Understanding User Intent Behind “Recommended Restaurants Near Me”
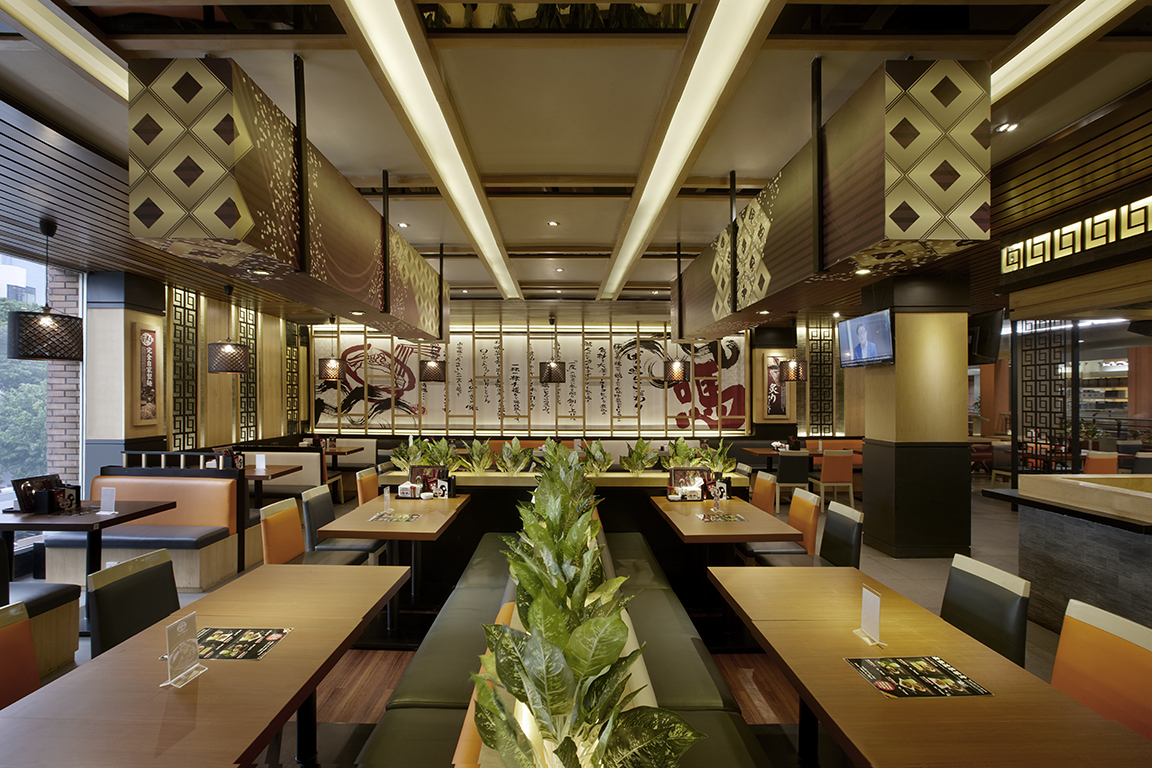
The search query “recommended restaurants near me” reveals a user’s immediate need for dining options in their current vicinity. This seemingly simple phrase, however, masks a wide range of underlying motivations and preferences, making it crucial to understand the nuances of user intent for effective search engine optimization and targeted recommendations. The user isn’t just looking for *any* restaurant; they’re seeking a specific dining experience tailored to their current situation and desires.
The user’s intent is driven by a combination of factors, including time constraints, budget, desired cuisine, and the overall dining experience they’re hoping for. Understanding these factors is key to providing truly valuable and relevant recommendations. Failure to do so results in a suboptimal user experience and lost opportunities for businesses.
