅ sin²(Δλ/2)
c = 2 ⋅ atan2( √a, √(1−a) )
d = R ⋅ c
where φ is latitude, λ is longitude, R is Earth’s radius (mean radius = 6,371km); note that angles need to be in radians.
This formula allows the system to efficiently calculate the distance between the user’s location and each restaurant, enabling the ranking of restaurants based on proximity. Optimizing this calculation is crucial for performance, especially when dealing with a large number of restaurants. Consider using efficient data structures and algorithms to minimize computation time. For example, spatial indexing techniques can significantly speed up the process of finding nearby restaurants.
Filtering and Sorting Restaurant Results
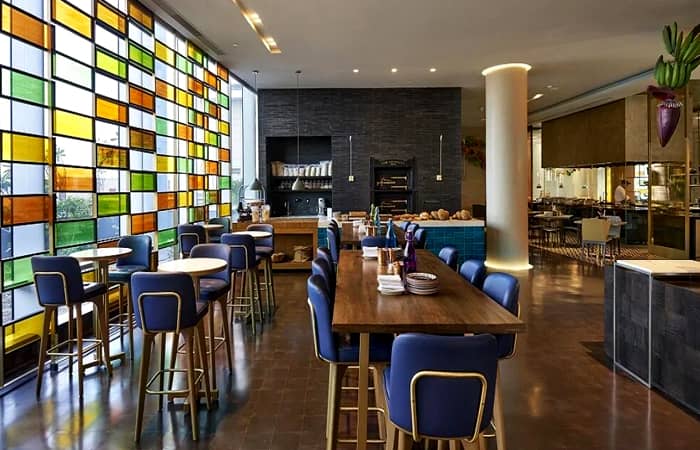
Finding the perfect restaurant amidst a sea of options requires a powerful and intuitive filtering and sorting mechanism. This is crucial for user experience, driving engagement and ultimately, conversions – whether that’s a reservation or a direct order. A well-designed system allows users to quickly narrow down choices based on their preferences, making the search process efficient and enjoyable. This section details the implementation of such a system.
Effective filtering and sorting are the backbone of a successful restaurant recommendation system. By enabling users to refine their search based on specific criteria, you significantly improve the chances of them finding a restaurant that perfectly matches their needs. This leads to higher user satisfaction and increased engagement with your platform.
Cuisine Type Filtering
Implementing cuisine type filtering involves creating a list of common cuisine types (e.g., Italian, Mexican, Chinese, Indian, etc.) and allowing users to select one or more options. This can be achieved using checkboxes or a multi-select dropdown menu. Backend processing then filters the restaurant database to return only those establishments matching the selected cuisines. For example, if a user selects “Italian” and “Seafood,” the system will only display restaurants offering both cuisines.
Price Range Filtering
Price range filtering allows users to specify a budget. This is usually implemented using a slider or a dropdown menu with predefined price ranges (e.g., $, $$, $$$). The backend filters the restaurant data based on the selected price range, considering factors like average meal cost or price points mentioned in reviews. A visual representation of the price range, such as dollar signs, provides immediate clarity to the user.
Other Attribute Filtering
Beyond cuisine and price, users might want to filter by other attributes. These could include dietary restrictions (vegetarian, vegan, gluten-free), ambiance (casual, fine dining), amenities (outdoor seating, Wi-Fi), or even ratings. Each attribute requires a corresponding filtering mechanism, typically checkboxes or dropdown menus, depending on the nature of the attribute. The backend logic then combines these filters to provide a highly refined set of restaurant recommendations.
Sorting Restaurant Results
Once filtered, the results need to be sorted for optimal presentation. Common sorting criteria include rating (highest to lowest), distance (nearest to farthest), and popularity (most reviewed or highest average rating). These sorting options should be clearly presented to the user, typically as a dropdown menu. The backend uses the selected sorting criterion to order the filtered restaurant data.
User Interface Elements for Filtering and Sorting
The following HTML snippets illustrate basic user interface elements for filtering and sorting. Note that this is a simplified example and would need to be integrated with a backend system for dynamic data retrieval and processing.
Consider the following HTML example for a simple filter:
<label for="cuisine">Cuisine:</label>
<select id="cuisine" name="cuisine">
<option value="all">All</option>
<option value="italian">Italian</option>
<option value="mexican">Mexican</option>
</select>
And a similar example for sorting:
<label for="sort">Sort By:</label>
<select id="sort" name="sort">
<option value="rating">Rating</option>
<option value="distance">Distance</option>
</select>
Presenting Diverse Restaurant Options: Good Restaurants Nearby
Showing a diverse range of restaurants is crucial for providing users with a truly representative selection and avoiding algorithmic bias. A system that only prioritizes popular chains overlooks hidden gems and unique culinary experiences, ultimately failing to meet the needs of a diverse user base. This section Artikels strategies to ensure your restaurant recommendations are inclusive and reflect the rich tapestry of dining options available.
Presenting a diverse selection goes beyond simply listing restaurants; it requires strategic planning and implementation. We need to actively combat biases inherent in data, like popularity metrics that favor established businesses over newcomers. By actively incorporating strategies to highlight underrepresented restaurants and emphasizing unique qualities, we create a more equitable and engaging user experience.
Highlighting Less Popular, Highly-Rated Restaurants
Many highly-rated restaurants remain relatively unknown due to limited marketing budgets or location. To surface these hidden gems, we need to move beyond simple popularity rankings. Implementing a weighted scoring system that balances popularity with ratings and reviews can effectively prioritize quality over sheer volume of reviews. For instance, a restaurant with fewer reviews but consistently high ratings (4.8 stars out of 5, with 20 reviews) could be ranked higher than a more popular restaurant (4.0 stars out of 5, with 500 reviews) that has a lower average rating. This prioritizes quality and diversity, giving users a wider range of options to explore.
Utilizing Visual Cues to Showcase Unique Restaurant Features
Visual elements are powerful tools for communicating information quickly and effectively. Using carefully chosen imagery and icons can significantly enhance the user experience by highlighting unique features. For example:
- A small icon depicting a vegetarian symbol next to vegetarian-friendly restaurants.
- A picture of a cozy fireplace for restaurants with a romantic ambiance.
- An image of a bustling outdoor patio for restaurants with outdoor seating.
- A graphic representing a family-friendly environment, suitable for restaurants that cater to families.
These visual cues allow users to quickly filter options based on their preferences without having to read lengthy descriptions. Think of it as a visual shorthand, making the search process more efficient and enjoyable. Imagine a user looking for a romantic Italian restaurant; a picture of a candlelit table immediately communicates the ambiance, enhancing the user’s decision-making process. The key is to use relevant and high-quality images that accurately reflect the restaurant’s atmosphere and offerings.
Prioritizing Restaurant Diversity Based on Cuisine Type and Price Range
To further enhance diversity, actively categorize restaurants based on cuisine type and price range. This allows users to easily filter results based on their preferences and budget constraints. For instance, instead of only showing the top 10 results based on a generic popularity algorithm, present a curated selection showcasing diverse cuisines, such as Italian, Mexican, Indian, Thai, and Ethiopian restaurants. Similarly, separate the results by price range (e.g., $, $$, $$$) to provide options for users with different budgets. This layered approach ensures a more representative and inclusive selection.