Types of Restaurants Sought
Users searching for nearby restaurants aren’t looking for a monolithic category. Their needs are diverse, reflecting a spectrum of dining preferences and occasions. They might be craving a quick and affordable bite, a romantic dinner for two, a family-friendly establishment, or a sophisticated fine-dining experience. This diversity necessitates a granular understanding of different restaurant types and the specific needs they cater to. For instance, a user rushing for a quick lunch might seek fast food or a casual eatery, while someone celebrating an anniversary would prioritize a fine-dining establishment. The search query itself acts as a proxy for a much more complex decision-making process.
Factors Influencing Restaurant Choice Based on Location and Proximity
Location is paramount in this search. The “near me” qualifier explicitly indicates the user’s prioritization of convenience and minimal travel time. Several factors influence their choice based on this:
Recommended restaurants near me – Proximity significantly impacts the user’s decision. A restaurant located within walking distance or a short drive is highly preferable to one requiring a long commute, especially during peak hours or when time is limited. This is especially true for users who are pressed for time, or those without access to personal transportation.
Accessibility also plays a crucial role. Users consider factors like parking availability, ease of access for people with disabilities, and proximity to public transportation. A restaurant with ample parking might be preferred over one with limited or expensive parking options, even if the latter is slightly closer. Similarly, the availability of accessible entrances and restrooms is a major consideration for users with mobility challenges.
Furthermore, the perceived safety and overall ambiance of the surrounding area also influence the user’s choice. Users are more likely to choose a restaurant located in a well-lit and safe area, especially during evening hours. The presence of nearby attractions or landmarks can also positively influence the user’s perception of the restaurant’s location.
Data Sources for Restaurant Recommendations
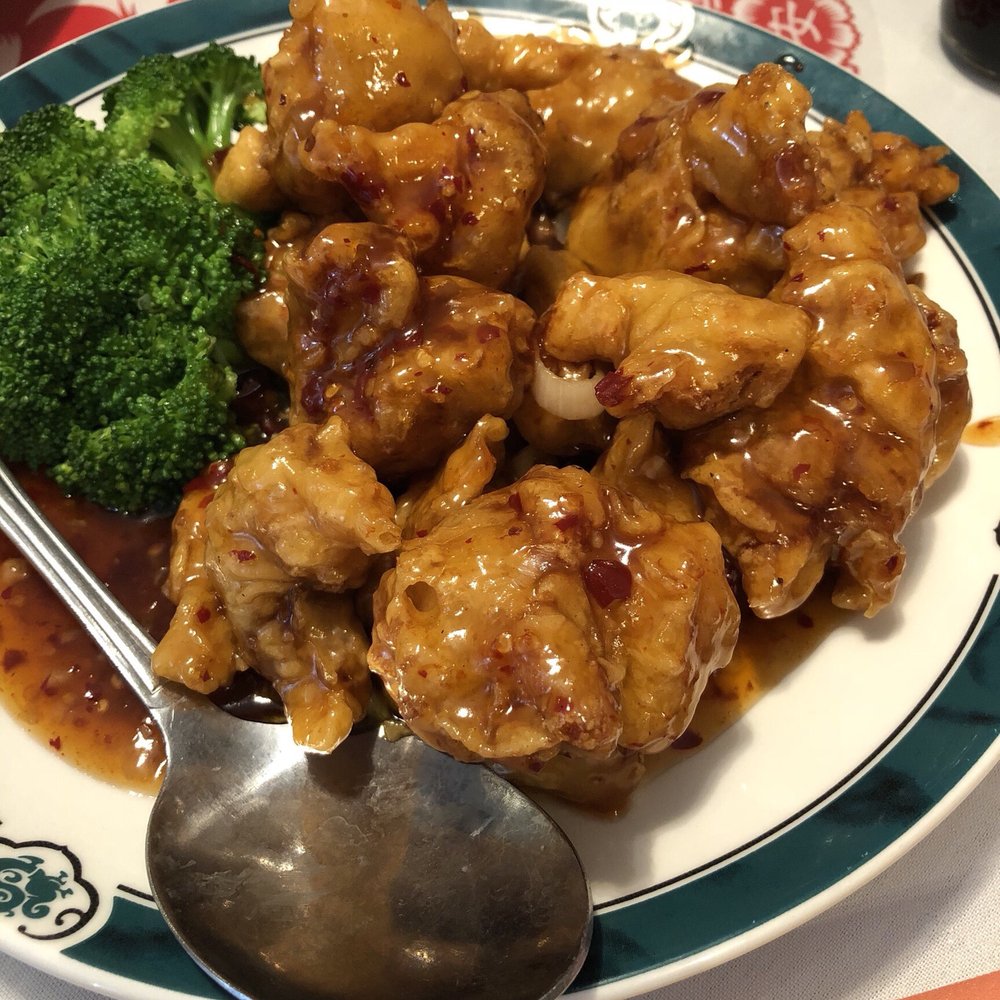
Finding the perfect restaurant can feel like searching for a needle in a haystack. But thankfully, we live in an age of abundant data, allowing us to leverage powerful tools and platforms to make informed decisions. Understanding the strengths and weaknesses of different data sources is crucial for getting accurate and unbiased recommendations. This section will dissect the major players in the restaurant recommendation game, analyzing their contributions and limitations.
The accuracy and completeness of restaurant recommendations heavily depend on the quality and quantity of data used. Different platforms employ varying methodologies and data sources, leading to diverse results. This analysis will explore the key data sources, focusing on their advantages, disadvantages, and potential biases.
Yelp’s Role in Restaurant Recommendations
Yelp has become synonymous with user-generated restaurant reviews. Its vast database, built on millions of user contributions, provides a rich source of information, including ratings, reviews, photos, and even wait times. Yelp’s algorithm prioritizes user engagement, pushing highly-rated and actively reviewed establishments to the top.
Advantages of Yelp include its comprehensive coverage, detailed user reviews, and the ability to filter results based on various criteria (e.g., price range, cuisine, ambiance). However, Yelp’s reliance on user-generated content introduces potential biases. Reviews can be subjective, influenced by personal experiences and even manipulated by businesses or competitors. Furthermore, the algorithm’s focus on engagement can inadvertently amplify popular, trendy restaurants, potentially overshadowing hidden gems with fewer reviews.
Google Maps’ Influence on Restaurant Discovery
Google Maps boasts a massive user base and integrates seamlessly with other Google services. Its restaurant information is often comprehensive, including menus, hours, contact information, and user ratings. Google’s algorithm, known for its sophisticated machine learning capabilities, analyzes various data points, including user reviews, location data, and business listings, to provide personalized recommendations.
Google Maps excels in its accuracy of location data and its integration with other services, making it easy to plan a route or check restaurant availability. However, the sheer volume of data can sometimes lead to less detailed reviews compared to Yelp. Additionally, Google’s algorithm, while powerful, can be opaque, making it difficult to understand the factors influencing recommendations.
TripAdvisor’s Impact on Restaurant Choices
TripAdvisor, primarily focused on travel, also offers extensive restaurant information. Its database encompasses a global reach, providing reviews and ratings for establishments across various countries and regions. TripAdvisor uses a points-based system to rank restaurants, taking into account both the quantity and quality of reviews.
TripAdvisor’s global reach is a significant advantage, especially for travelers. Its points-based ranking system provides a relatively objective measure of restaurant quality. However, similar to Yelp, TripAdvisor is susceptible to biases stemming from user-generated content. Additionally, the focus on tourist-oriented establishments might lead to an underrepresentation of local favorites.
The Significance of User Reviews and Ratings
User reviews and ratings form the backbone of most restaurant recommendation systems. They provide invaluable insights into the dining experience, encompassing aspects like food quality, service, ambiance, and value. The sheer volume of user feedback allows for a statistical analysis, identifying trends and patterns that help predict customer satisfaction.
However, the interpretation of user reviews requires caution. Individual experiences are subjective, and reviews can be influenced by factors unrelated to the restaurant’s inherent quality (e.g., a bad day, a specific server’s performance). Therefore, relying solely on reviews can be misleading. A robust recommendation system should consider a variety of data points, combining user feedback with objective metrics and contextual information.
Factors Influencing Restaurant Recommendations
Restaurant recommendation systems, the digital gatekeepers to our next culinary adventure, are far more sophisticated than simple star ratings. They leverage a complex interplay of factors to deliver personalized results, optimizing for relevance and user satisfaction. Understanding these factors is crucial for both businesses aiming to improve their online presence and users seeking the perfect dining experience. This analysis delves into the key elements shaping restaurant rankings and the algorithms behind them.
The weighting of different factors in a recommendation system is not static; it’s dynamic and adapts based on individual user preferences and the overall data landscape. Think of it as a constantly recalibrating scale, with some factors holding more weight than others depending on the context. For instance, a user who frequently searches for “cheap eats” will see price range as a significantly heavier factor than ambiance, compared to a user who consistently chooses high-end restaurants.
Key Factors in Restaurant Ranking
Several key elements contribute to a restaurant’s prominence in recommendation systems. These range from objective metrics like ratings and reviews to subjective aspects such as cuisine type and ambiance. The relative importance of each factor is constantly adjusted by the algorithm based on user behavior and data analysis.
- Ratings and Reviews: Average star ratings and the volume of reviews are foundational. A high average rating with numerous reviews signals strong overall satisfaction. The recency of reviews is also considered; newer reviews hold more weight as they reflect the current state of the restaurant.
- Cuisine Type and Dietary Restrictions: User preferences for specific cuisines (e.g., Italian, Mexican, Vegan) are heavily considered. The system matches restaurants offering the desired cuisine types and caters to dietary needs (vegetarian, gluten-free, etc.) indicated in the user profile.
- Price Range: Budget is a critical filter. Recommendations are tailored to the user’s specified or inferred price range, ensuring suggestions align with their spending capacity. A user searching for “budget-friendly restaurants” will see drastically different results than one looking for “fine dining experiences”.
- Ambiance and Atmosphere: The desired dining atmosphere (romantic, casual, family-friendly) is factored in. User reviews and descriptions often provide insights into a restaurant’s ambiance, allowing the system to match preferences accurately. A user looking for a quiet date night will be shown different results than someone searching for a lively spot for a group celebration.
- Location and Distance: Proximity is paramount. Recommendations prioritize restaurants within a reasonable distance from the user’s specified location or their automatically detected location. This ensures practical accessibility for the user.
- Menu Items and Specific Dishes: Advanced systems analyze menu data to understand the specific dishes offered. This enables more precise matching based on user preferences for particular cuisines or dishes, going beyond simple cuisine categorization.
A Hypothetical Recommendation Algorithm, Recommended restaurants near me
A sophisticated recommendation algorithm would incorporate these factors using a weighted scoring system. Imagine an algorithm that assigns weights to each factor based on user history and current search parameters.
The overall score for a restaurant (Rscore) could be calculated as:
Rscore = w1 * Rrating + w2 * Rreviews + w3 * Ccuisine + w4 * Pprice + w5 * Aambiance + w6 * Ddistance + w7 * Mmenu
Where:
* Rrating = Average rating
* Rreviews = Number of reviews
* Ccuisine = Cuisine type match
* Pprice = Price range match
* Aambiance = Ambiance match
* Ddistance = Distance from user
* Mmenu = Menu item match
* w1…w7 = weights assigned to each factor based on user preferences and context.
This formula provides a flexible framework. The weights (w1 to w7) are dynamically adjusted based on user behavior, ensuring that the system continuously learns and refines its recommendations. For example, a user who frequently ignores price range suggestions will see the weight of Pprice reduced over time.
Presenting Restaurant Recommendations: Recommended Restaurants Near Me
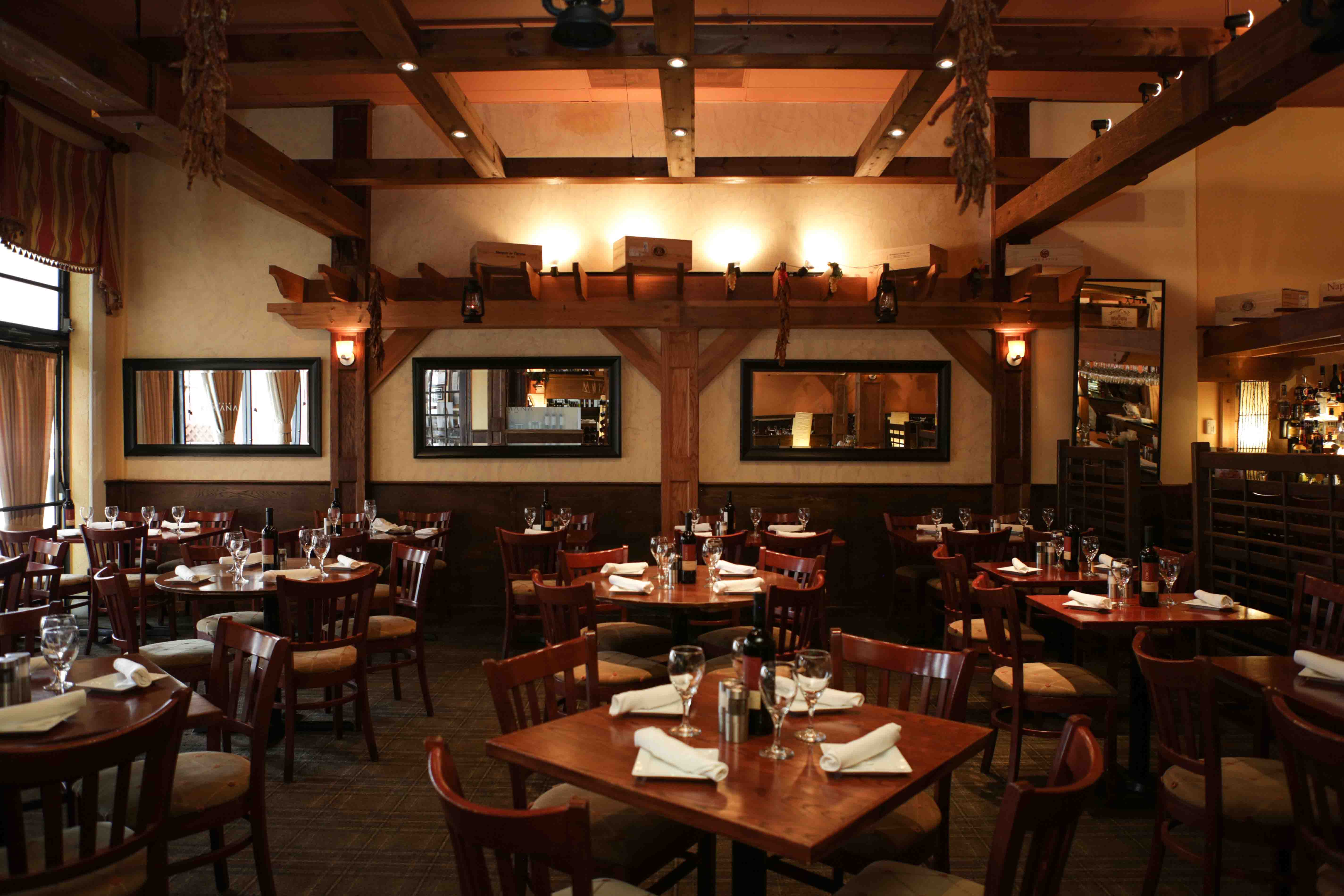
Getting the right restaurant recommendations in front of your users is crucial for success. A poorly presented list can lead to frustration and lost opportunities, while a well-designed presentation can significantly boost engagement and conversions. Remember, the goal is to make finding the perfect meal as effortless and enjoyable as possible. The key is clarity, visual appeal, and easy access to essential information.
Presenting restaurant recommendations effectively requires a strategic approach. You need to consider not only the data you’re displaying but also the user experience. Think about how people naturally search for and choose restaurants. Are they looking for something quick and cheap, or a luxurious dining experience? Tailoring your presentation to these diverse needs is vital.
Restaurant Recommendation Table
A well-structured table is a classic and effective way to present restaurant information concisely. Using responsive design ensures readability across various devices.
Restaurant Name | Address | Cuisine | Rating | Online Ordering |
---|---|---|---|---|
The Italian Place | 123 Main Street, Anytown | Italian | 4.5 stars | Order Now |
Spicy Tacos | 456 Oak Avenue, Anytown | Mexican | 4 stars | Order Now |
Sushi Sensation | 789 Pine Lane, Anytown | Japanese | 4.8 stars | Order Now |
Burger Bliss | 101 Elm Street, Anytown | American | 4.2 stars | Order Now |
Alternative Presentation Methods
Tables aren’t the only option. Diversifying your presentation methods caters to different user preferences and improves overall engagement.
Bullet Points: A simple, straightforward approach, ideal for quick overviews. For example: *The Italian Place (Italian, 4.5 stars),* *Spicy Tacos (Mexican, 4 stars),* etc. This works well for concise lists but lacks visual appeal for larger datasets.
Image Cards: Visually rich and engaging. Each card could feature a restaurant’s logo or a mouth-watering food image, along with its name, cuisine, and rating. Imagine a card showing a sizzling steak with “Steakhouse 57 (American, 4.7 stars)” overlaid. This approach is highly effective for grabbing attention but requires more design effort.
Map Integration: Allows users to visually locate restaurants and see their proximity to their current location. This is particularly useful for users who prioritize location. A map with restaurant markers, each linked to its details page, is highly effective for location-based search.
Effective and Ineffective Restaurant Information Display
The effectiveness of your presentation hinges on clarity and user-friendliness. Consider these examples:
Effective: Clear, concise language; prominent display of key information (name, cuisine, rating); easy-to-use filters and sorting options; high-quality images; clear calls to action (e.g., “Order Now,” “View Menu”). For instance, a clean interface with large fonts, clear imagery, and a simple star rating system.
Ineffective: Cluttered layout; too much text; small, hard-to-read fonts; low-quality images; ambiguous or misleading information; lack of clear calls to action. Imagine a page crammed with tiny text, blurry pictures, and unclear ratings – it’s overwhelming and frustrating for the user.
Handling User Preferences and Context
Personalizing the restaurant recommendation experience is paramount to driving user engagement and satisfaction. Ignoring user preferences leads to irrelevant suggestions and ultimately, a frustrating user experience. By effectively incorporating user data and context, we can transform a generic search into a highly targeted and valuable service. This involves understanding not just *what* the user wants, but *who* the user is and what their needs are at that specific moment.
Understanding and leveraging user location, dietary restrictions, price sensitivity, and preferred cuisines are crucial steps in delivering relevant restaurant recommendations. Sophisticated algorithms analyze this data in real-time to provide personalized results. This goes beyond simply filtering results; it’s about predicting what a user will find most appealing based on their unique profile. Imagine a vegetarian user searching for restaurants near their current location – a system without context might return a list of steakhouses. A well-designed system, however, will prioritize vegetarian options in the immediate vicinity.
User Location and Preferences
User location is the cornerstone of any location-based service. GPS data, IP address, or manually entered addresses provide the geographical context. This allows the system to filter restaurants based on proximity, travel time, or even specific neighborhoods. Preferences, such as cuisine type (e.g., Italian, Mexican, Thai), price range (e.g., $, $$, $$$), and dietary restrictions (e.g., vegetarian, vegan, gluten-free) are crucial filters that refine the recommendations. A database of restaurants with detailed attributes, including menus, price points, and user reviews, is essential for effective filtering. For example, a user specifying “vegan Thai food under $20 near me” will receive a highly tailored list of matching establishments. The system should also consider factors like opening hours and current availability to provide the most accurate and up-to-date recommendations.
Handling Ambiguous or Incomplete Queries
Users don’t always provide perfectly clear requests. Ambiguous queries, such as “restaurants nearby,” require the system to make intelligent assumptions. This often involves utilizing contextual information. For instance, “restaurants nearby” might default to a radius of 5 kilometers around the user’s location, or it might present a range of options with varying distances, clearly labeled. Incomplete queries, like specifying only cuisine type without a location, might trigger a prompt asking for further clarification or default to the user’s last known location. Natural Language Processing (NLP) techniques can help interpret vague language and infer user intent, making the recommendation process more robust and user-friendly. For example, a query like “best pizza” might be interpreted as “best-rated pizza restaurants near me.”
Personalized Recommendations Based on Past User Behavior
Past user behavior is a goldmine for personalization. Tracking past searches, restaurant visits, ratings, and reviews allows the system to build a detailed user profile. This profile informs future recommendations, suggesting restaurants similar to those the user has liked in the past. Collaborative filtering techniques, which analyze the preferences of similar users, can further enhance personalization. For example, if a user consistently rates Italian restaurants highly, the system might prioritize Italian options in future searches, even if the user doesn’t explicitly specify a cuisine type. This approach leverages the wisdom of the crowd to provide more accurate and insightful recommendations. Machine learning models can be trained on this data to predict user preferences with increasing accuracy over time.
Visual Representation of Restaurant Information
Visuals are paramount in driving user engagement and effectively conveying crucial restaurant information. A picture, as they say, is worth a thousand words, and in the context of restaurant recommendations, compelling visuals can significantly impact a user’s decision-making process. We need to leverage this power to present information clearly and attractively.
Effective visual representation of restaurant information goes beyond simply displaying a logo. It’s about creating a holistic experience that captures the essence of the dining establishment and caters to the user’s desire for a seamless and informative search.
Restaurant Scene Depiction
Imagine a photograph showcasing a bustling yet intimate restaurant scene. The image is warm and inviting, bathed in the soft glow of strategically placed lighting. The foreground features a beautifully presented dish – perhaps a vibrant pasta primavera or a succulent steak – garnished meticulously and styled for maximum visual appeal. In the background, you see happy diners engaged in conversation, laughter echoing subtly in the ambiance. The restaurant’s décor is visible – perhaps exposed brick walls complemented by modern artwork, or sleek, minimalist furniture creating a sophisticated atmosphere. The overall effect is one of warmth, sophistication, and delicious food, carefully composed to draw the user in. The image subtly highlights key features: the quality of the food presentation, the inviting ambiance, and the positive customer experience, all without being overly staged or artificial. The color palette is rich and vibrant, capturing the essence of a delightful dining experience. This isn’t just a picture of a restaurant; it’s a carefully curated visual narrative.
Infographic Illustrating Restaurant Type Distribution
A compelling infographic would present the distribution of restaurant types within a specific geographical area. Imagine a circular map representing the area, divided into color-coded segments representing different cuisines. The size of each segment corresponds directly to the number of restaurants of that specific type. For example, a large segment of vibrant red might represent the high concentration of Italian restaurants in the area, while a smaller, teal segment might signify a smaller but still significant number of Thai restaurants. A legend clearly defines each color and the corresponding cuisine. This visual representation instantly provides users with a clear understanding of the culinary landscape in their vicinity. The infographic could further incorporate data visualizations, such as bar charts, to show the popularity trends of different cuisines over time, providing even more context and insightful information for users. For instance, one might show a comparison of the growth of vegan restaurants versus traditional burger joints over the past five years. This type of data-driven visual representation offers valuable insights that enhance the user experience.